Graph Networks with Physics-aware Knowledge Informed in Latent Space.
AAAI Spring Symposium - MLPS(2021)
摘要
While physics conveys knowledge of nature built from an interplay between observations and theory, it has been considered less important for modeling deep neural networks. Despite the usefulness of physical rules, it is particularly challenging to leverage the knowledge for sparse data since most physics equations are well defined on the continuous and dense space. In addition, it is even harder to inform the equations into a model if the observations are not fully governed by the given physical knowledge. In this work, we present a novel architecture to incorporate physics or domain knowledge given as a form of partial differential equations (PDEs) on sparse observations by utilizing graph structure. Moreover, we leverage the representation power of deep learning by informing the knowledge in latent space. We demonstrate that climate prediction tasks are significantly improved and validate the effectiveness and importance of the proposed model.
更多查看译文
关键词
Knowledge Graph Embedding,Signal Processing on Graphs,Graph Neural Networks,Graph Convolutional Networks,Heterogeneous Networks
AI 理解论文
溯源树
样例
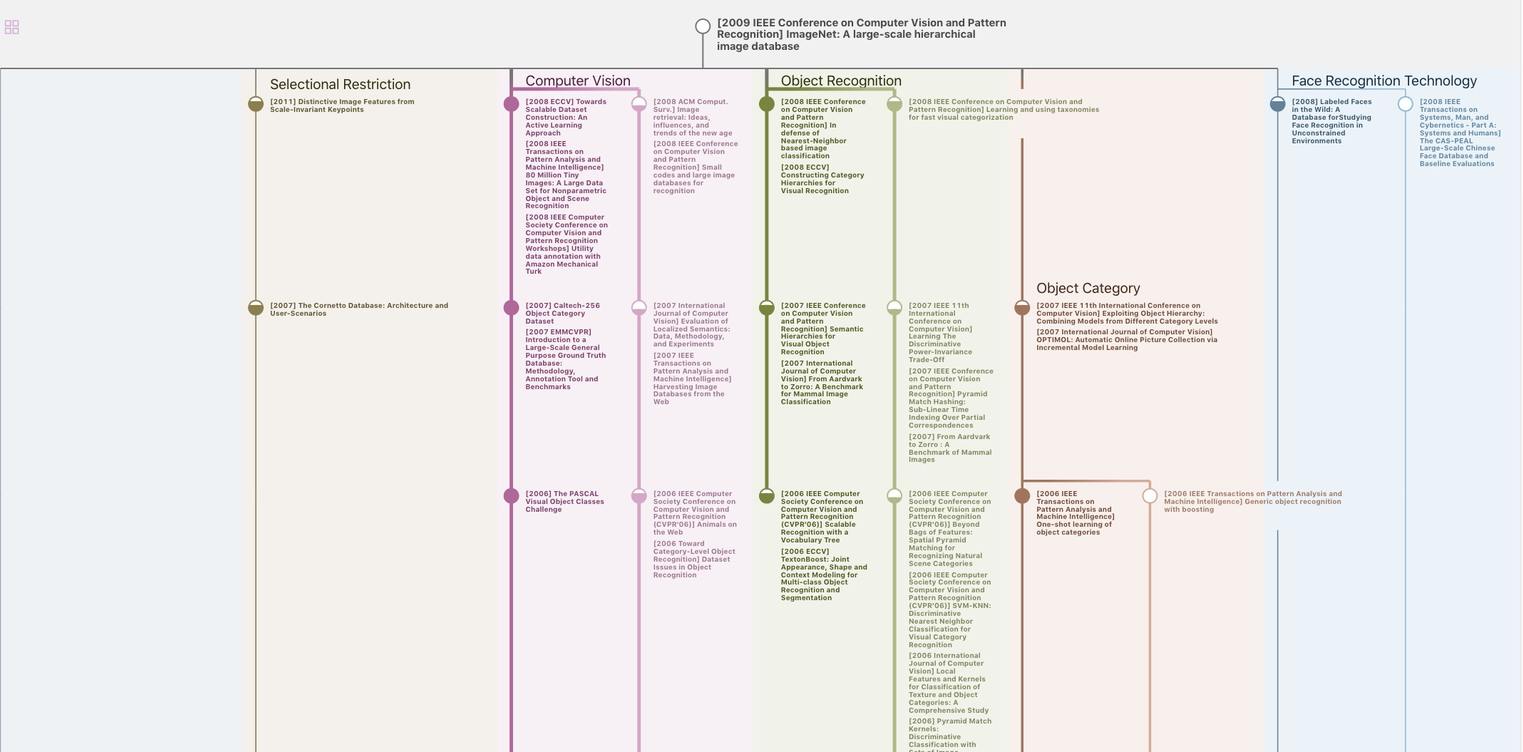
生成溯源树,研究论文发展脉络
Chat Paper
正在生成论文摘要