Machine learner optimization of optical nanofiber-based dipole traps for cold $^{87}$Rb atoms
arxiv(2021)
摘要
In two-color optical nanofiber-based dipole traps for cold alkali atoms, the trap efficiency depends on the wavelength and intensity of light in the evanescent field, and the initial laser-cooling process. Typically, no more than one atom can be trapped per trapping site. Improving the trapping efficiency can increase the number of filled trapping sites, thereby increasing the optical depth. Here, we report on the implementation of an in-loop stochastic artificial neural network machine learner to trap $^{87}$Rb atoms in an uncompensated two-color evanescent field dipole trap by optimizing the absorption of a near-resonant, nanofiber-guided, probe beam. By giving the neural network control of the laser cooling process, we observe an increase in the number of dipole-trapped atoms by $\sim$ 50%, a small decrease in their average temperature from 150 $\mu$K to 140 $\mu$K, and an increase in peak optical depth by 70%. The machine learner is able to quickly and effectively explore the large parameter space of the laser cooling control to find optimal parameters for loading the dipole traps. The increased number of atoms should facilitate studies of collective atom-light interactions mediated via the evanescent field.
更多查看译文
关键词
dipole traps,nanofiber-based
AI 理解论文
溯源树
样例
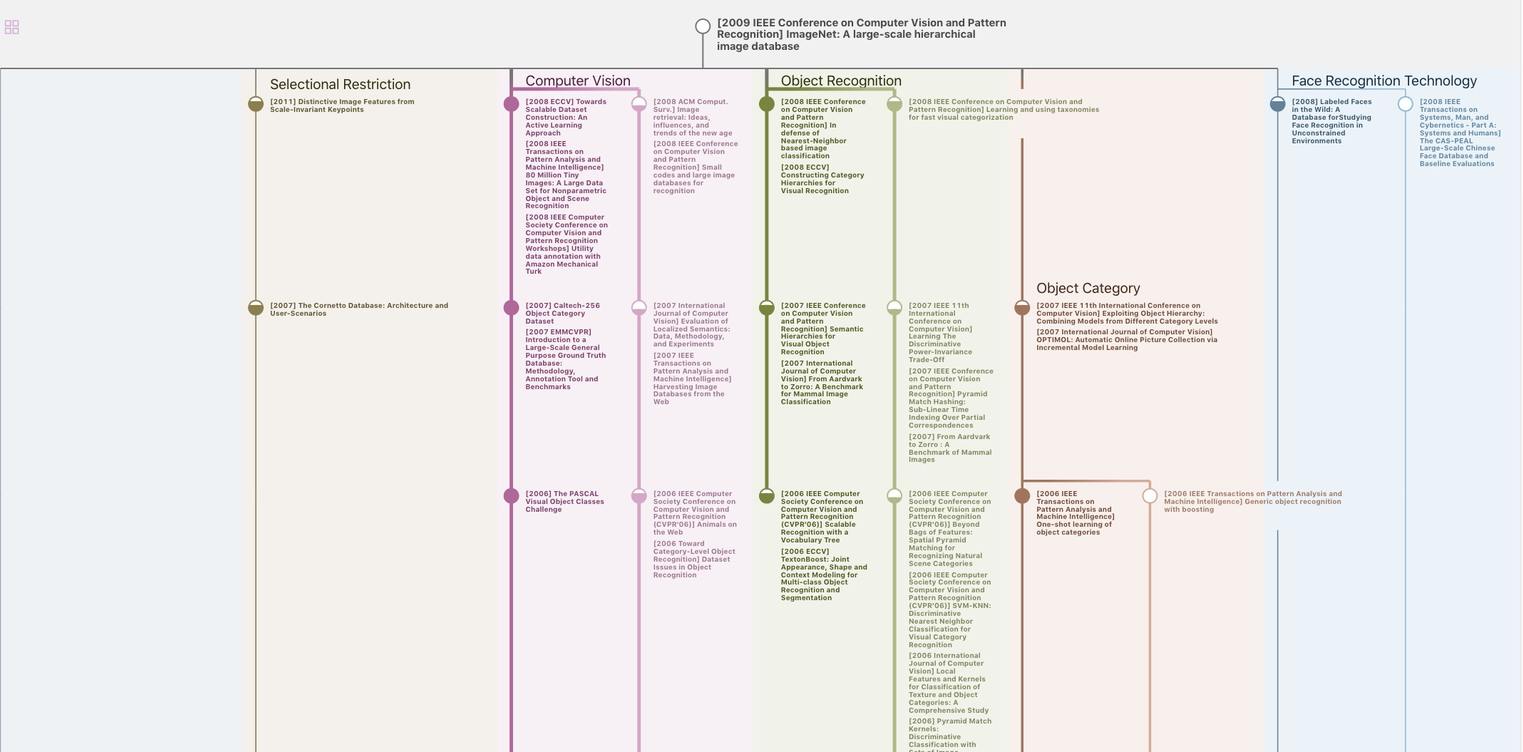
生成溯源树,研究论文发展脉络
Chat Paper
正在生成论文摘要