Improving Kinodynamic Planners for Vehicular Navigation with Learned Goal-Reaching Controllers
2021 IEEE/RSJ INTERNATIONAL CONFERENCE ON INTELLIGENT ROBOTS AND SYSTEMS (IROS)(2021)
摘要
This paper aims to improve the path quality and computational efficiency of sampling-based kinodynamic planners for vehicular navigation. It proposes a learning framework for identifying promising controls during the expansion process of sampling-based planners. Given a dynamics model, a reinforcement learning process is trained offline to return a low-cost control that reaches a local goal state (i.e., a waypoint) in the absence of obstacles. By focusing on the system's dynamics and not knowing the environment, this process is data-efficient and takes place once for a robotic system. In this way, it can be reused in different environments. The planner generates online local goal states for the learned controller in an informed manner to bias towards the goal and consecutively in an exploratory, random manner. For the informed expansion, local goal states are generated either via (a) medial axis information in environments with obstacles, or (b) wavefront information for setups with traversability costs. The learning process and the resulting planning framework are evaluated for a first and second-order differential drive system, as well as a physically simulated Segway robot. The results show that the proposed integration of learning and planning can produce higher quality paths than sampling-based kinodynamic planning with random controls in fewer iterations and computation time.
更多查看译文
关键词
vehicular navigation,learned goal-reaching controllers,path quality,computational efficiency,sampling-based kinodynamic planners,learning framework,promising controls,expansion process,sampling-based planners,dynamics model,reinforcement learning process,low-cost control,local goal state,data-efficient,robotic system,online local goal states,learned controller,informed manner,random manner,informed expansion,medial axis information,wavefront information,traversability costs,resulting planning framework,first order differential drive system,second-order differential drive system,higher quality paths,sampling-based kinodynamic planning,random controls
AI 理解论文
溯源树
样例
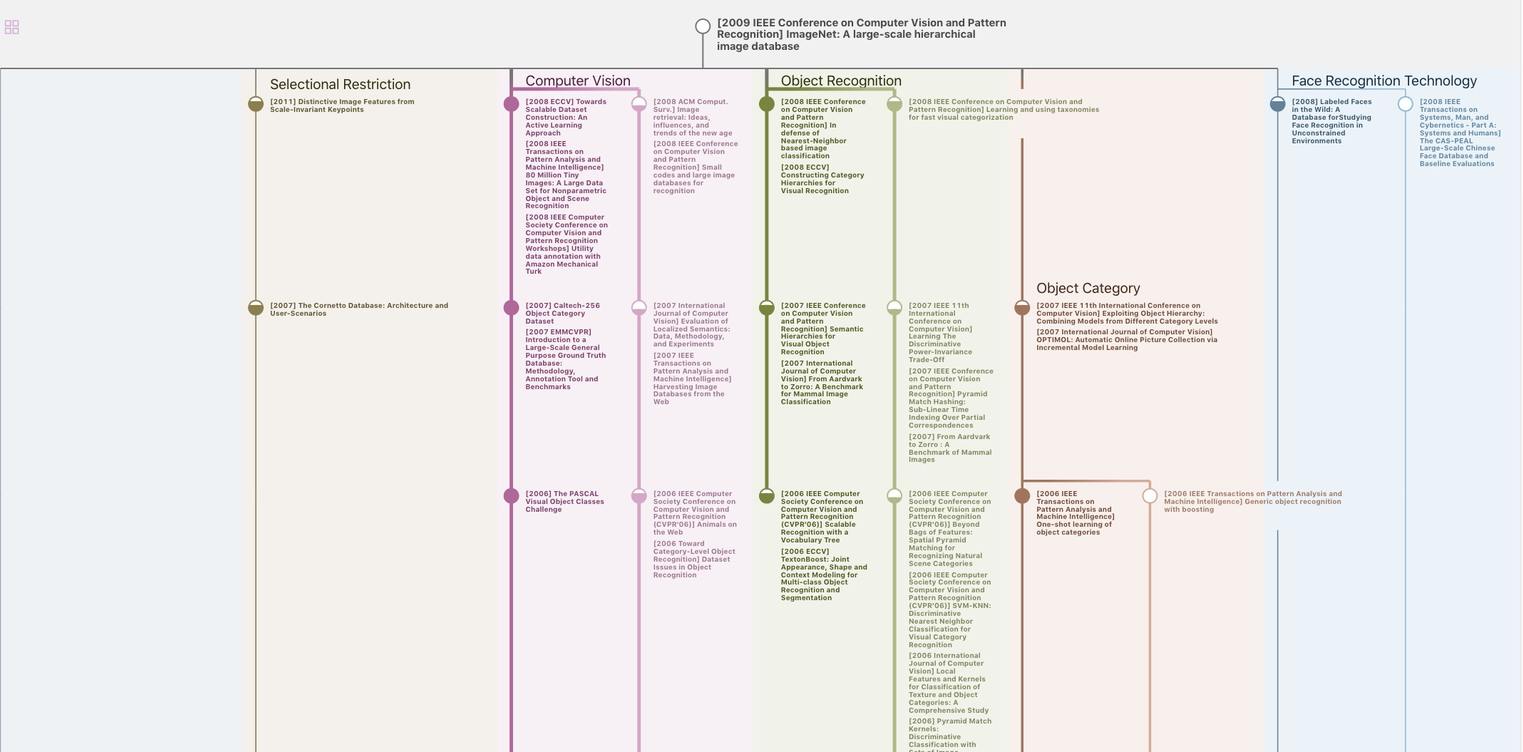
生成溯源树,研究论文发展脉络
Chat Paper
正在生成论文摘要