Self-explaining Neural Network with Plausible Explanations
arxiv(2021)
摘要
Explaining the predictions of complex deep learning models, often referred to as black boxes, is critical in high-stakes domains like healthcare. However, post-hoc model explanations often are not understandable by clinicians and are difficult to integrate into clinical workflow. Further, while most explainable models use individual clinical variables as units of explanation, human understanding often rely on higher-level concepts or feature representations. In this paper, we propose a novel, self-explaining neural network for longitudinal in-hospital mortality prediction using domain-knowledge driven Sequential Organ Failure Assessment (SOFA) organ-specific scores as the atomic units of explanation. We also design a novel procedure to quantitatively validate the model explanations against gold standard discharge diagnosis information of patients. Our results provide interesting insights into how each of the SOFA organ scores contribute to mortality at different timesteps within longitudinal patient trajectory.
更多查看译文
关键词
plausible explanations,neural network,self-explaining
AI 理解论文
溯源树
样例
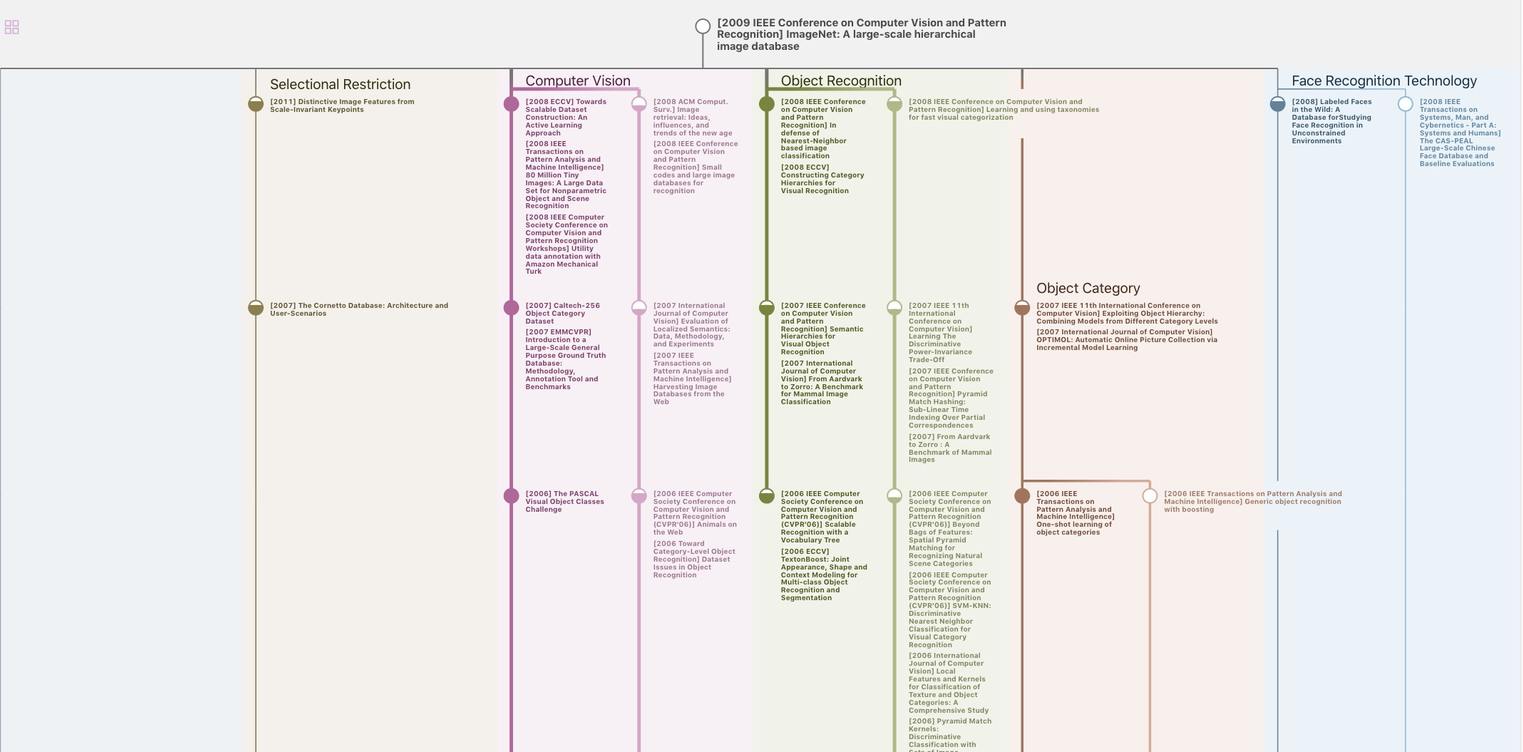
生成溯源树,研究论文发展脉络
Chat Paper
正在生成论文摘要