Self-appearance-aided Differential Evolution for Motion Transfer
arxiv(2021)
摘要
Image animation transfers the motion of a driving video to a static object in a source image, while keeping the source identity unchanged. Great progress has been made in unsupervised motion transfer recently, where no labelled data or ground truth domain priors are needed. However, current unsupervised approaches still struggle when there are large motion or viewpoint discrepancies between the source and driving images. In this paper, we introduce three measures that we found to be effective for overcoming such large viewpoint changes. Firstly, to achieve more fine-grained motion deformation fields, we propose to apply Neural-ODEs for parametrizing the evolution dynamics of the motion transfer from source to driving. Secondly, to handle occlusions caused by large viewpoint and motion changes, we take advantage of the appearance flow obtained from the source image itself ("self-appearance"), which essentially "borrows" similar structures from other regions of an image to inpaint missing regions. Finally, our framework is also able to leverage the information from additional reference views which help to drive the source identity in spite of varying motion state. Extensive experiments demonstrate that our approach outperforms the state-of-the-arts by a significant margin (~40%), across six benchmarks varying from human faces, human bodies to robots and cartoon characters. Model generality analysis indicates that our approach generalises the best across different object categories as well.
更多查看译文
关键词
motion transfer,differential evolution,self-appearance-aided
AI 理解论文
溯源树
样例
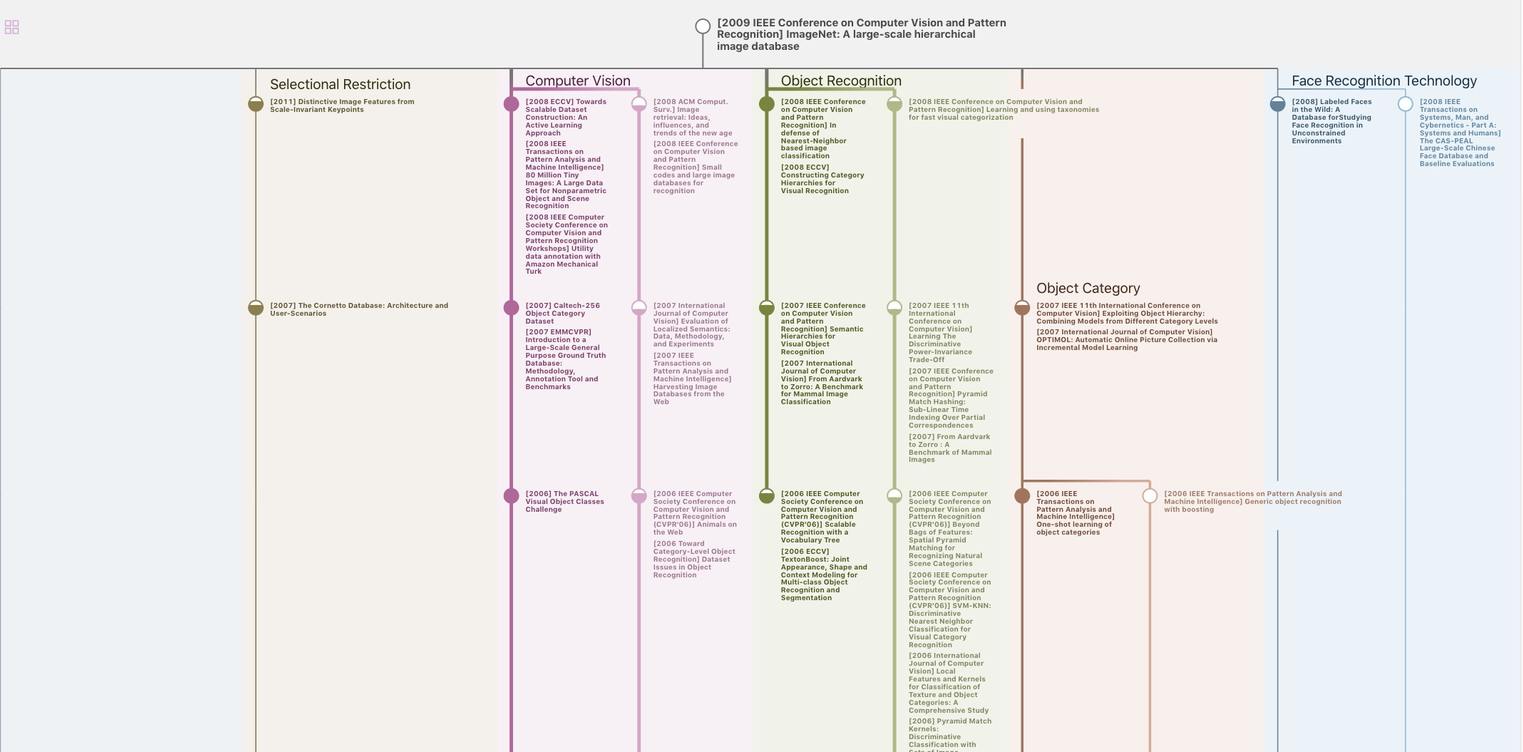
生成溯源树,研究论文发展脉络
Chat Paper
正在生成论文摘要