Reinforcement Learning for Systematic FX Trading
IEEE ACCESS(2022)
摘要
We explore online inductive transfer learning, with a feature representation transfer from a radial basis function network formed of Gaussian mixture model hidden processing units to a direct, recurrent reinforcement learning agent. This agent is put to work in an experiment, trading the major spot market currency pairs, where we accurately account for transaction and funding costs. These sources of profit and loss, including the price trends that occur in the currency markets, are made available to the agent via a quadratic utility, who learns to target a position directly. We improve upon earlier work by targeting a risk position in an online transfer learning context. Our agent achieves an annualised portfolio information ratio of 0.52 with a compound return of 9.3%, net of execution and funding cost, over a 7-year test set; this is despite forcing the model to trade at the close of the trading day at 5 pm EST when trading costs are statistically the most expensive.
更多查看译文
关键词
Transfer learning, Reinforcement learning, Costs, Time series analysis, Currencies, Task analysis, Portfolios, Policy gradients, recurrent reinforcement learning, online learning, transfer learning, financial time series
AI 理解论文
溯源树
样例
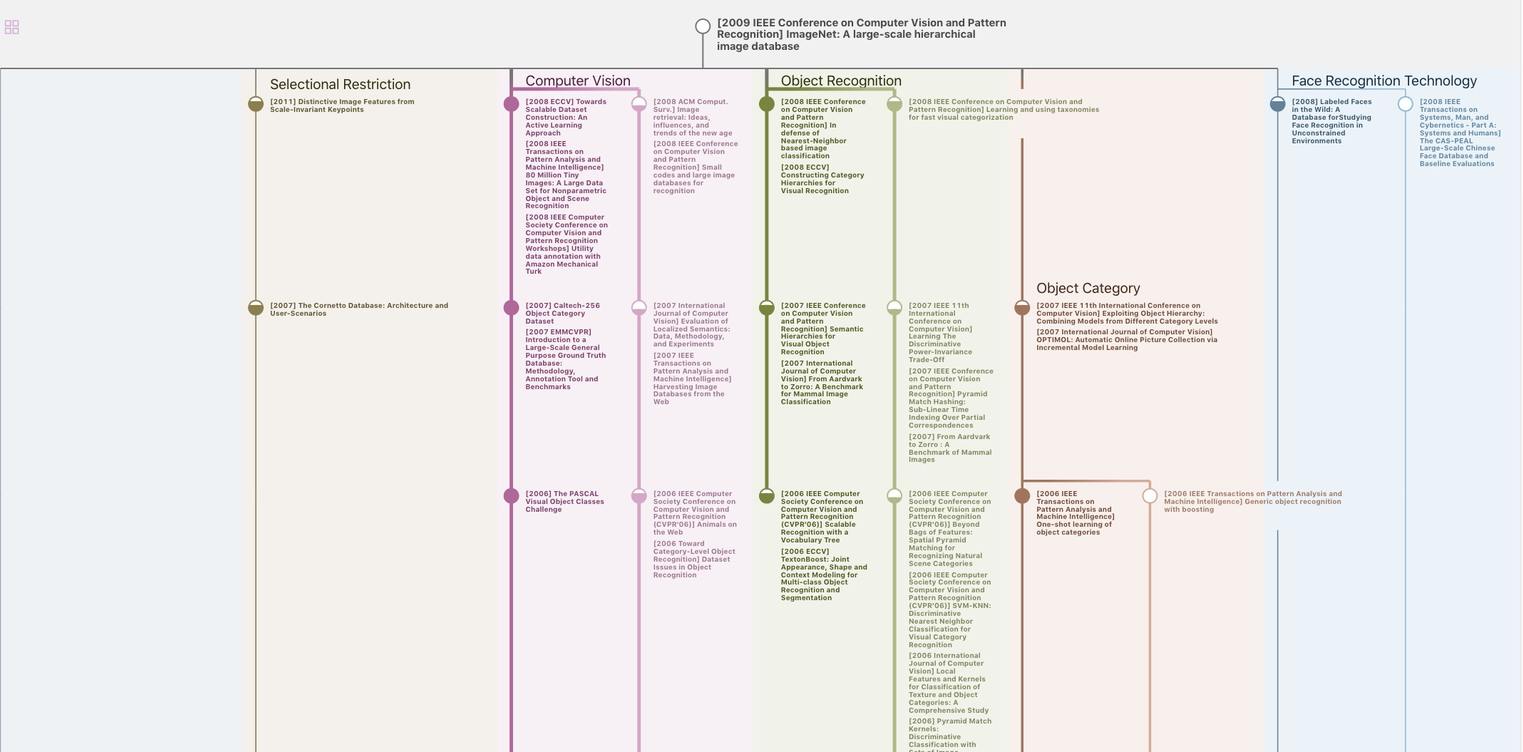
生成溯源树,研究论文发展脉络
Chat Paper
正在生成论文摘要