CoRGi: Content-Rich Graph Neural Networks with Attention
KDD '22: Proceedings of the 28th ACM SIGKDD Conference on Knowledge Discovery and Data Mining(2022)
摘要
Graph representations of a target domain often project it to a set of entities (nodes) and their relations (edges). However, such projections often miss important and rich information. For example, in graph representations used in missing value imputation, items --- represented as nodes --- may contain rich textual information. However, when processing graphs with graph neural networks (GNN), such information is either ignored or summarized into a single vector representation used to initialize the GNN. Towards addressing this, we present CoRGi, a GNN that considers the rich data within nodes in the context of their neighbors. This is achieved by endowing CoRGi's message passing with a personalized attention mechanism over the content of each node. This way, CoRGi assigns user-item-specific attention scores with respect to the words that appear in an item's content. We evaluate CoRGi on two edge-value prediction tasks and show that CoRGi is better at making edge-value predictions over existing methods, especially on sparse regions of the graph.
更多查看译文
关键词
attention,networks,content-rich
AI 理解论文
溯源树
样例
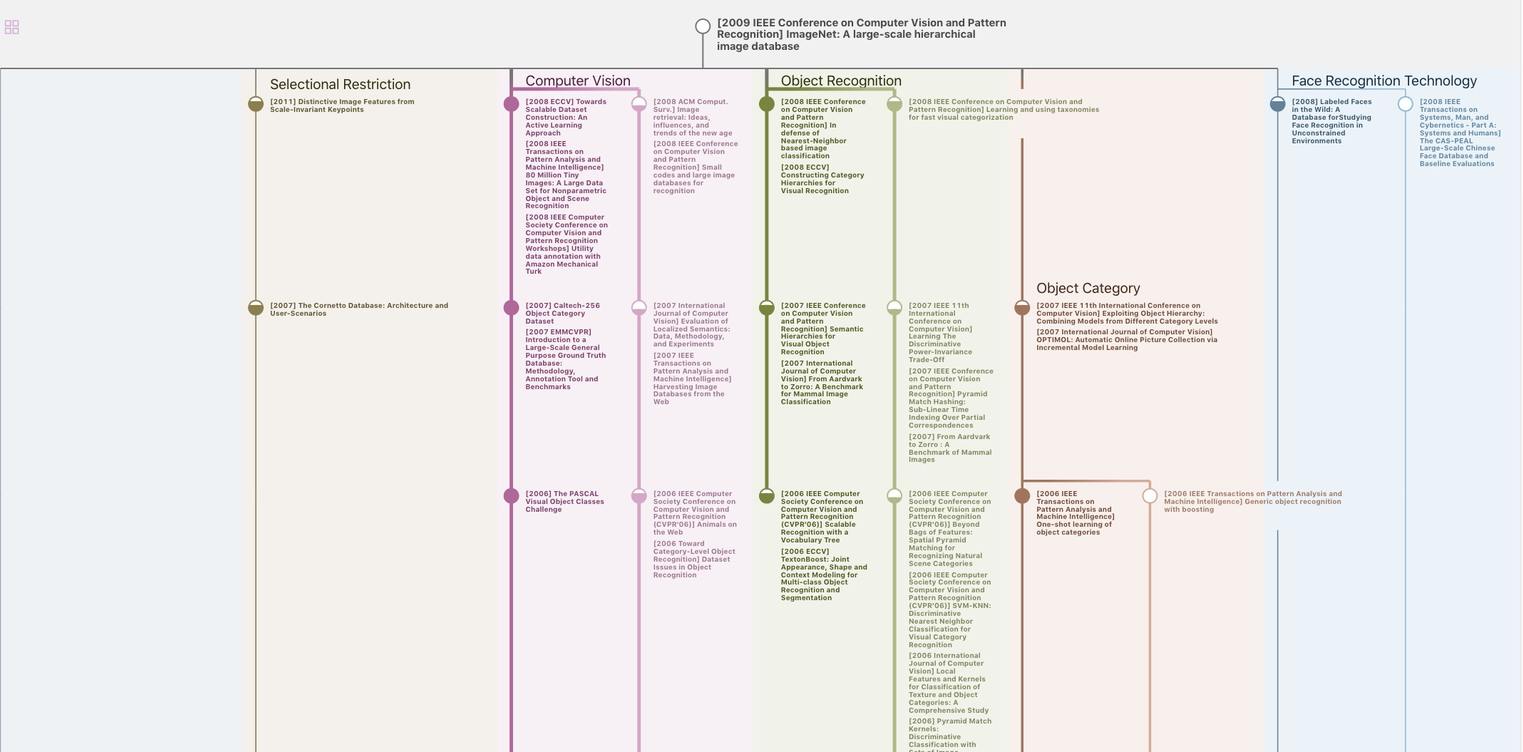
生成溯源树,研究论文发展脉络
Chat Paper
正在生成论文摘要