Multi-View Self-Attention Based Transformer for Speaker Recognition.
IEEE International Conference on Acoustics, Speech, and Signal Processing (ICASSP)(2022)
摘要
Initially developed for natural language processing (NLP), Transformer model is now widely used for speech processing tasks such as speaker recognition, due to its powerful sequence modeling capabilities. However, conventional self-attention mechanisms are originally designed for modeling textual sequence without considering the characteristics of speech and speaker modeling. Besides, different Transformer variants for speaker recognition have not been well studied. In this work, we propose a novel multi-view self-attention mechanism and present an empirical study of different Transformer variants with or without the proposed attention mechanism for speaker recognition. Specifically, to balance the capabilities of capturing global dependencies and modeling the locality, we propose a multi-view self-attention mechanism for speaker Transformer, in which different attention heads can attend to different ranges of the receptive field. Furthermore, we introduce and compare five Transformer variants with different network architectures, embedding locations, and pooling methods to learn speaker embeddings. Experimental results on the VoxCeleb1 and VoxCeleb2 datasets show that the proposed multi-view self-attention mechanism achieves improvement in the performance of speaker recognition, and the proposed speaker Transformer network attains excellent results compared with state-of-the-art models.
更多查看译文
关键词
speaker recognition,Transformer,speaker identification,speaker verification
AI 理解论文
溯源树
样例
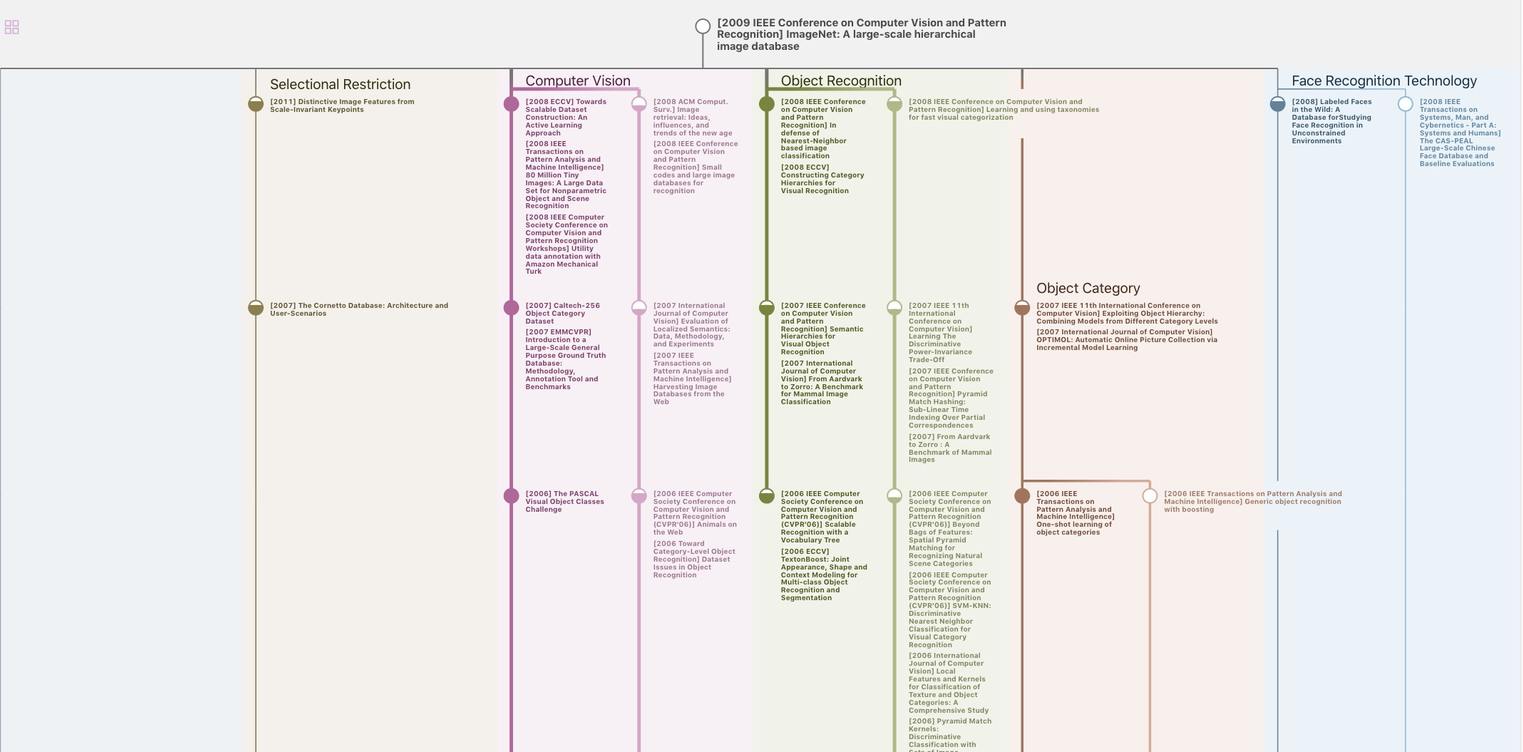
生成溯源树,研究论文发展脉络
Chat Paper
正在生成论文摘要