A Comparative Study on Non-Autoregressive Modelings for Speech-to-Text Generation.
2021 IEEE Automatic Speech Recognition and Understanding Workshop (ASRU)(2021)
摘要
Non-autoregressive (NAR) models simultaneously generate multiple outputs in a sequence, which significantly reduces the inference speed at the cost of accuracy drop compared to autoregressive baselines. Showing great potential for real-time applications, an increasing number of NAR models have been explored in different fields to mitigate the performance gap against AR models. In this work, we conduct a comparative study of various NAR modeling methods for end-to-end automatic speech recognition (ASR). Experiments are performed in the state-of-the-art setting using ESPnet. The results on various tasks provide interesting findings for developing an understanding of NAR ASR, such as the accuracy-speed trade-off and robustness against long-form utterances. We also show that the techniques can be combined for further improvement and applied to NAR end-to-end speech translation. All the implementations are publicly available to encourage further research in NAR speech processing.
更多查看译文
关键词
Non-autoregressive sequence generation,end-to-end speech recognition,end-to-end speech translation
AI 理解论文
溯源树
样例
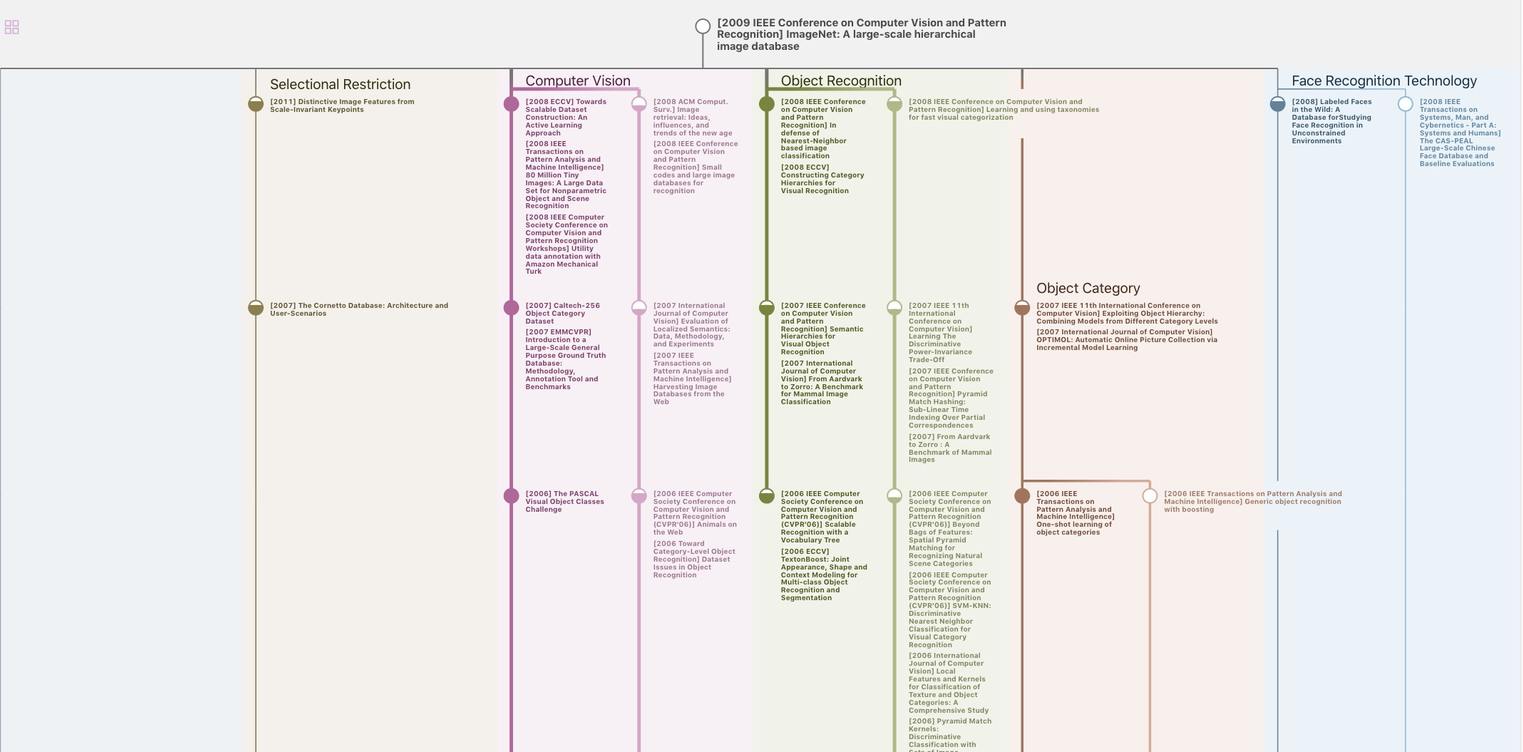
生成溯源树,研究论文发展脉络
Chat Paper
正在生成论文摘要