Anti-cancer Peptide Recognition Based on Grouped Sequence and Spatial Dimension Integrated Networks
Interdisciplinary Sciences: Computational Life Sciences(2021)
摘要
The diversification of the characteristic sequences of anti-cancer peptides has imposed difficulties on research. To effectively predict new anti-cancer peptides, this paper proposes a more suitable feature grouping sequence and spatial dimension-integrated network algorithm for anti-cancer peptide sequence prediction called GRCI-Net. The main process is as follows: First, we implemented the fusion reduction of binary structure features and K-mer sparse matrix features through principal component analysis and generated a set of new features; second, we constructed a new bidirectional long- and short-term memory network. We used traditional convolution and dilated convolution to acquire features in the spatial dimension using the memory network's grouping sequence model, which is designed to better handle the diversification of anti-cancer peptide feature sequences and to fully learn the contextual information between features. Finally, we achieved the fusion of grouping sequence features and spatial dimensional integration features through two sets of dense network layers, achieved the prediction of anti-cancer peptides through the sigmoid function, and verified the approach with two public datasets, ACP740 (accuracy reached 0.8230) and ACP240 (accuracy reached 0.8750). The following is a link to the model code and datasets mentioned in this article: https://github.com/ YouHongfeng101/ACP-DL.
更多查看译文
关键词
Grouping sequence, Long short-term memory, Anti-cancer peptide, Spatial dimension fusion
AI 理解论文
溯源树
样例
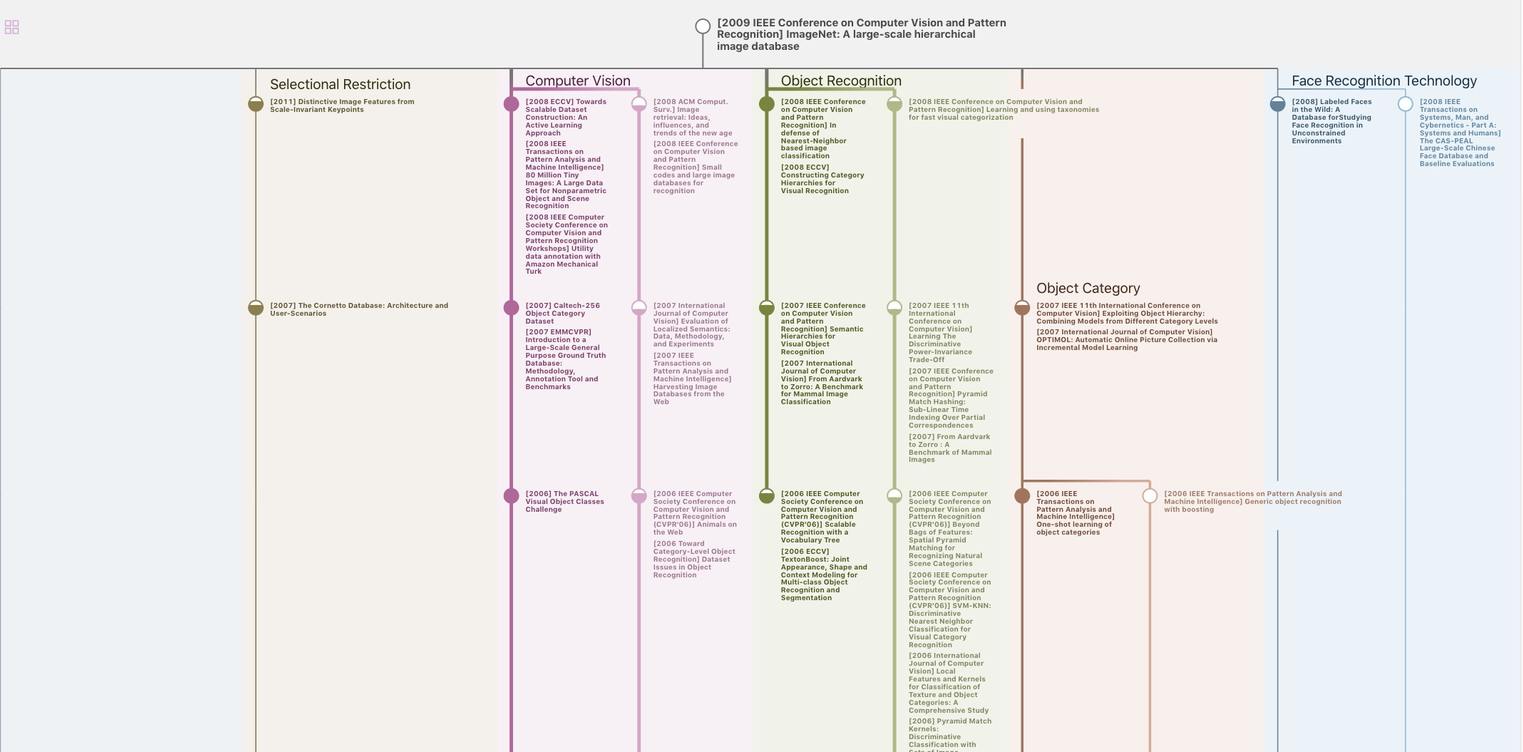
生成溯源树,研究论文发展脉络
Chat Paper
正在生成论文摘要