Incremental k-Nearest Neighbors Using Reservoir Sampling for Data Streams.
DS(2021)
摘要
The online and potentially infinite nature of data streams leads to the inability to store the flow in its entirety and thus restricts the storage to a part of - and/or synopsis information from - the stream. To process these evolving data, we need efficient and accurate methodologies and systems, such as window models (e.g., sliding windows) and summarization techniques (e.g., sampling, sketching, dimensionality reduction). In this paper, we propose, RW-kNN, a k-Nearest Neighbors (kNN) algorithm that employs a practical way to store information about past instances using the biased reservoir sampling to sample the input instances along with a sliding window to maintain the most recent instances from the stream. We evaluate our proposal on a diverse set of synthetic and real datasets and compare against state-of-the-art algorithms in a traditional test-then-train evaluation. Results show how our proposed RW-kNN approach produces high-predictive performance for both real and synthetic datasets while using a feasible amount of resources.
更多查看译文
关键词
Data stream classification,K-nearest neighbors,Reservoir sampling,Sliding window
AI 理解论文
溯源树
样例
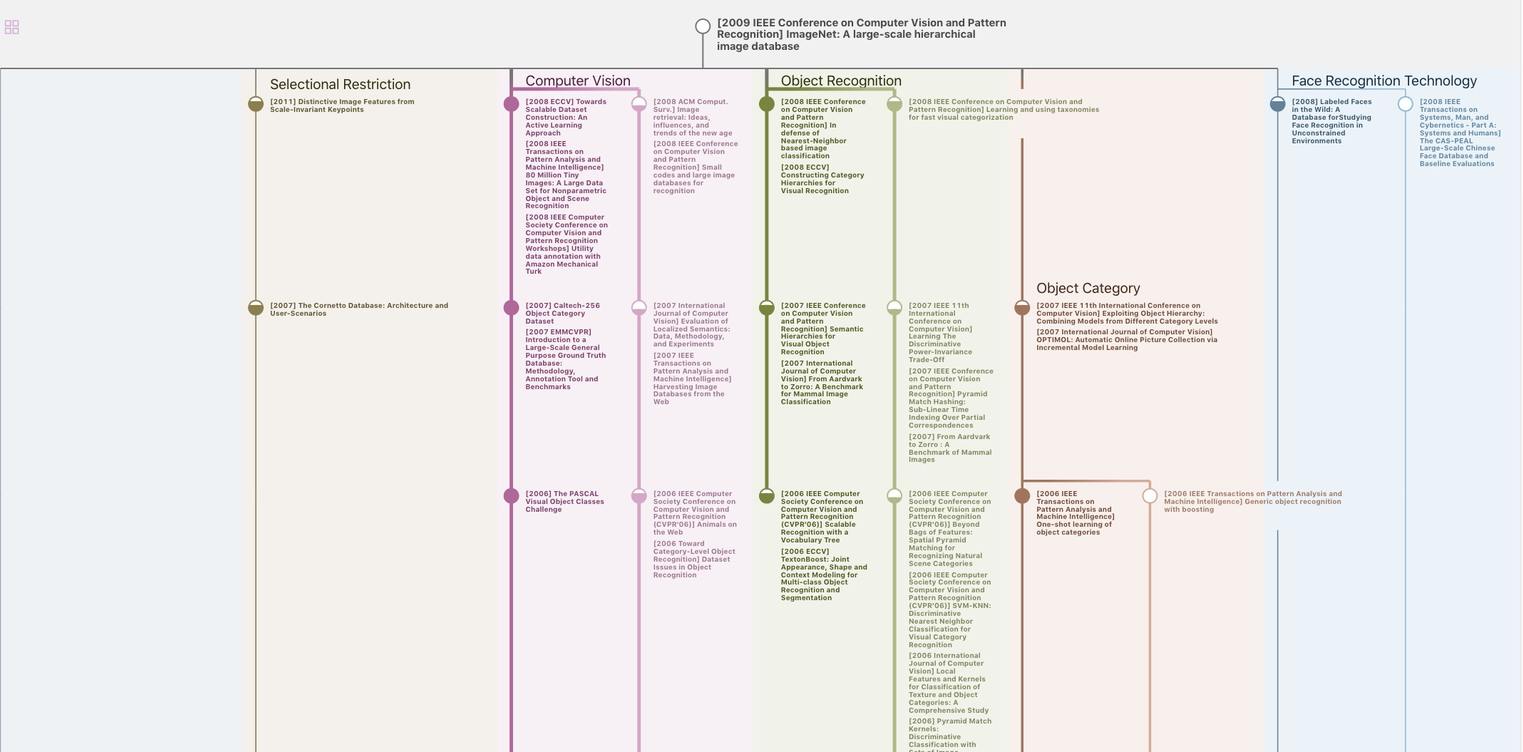
生成溯源树,研究论文发展脉络
Chat Paper
正在生成论文摘要