M2M - Learning to Enhance Low-Light Image from Model to Mobile FPGA.
CGI(2021)
摘要
With the development of convolutional neural networks, the effectiveness of low-light image enhancement techniques have been greatly advanced. However, the calculate-intensive and memory-intensive characteristics of convolutional neural networks make them difficult to be implemented in mobile platform with low power and limited bandwidth. This paper proposes a complete solution for low-light image enhancement from CNN model to mobile (M2M) FPGA. The proposed solution utilizes a pseudo-symmetry quantization method to compress the low-light image enhancement model, and an accelerator to permit the processing ability of the system significantly. We implemented the whole system on a customized FPGA SOC platform (a low-cost chip, Xilinx Inc. ZYNQ(TM) XC7Z035). Extensive experiments show that our method achieved competitive results with the other three platforms, i.e. achieved better speed compare to ARM and CPU; and achieved better power efficiency compared to ARM, CPU, and GPU.
更多查看译文
关键词
image,model,low-light
AI 理解论文
溯源树
样例
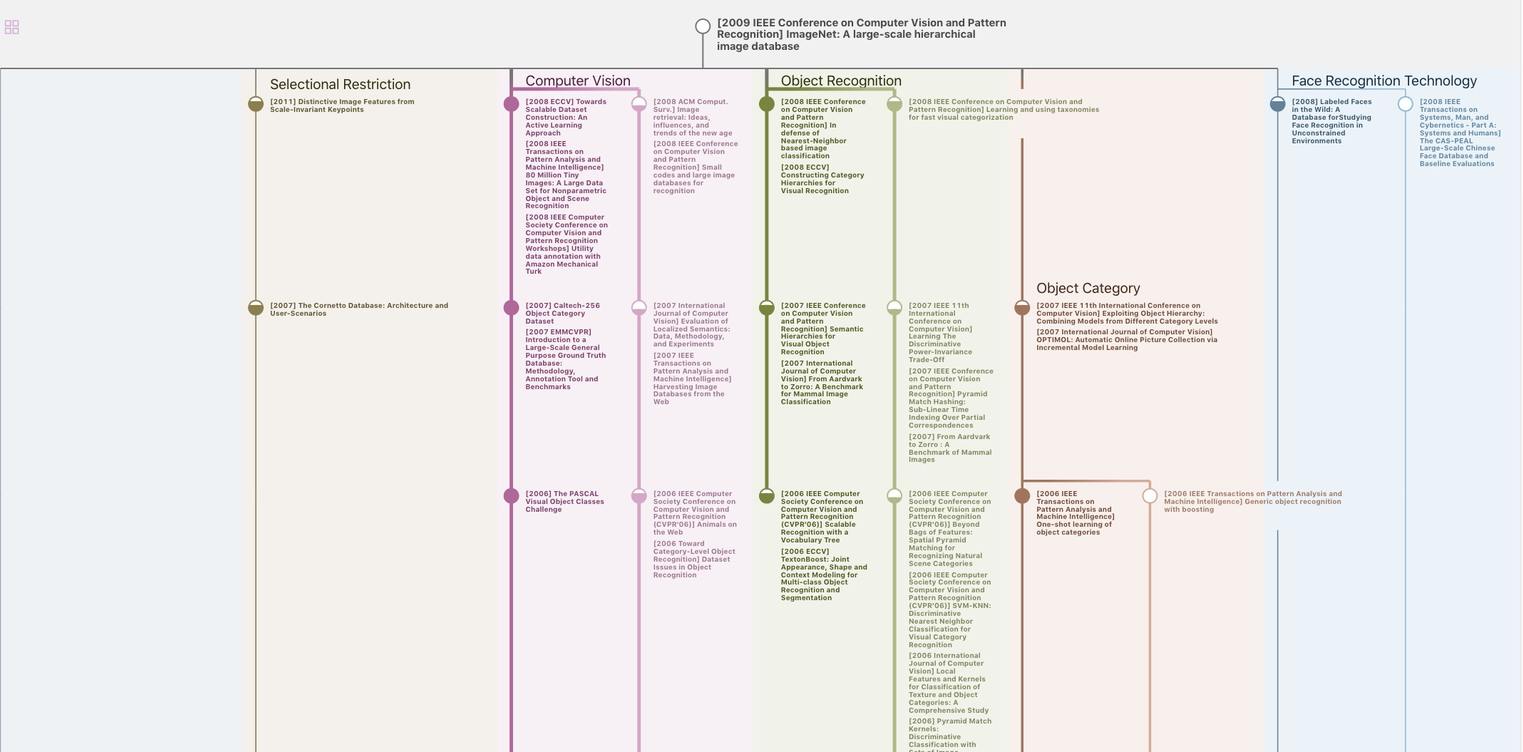
生成溯源树,研究论文发展脉络
Chat Paper
正在生成论文摘要