Continual Learning Using Lattice-Free MMI for Speech Recognition
IEEE International Conference on Acoustics, Speech, and Signal Processing (ICASSP)(2022)
摘要
Continual learning (CL), or domain expansion, recently became a popular topic for automatic speech recognition (ASR) acoustic modeling because practical systems have to be updated frequently in order to work robustly on types of speech not observed during initial training. While sequential adaptation allows tuning a system to a new domain, it may result in performance degradation on the old domains due to catastrophic forgetting. In this work we explore regularization-based CL for neural network acoustic models trained with the lattice-free maximum mutual information (LF-MMI) criterion. We simulate domain expansion by incrementally adapting the acoustic model on different public datasets that include several accents and speaking styles. We investigate two well-known CL techniques, elastic weight consolidation (EWC) and learning without forgetting (LWF), which aim to reduce forgetting by preserving model weights or network outputs. We additionally introduce a sequence-level LWF regularization, which exploits posteriors from the denominator graph of LF-MMI to further reduce forgetting. Empirical results show that the proposed sequence-level LWF can improve the best average word error rate across all domains by up to 9.4% relative compared with using regular LWF.
更多查看译文
关键词
Automatic Speech Recognition,Continual Learning,Domain Expansion,Lattice-free MMI,Learning Without Forgetting
AI 理解论文
溯源树
样例
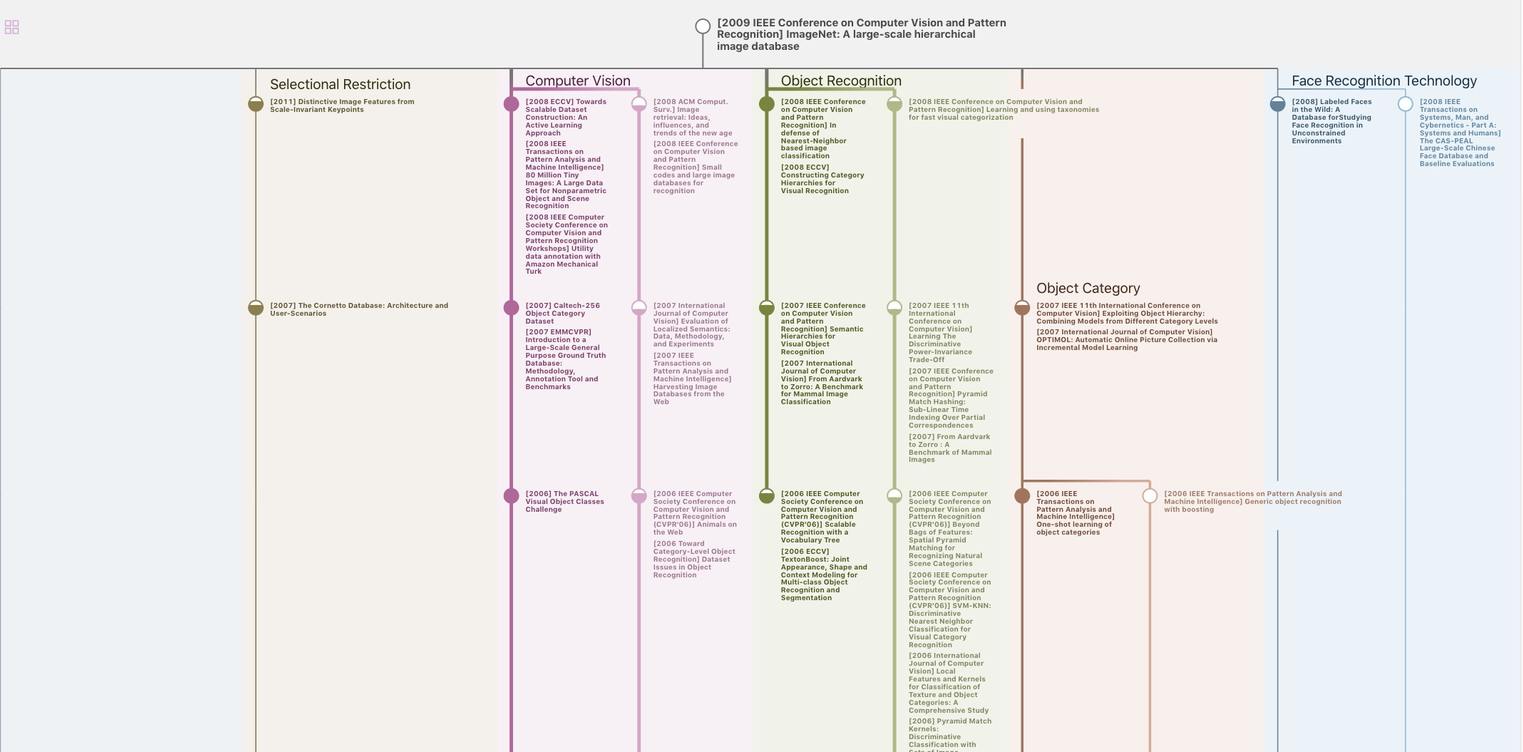
生成溯源树,研究论文发展脉络
Chat Paper
正在生成论文摘要