Multi-ACCDOA: Localizing And Detecting Overlapping Sounds From The Same Class With Auxiliary Duplicating Permutation Invariant Training
ICASSP 2022 - 2022 IEEE International Conference on Acoustics, Speech and Signal Processing (ICASSP)(2022)
摘要
Sound event localization and detection (SELD) involves identifying the direction-of-arrival (DOA) and the event class. The SELD methods with a class-wise output format make the model predict activities of all sound event classes and corresponding locations. The class-wise methods can output activity-coupled Cartesian DOA (ACCDOA) vectors, which enable us to solve a SELD task with a single target using a single network. However, there is still a challenge in detecting the same event class from multiple locations. To overcome this problem while maintaining the advantages of the class-wise format, we extended ACCDOA to a multi one and proposed auxiliary duplicating permutation invariant training (ADPIT). The multi-ACCDOA format (a class- and track-wise output format) enables the model to solve the cases with overlaps from the same class. The class-wise ADPIT scheme enables each track of the multi-ACCDOA format to learn with the same target as the single-ACCDOA format. In evaluations with the DCASE 2021 Task 3 dataset, the model trained with the multi-ACCDOA format and with the class-wise ADPIT detects overlapping events from the same class while maintaining its performance in the other cases. Also, the proposed method performed comparably to state-of-the-art SELD methods with fewer parameters.
更多查看译文
关键词
Sound event localization and detection,activity-coupled Cartesian direction of arrival,permutation invariant training
AI 理解论文
溯源树
样例
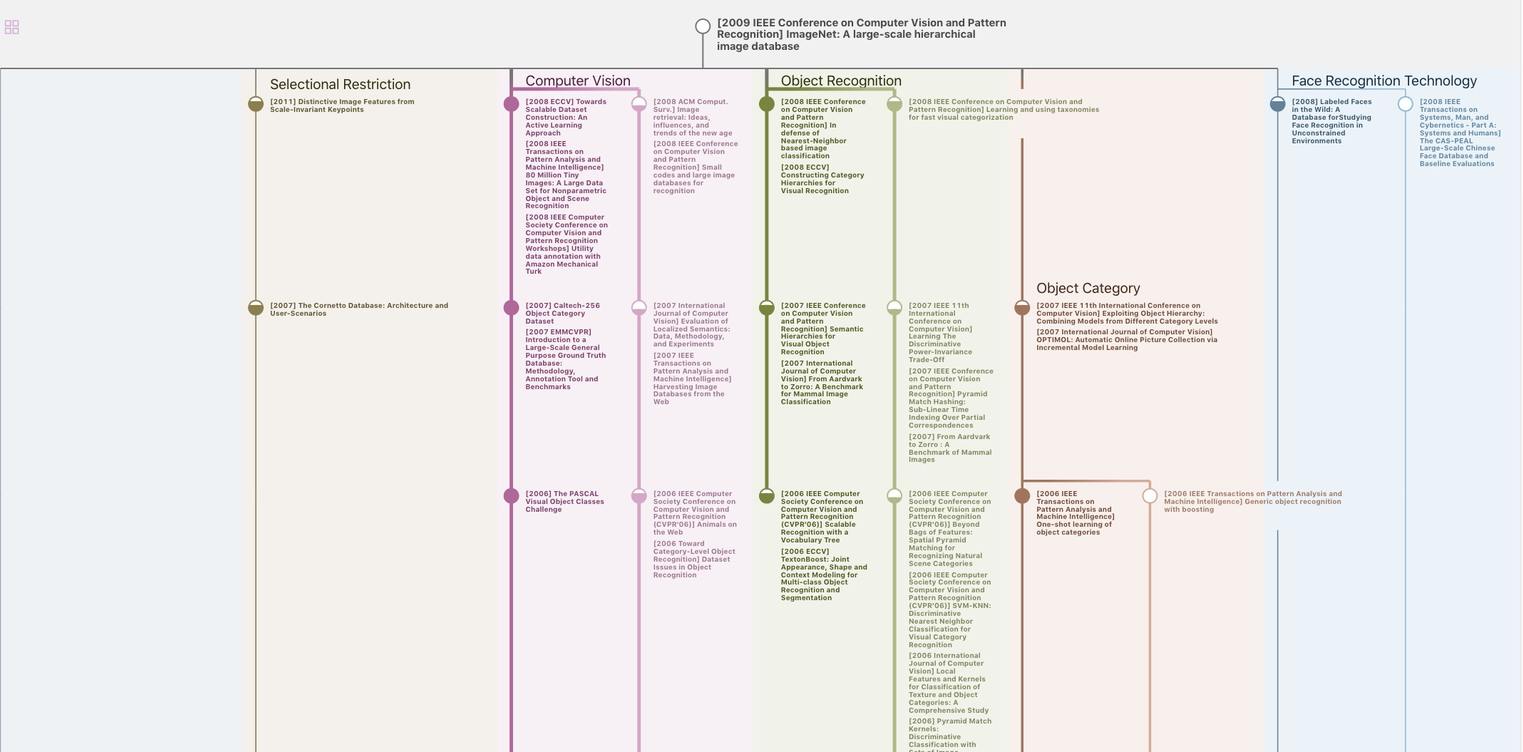
生成溯源树,研究论文发展脉络
Chat Paper
正在生成论文摘要