Bandwidth Utilization Side-Channel on ML Inference Accelerators
CoRR(2021)
Abstract
Accelerators used for machine learning (ML) inference provide great performance benefits over CPUs. Securing confidential model in inference against off-chip side-channel attacks is critical in harnessing the performance advantage in practice. Data and memory address encryption has been recently proposed to defend against off-chip attacks. In this paper, we demonstrate that bandwidth utilization on the interface between accelerators and the weight storage can serve a side-channel for leaking confidential ML model architecture. This side channel is independent of the type of interface, leaks even in the presence of data and memory address encryption and can be monitored through performance counters or through bus contention from an on-chip unprivileged process.
MoreTranslated text
Key words
ml inference accelerators,utilization,side-channel
AI Read Science
Must-Reading Tree
Example
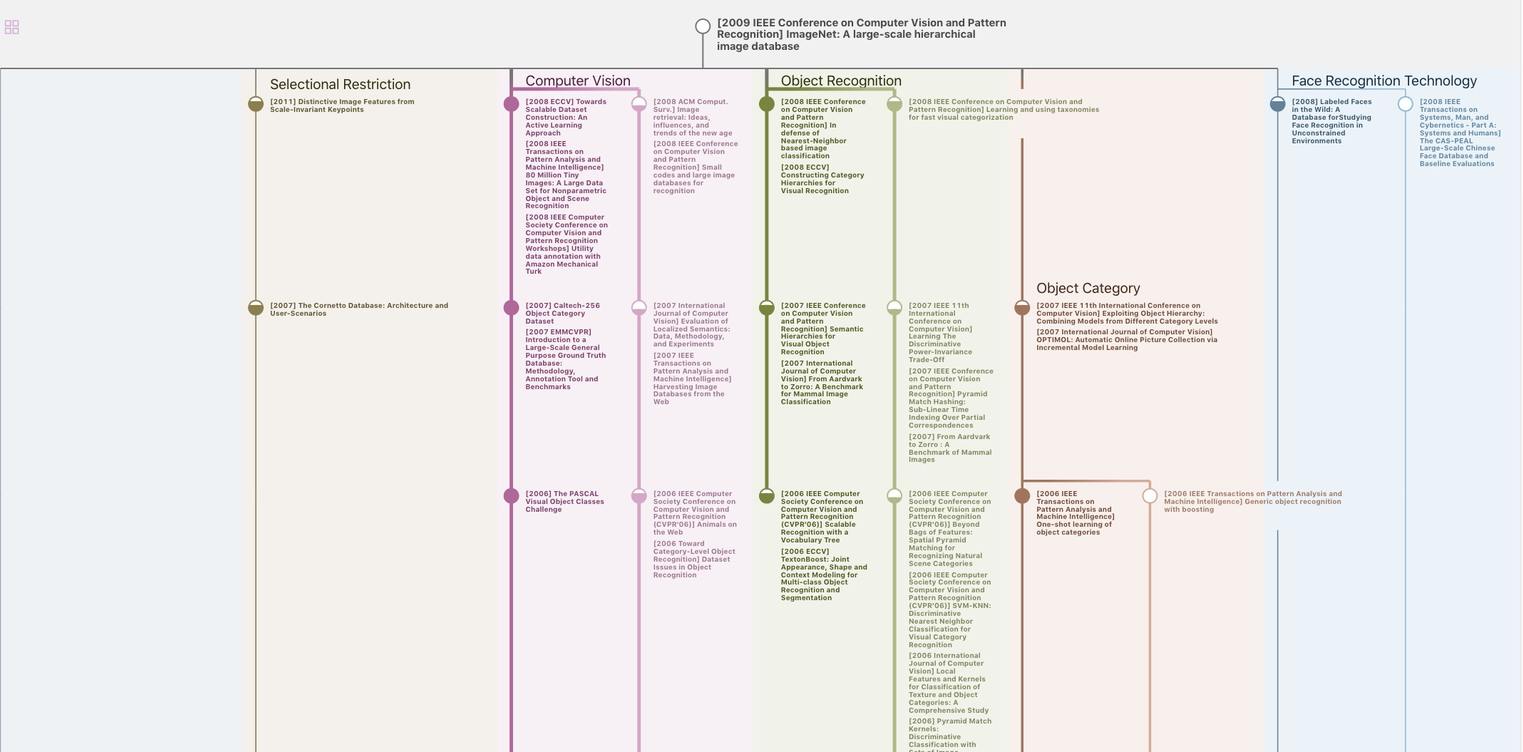
Generate MRT to find the research sequence of this paper
Chat Paper
Summary is being generated by the instructions you defined