Conformer-Based Self-Supervised Learning For Non-Speech Audio Tasks.
IEEE International Conference on Acoustics, Speech, and Signal Processing (ICASSP)(2022)
摘要
Representation learning from unlabeled data has been of major interest in artificial intelligence research. While self-supervised speech representation learning has been popular in the speech research community, very few works have comprehensively analyzed audio representation learning for non-speech audio tasks. In this paper, we propose a self-supervised audio representation learning method and apply it to a variety of downstream non-speech audio tasks. We combine the well-known wav2vec 2.0 framework, which has shown success in self-supervised learning for speech tasks, with parameter-efficient conformer architectures. On the AudioSet benchmark, we achieve a mean average precision (mAP) score of 0.415, which is a new state-of-the-art on this dataset through audio-only self-supervised learning. Our fine-tuned conformers also surpass or match the performance of previous systems pre-trained in a supervised way on several downstream tasks. We further discuss the important design considerations for both pre-training and fine-tuning.
更多查看译文
关键词
Self-supervised learning,representation learning,conformer,sound events,wav2vec
AI 理解论文
溯源树
样例
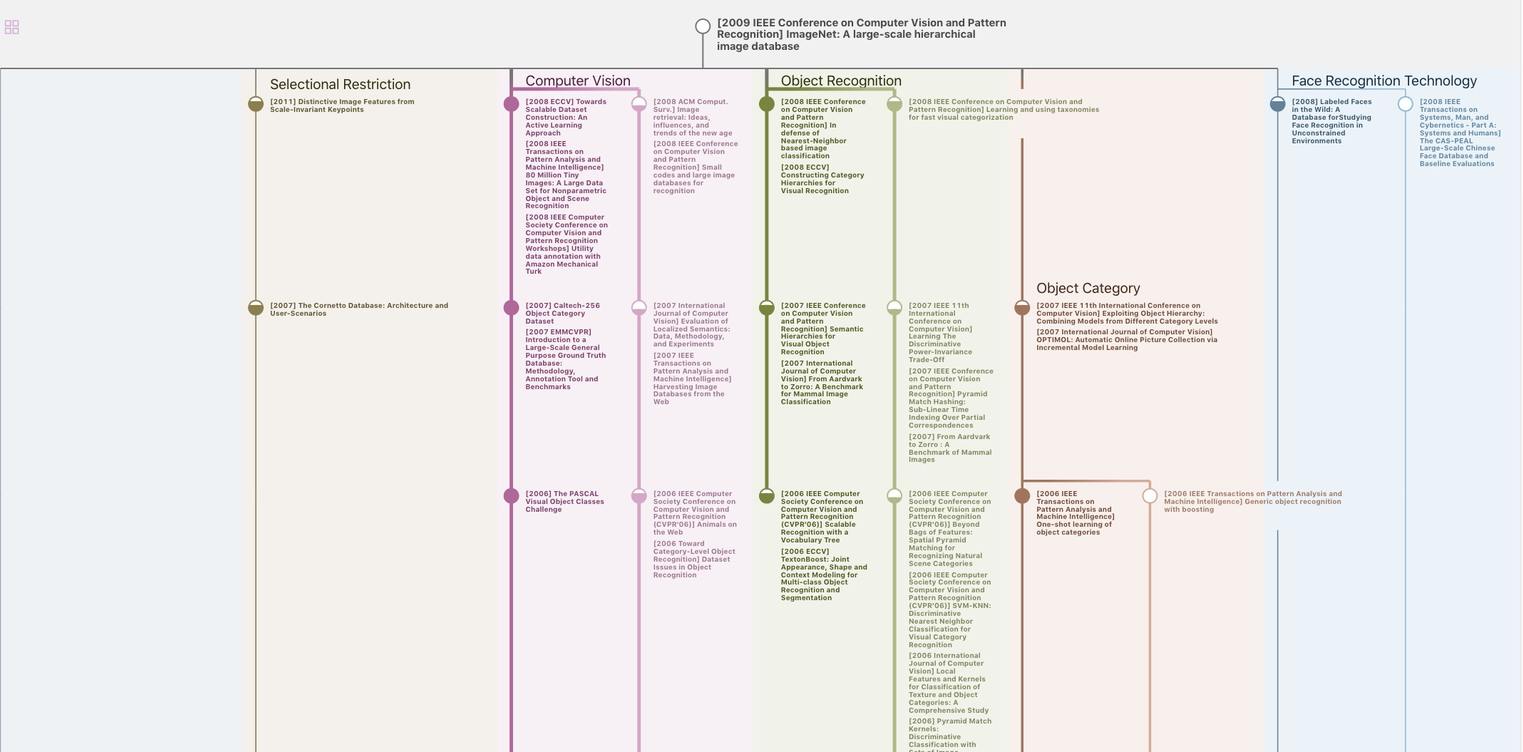
生成溯源树,研究论文发展脉络
Chat Paper
正在生成论文摘要