E2DNet: An Ensembling Deep Neural Network for Solving Nonconvex Economic Dispatch in Smart Grid
user-6144298de55422cecdaf68a5(2021)
摘要
Currently, nonconvex economic dispatch problem is one of the research focuses in the field of smart grid. A variety of algorithms are developed to solve it. However, these algorithms are prone to suffering from high computation cost and slow convergence rate, which creates an inevitable gap between theoretical analysis and practical real-time operations. In this paper, we aim at providing an ensemble deep learning based approach to tackle such a challenging issue. Firstly, a novel ensemble method is presented to explore the ground truth of nonconvex economic dispatch problems. Secondly, considering the time-varying total load demand, cost coefficients and dispatchability of all generation units in a practical SG system as the features, a new deep neural network structure is proposed to learn the complex mapping from instant features to an optimal nonconvex economic dispatch solution. If such a mapping is well approximated by the designed deep neural network, no significant effort is required to solve a new economic dispatch problem and the solution is obtained on the scale of milliseconds. Thirdly, analyzing that a single deep neural network may be weak to a small part of the mapping space of the nonconvex economic dispatch problem, we further present an ensemble of multiple parallel deep neural networks trained sequentially with a simplified Adaboost.R2 algorithm. Finally, case studies reveal that the proposed approach achieves orders of magnitude speedup in computational time while guaranteeing similar or better performance on minimizing the overall generation cost compared to the state-of-the-art nonconvex economic dispatch algorithms.
更多查看译文
AI 理解论文
溯源树
样例
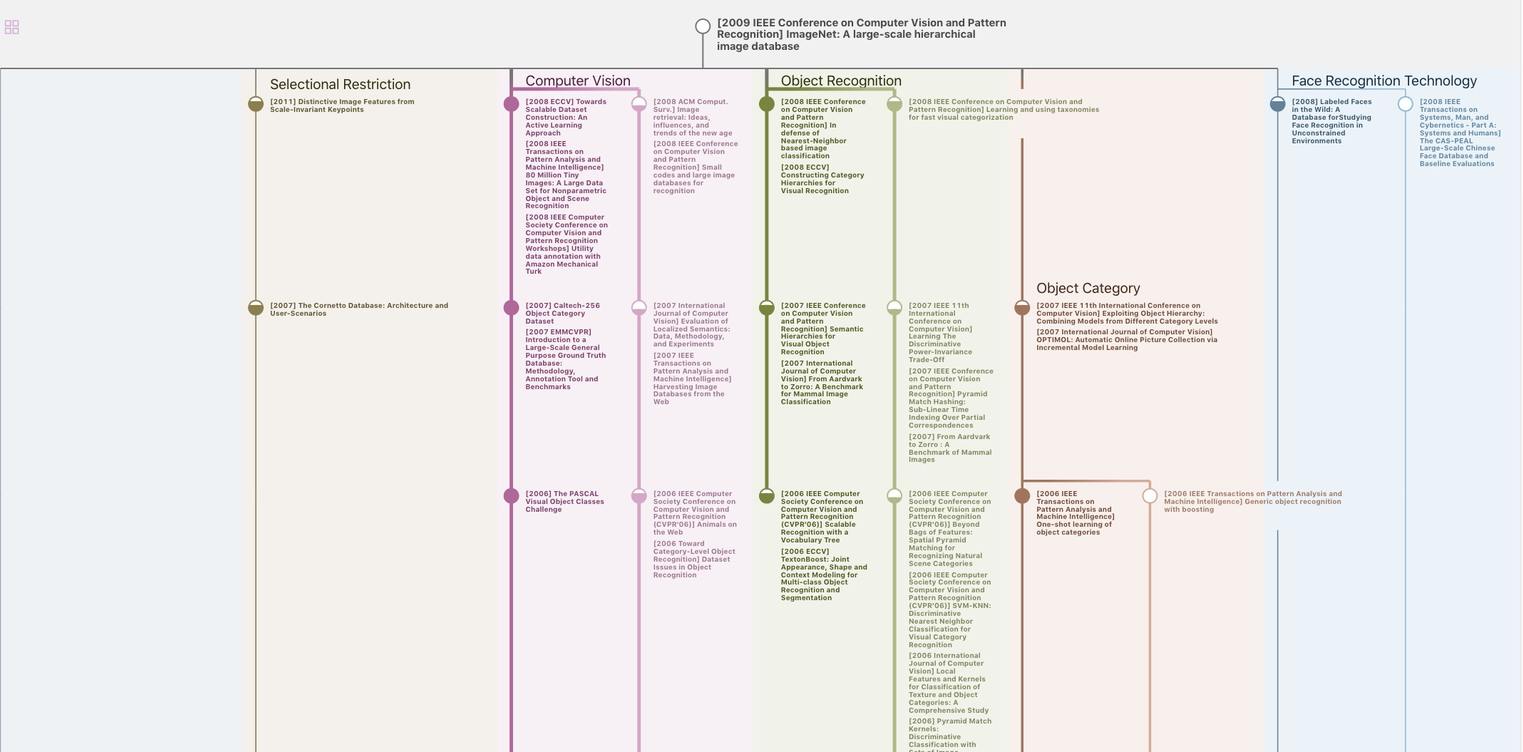
生成溯源树,研究论文发展脉络
Chat Paper
正在生成论文摘要