Towards Out-of-Distribution Detection for Remote Sensing.
IGARSS(2021)
摘要
In remote sensing, distributional mismatch between the training and test data may arise due to several reasons, including unseen classes in the test data, differences in the geographic area, and multi-sensor differences. Deep learning based models may behave in unexpected manners when subjected to test data that has such distributional shifts from the training data, also called out-of-distribution (OOD) examples. Vulnerability to OOD data severely reduces the reliability of deep learning based models. In this work, we address this issue by proposing a model to quantify distributional uncertainty of deep learning based remote sensing models. In particular, we adopt a Dirichlet Prior Network for remote sensing data. The approach seeks to maximize the representation gap between the in-domain and OOD examples for a better identification of unknown examples at test time. Experimental results on three exemplary test scenarios show that the proposed model can detect OOD images in remote sensing.
更多查看译文
关键词
Out-of-distribution,open set recognition,robustness,remote sensing
AI 理解论文
溯源树
样例
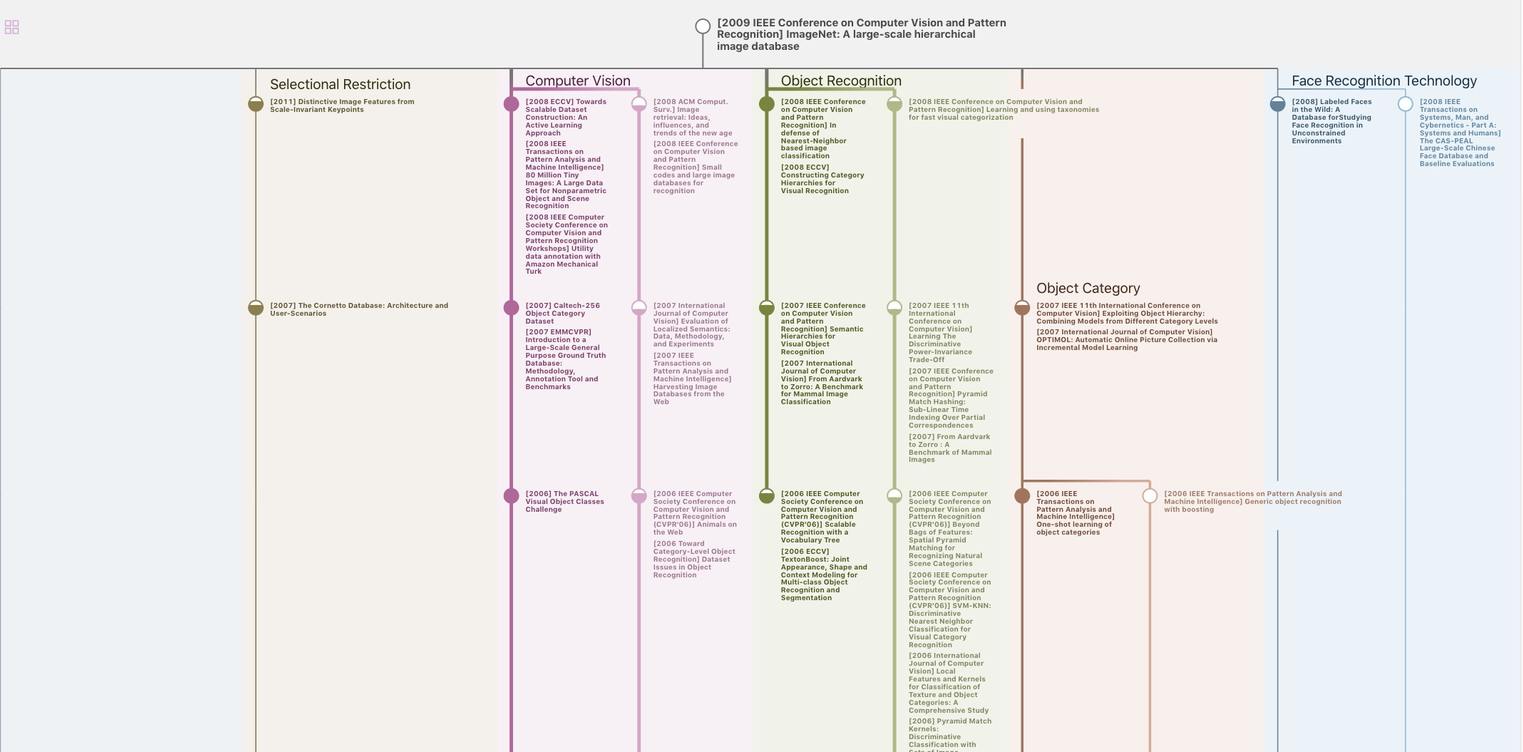
生成溯源树,研究论文发展脉络
Chat Paper
正在生成论文摘要