Theory and software for boosted nonparametric hazard estimation.
AAAI Symposium on Survival Prediction - Algorithms, Challenges and Applications (SPACA)(2021)
摘要
Nonparametric approaches for analyzing survival data in the presence of time-dependent covariates is a timely topic, given the availability of high frequency data capture systems in healthcare and beyond. We present a theoretically justified gradient boosted hazard estimator for this setting, and describe a tree-based implementation called BoXHED (pronounced ‘box-head’) that is available from GitHub: www.github.com/BoXHED. Our numerical study demonstrates that there is a place in the machine learning toolbox for a nonparametric method like BoXHED that can flexibly handle time-dependent covariates. The results presented here are distilled from the recent works of Lee et al. (2021) and Wang et al. (2020).
更多查看译文
AI 理解论文
溯源树
样例
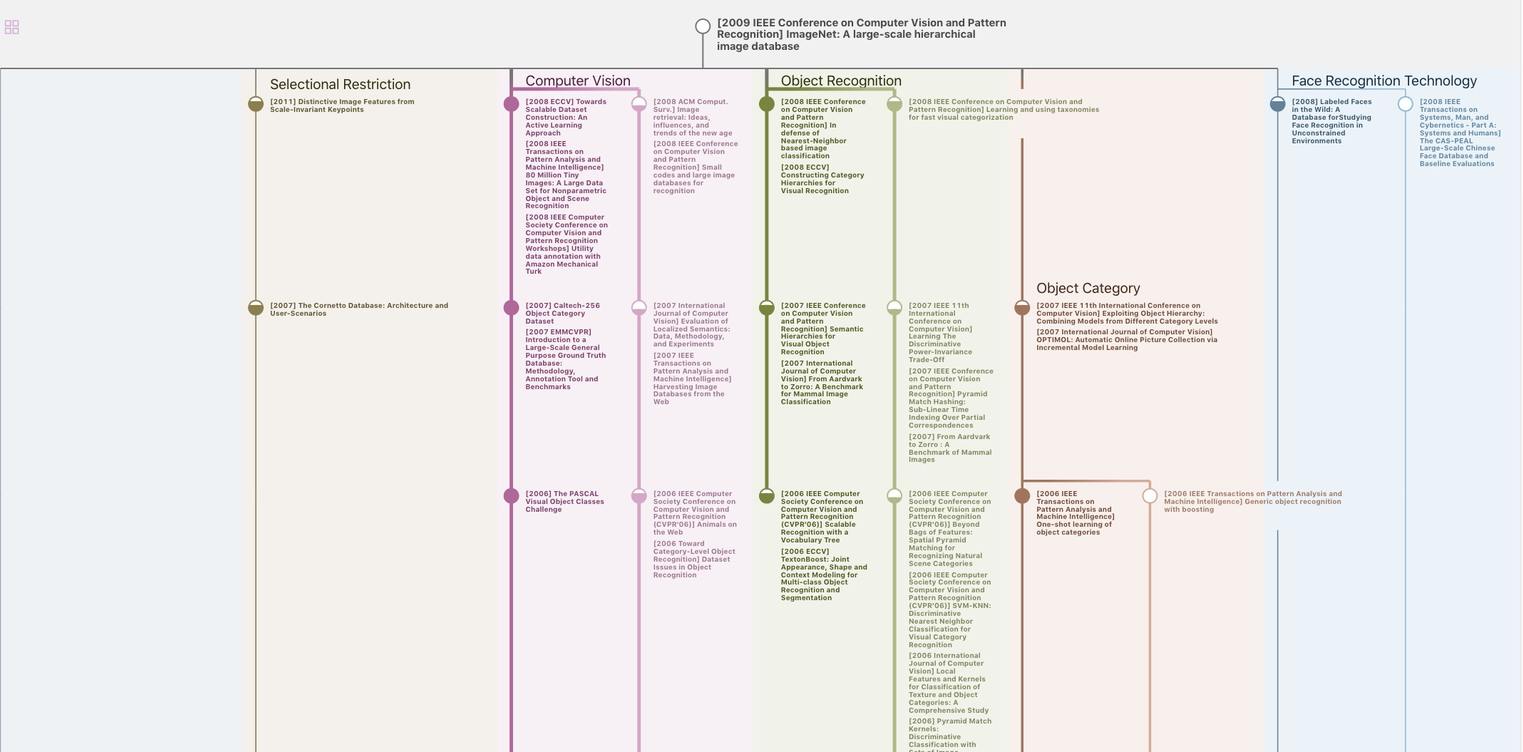
生成溯源树,研究论文发展脉络
Chat Paper
正在生成论文摘要