Variance Reduction in Stochastic Methods for Large-Scale Regularized Least-Squares Problems
European Signal Processing Conference (EUSIPCO)(2022)
摘要
Large dimensional least-squares and regularized least-squares problems are expensive to solve. There exist many approximate techniques, some deterministic (like conjugate gradient), some stochastic (like stochastic gradient descent). Among the latter, a new class of techniques uses Determinantal Point Processes (DPPs) to produce unbiased estimators of the solution. In particular, they can be used to perform Tikhonov regularization on graphs using random spanning forests, a specific DPP. While the unbiasedness of these algorithms is attractive, their variance can be high. We show here that variance can be reduced by combining the stochastic estimator with a deterministic gradient-descent step, while keeping the property of unbiasedness. We apply this technique to Tikhonov regularization on graphs, where the reduction in variance is found to be substantial at very small extra cost.
更多查看译文
关键词
graph signal processing, smoothing, variance reduction, random spanning forests
AI 理解论文
溯源树
样例
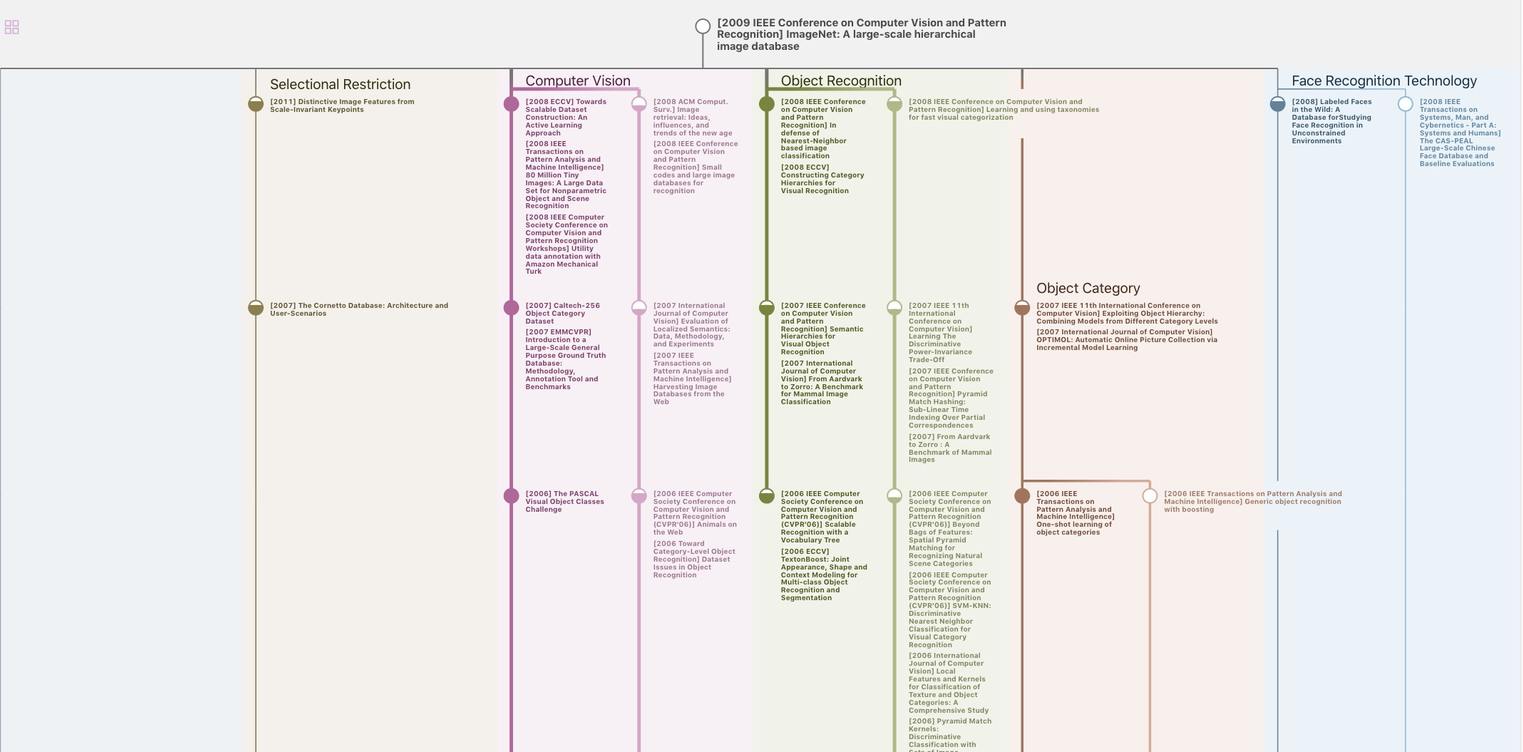
生成溯源树,研究论文发展脉络
Chat Paper
正在生成论文摘要