Breaking Down Multilingual Machine Translation
FINDINGS OF THE ASSOCIATION FOR COMPUTATIONAL LINGUISTICS (ACL 2022)(2022)
摘要
While multilingual training is now an essential ingredient in machine translation (MT) systems, recent work has demonstrated that it has different effects in different multilingual settings, such as many-to-one, one-to-many, and many-to-many learning. These training settings expose the encoder and the decoder in a machine translation model with different data distributions. In this paper, we examine how different varieties of multilingual training contribute to learning these two components of the MT model. Specifically, we compare bilingual models with encoders and/or decoders initialized by multilingual training. We show that multilingual training is beneficial to encoders in general, while it only benefits decoders for low-resource languages (LRLs). We further find the important attention heads for each language pair and compare their correlations during inference. Our analysis sheds light on how multilingual translation models work and enables us to propose methods to improve performance by training with highly related languages. Our many-to-one models for high-resource languages and one-to-many models for LRL outperform the best results reported by Aharoni et al. (2019).
更多查看译文
关键词
translation,breaking
AI 理解论文
溯源树
样例
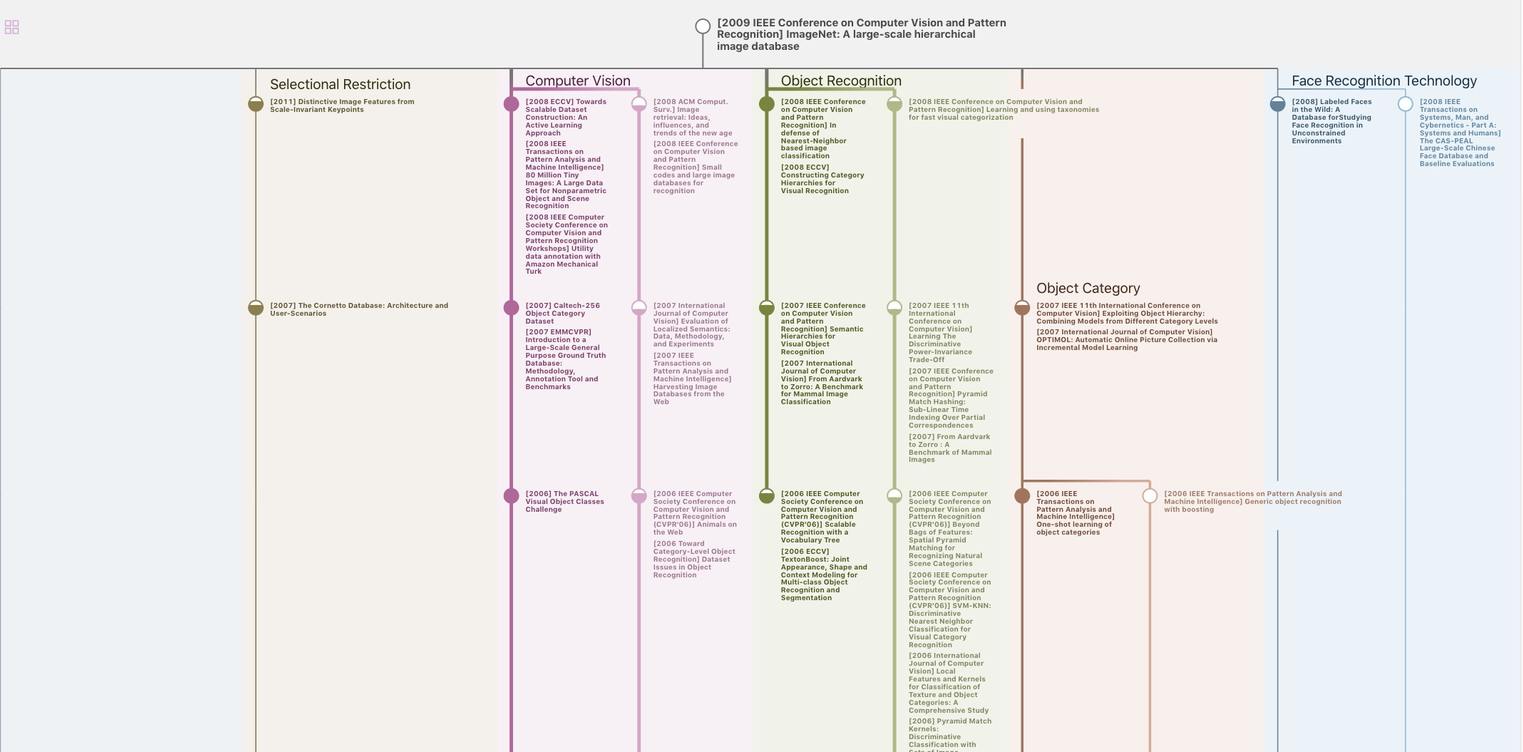
生成溯源树,研究论文发展脉络
Chat Paper
正在生成论文摘要