PredictionNet: Real-Time Joint Probabilistic Traffic Prediction for Planning, Control, and Simulation
IEEE International Conference on Robotics and Automation(2022)
摘要
Predicting the future motion of traffic agents is crucial for safe and efficient autonomous driving. To this end, we present PredictionNet, a deep neural network (DNN) that predicts the motion of all surrounding traffic agents together with the ego-vehicle's motion. All predictions are probabilistic and are represented in a simple top-down rasterization that allows an arbitrary number of agents. Conditioned on a multi-layer map with lane information, the network outputs future positions, velocities, and backtrace vectors jointly for all agents including the ego-vehicle in a single pass. Trajectories are then extracted from the output. The network can be used to simulate realistic traffic, and it produces competitive results on popular benchmarks. More importantly, it has been used to successfully control a real-world vehicle for hundreds of kilometers, by combining it with a motion planning/control subsystem. The network runs faster than real-time on an embedded GPU, and the system shows good generalization (across sensory modalities and locations) due to the choice of input representation. Furthermore, we demonstrate that by extending the DNN with reinforcement learning (RL), it can better handle rare or unsafe events like aggressive maneuvers and crashes.
更多查看译文
关键词
DNN,PredictionNet,real-time joint probabilistic traffic prediction,future motion,safe driving,efficient autonomous driving,deep neural network,surrounding traffic agents,ego-vehicle,simple top-down rasterization,arbitrary number,multilayer map,lane information,future positions,realistic traffic,real-world vehicle
AI 理解论文
溯源树
样例
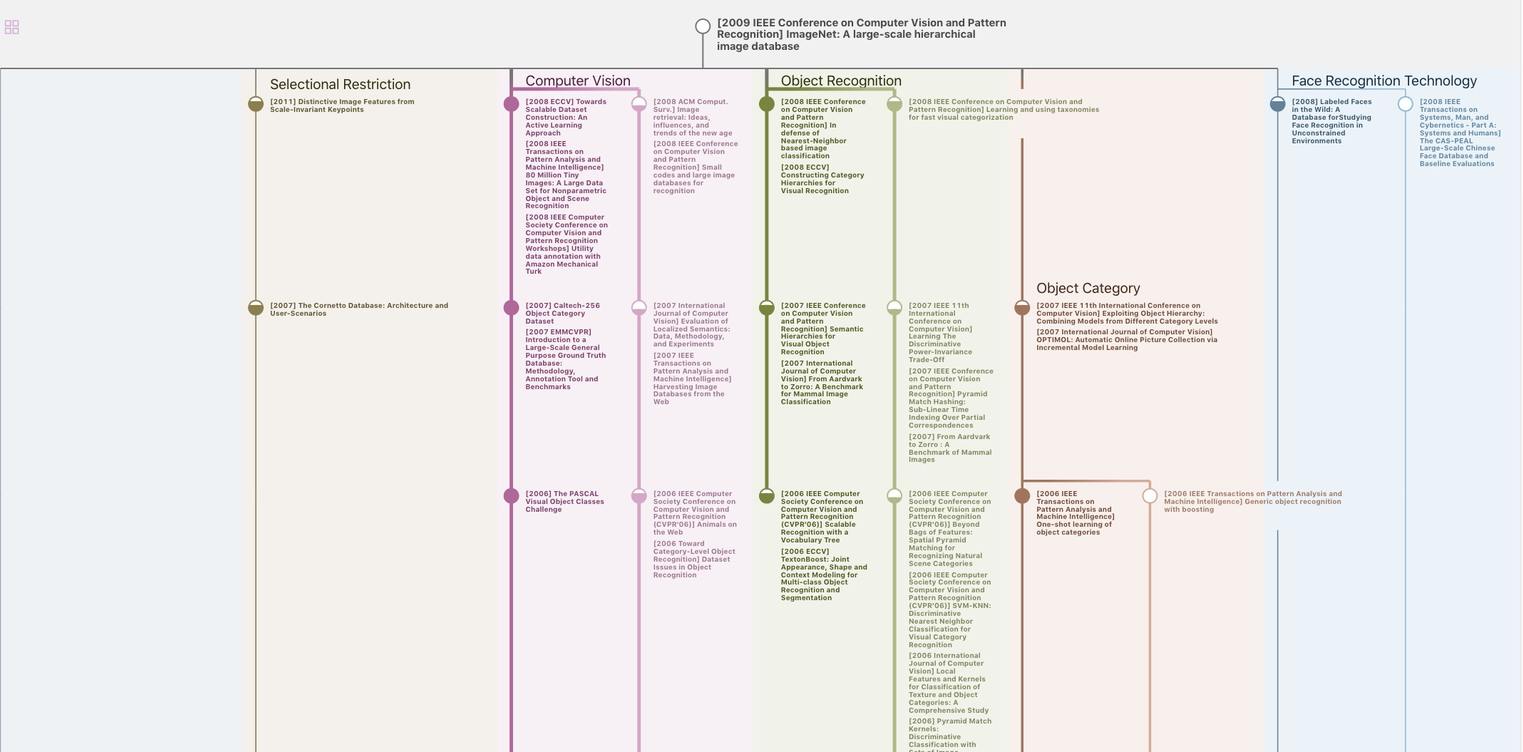
生成溯源树,研究论文发展脉络
Chat Paper
正在生成论文摘要