Deepest Cuts for Benders Decomposition
arxiv(2021)
摘要
Since its inception, Benders Decomposition (BD) has been successfully applied to a wide range of large-scale mixed-integer (linear) problems. The key element of BD is the derivation of Benders cuts, which are often not unique. In this paper, we introduce a novel unifying Benders cut selection technique based on a geometric interpretation of cut ``depth'', produce deepest Benders cuts based on $\ell_p$-norms, and study their properties. Specifically, we show that deepest cuts resolve infeasibility through minimal deviation from the incumbent point, are relatively sparse, and may produce optimality cuts even when classical Benders would require a feasibility cut. Leveraging the duality between separation and projection, we develop a Guided Projections Algorithm for producing deepest cuts while exploiting the combinatorial structure or decomposablity of problem instances. We then propose a generalization of our Benders separation problem that brings several well-known cut selection strategies under one umbrella. In particular, we provide systematic ways of selecting the normalization coefficients in the Minimal Infeasible Subsystems method by establishing its connection to our method. Finally, in our tests on facility location problems, we show deepest cuts often reduce both runtime and number of Benders iterations, as compared to other cut selection strategies; and relative to classical Benders, use $1/3$ the number of cuts and $1/2$ the runtime.
更多查看译文
关键词
deepest cuts,decomposition
AI 理解论文
溯源树
样例
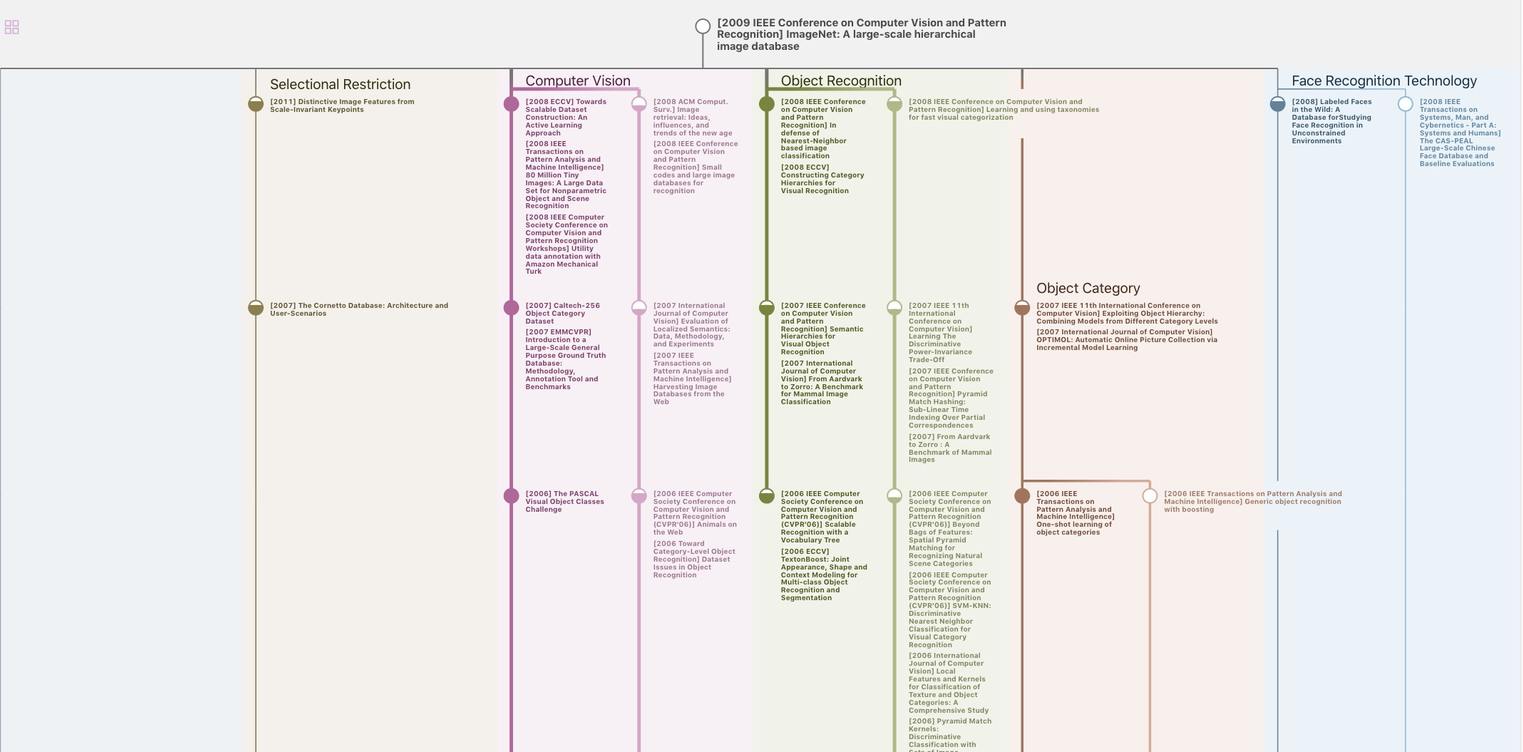
生成溯源树,研究论文发展脉络
Chat Paper
正在生成论文摘要