Learning in High Dimension Always Amounts to Extrapolation
arxiv(2021)
摘要
The notion of interpolation and extrapolation is fundamental in various fields from deep learning to function approximation. Interpolation occurs for a sample $x$ whenever this sample falls inside or on the boundary of the given dataset's convex hull. Extrapolation occurs when $x$ falls outside of that convex hull. One fundamental (mis)conception is that state-of-the-art algorithms work so well because of their ability to correctly interpolate training data. A second (mis)conception is that interpolation happens throughout tasks and datasets, in fact, many intuitions and theories rely on that assumption. We empirically and theoretically argue against those two points and demonstrate that on any high-dimensional ($>$100) dataset, interpolation almost surely never happens. Those results challenge the validity of our current interpolation/extrapolation definition as an indicator of generalization performances.
更多查看译文
关键词
extrapolation,learning,high dimension
AI 理解论文
溯源树
样例
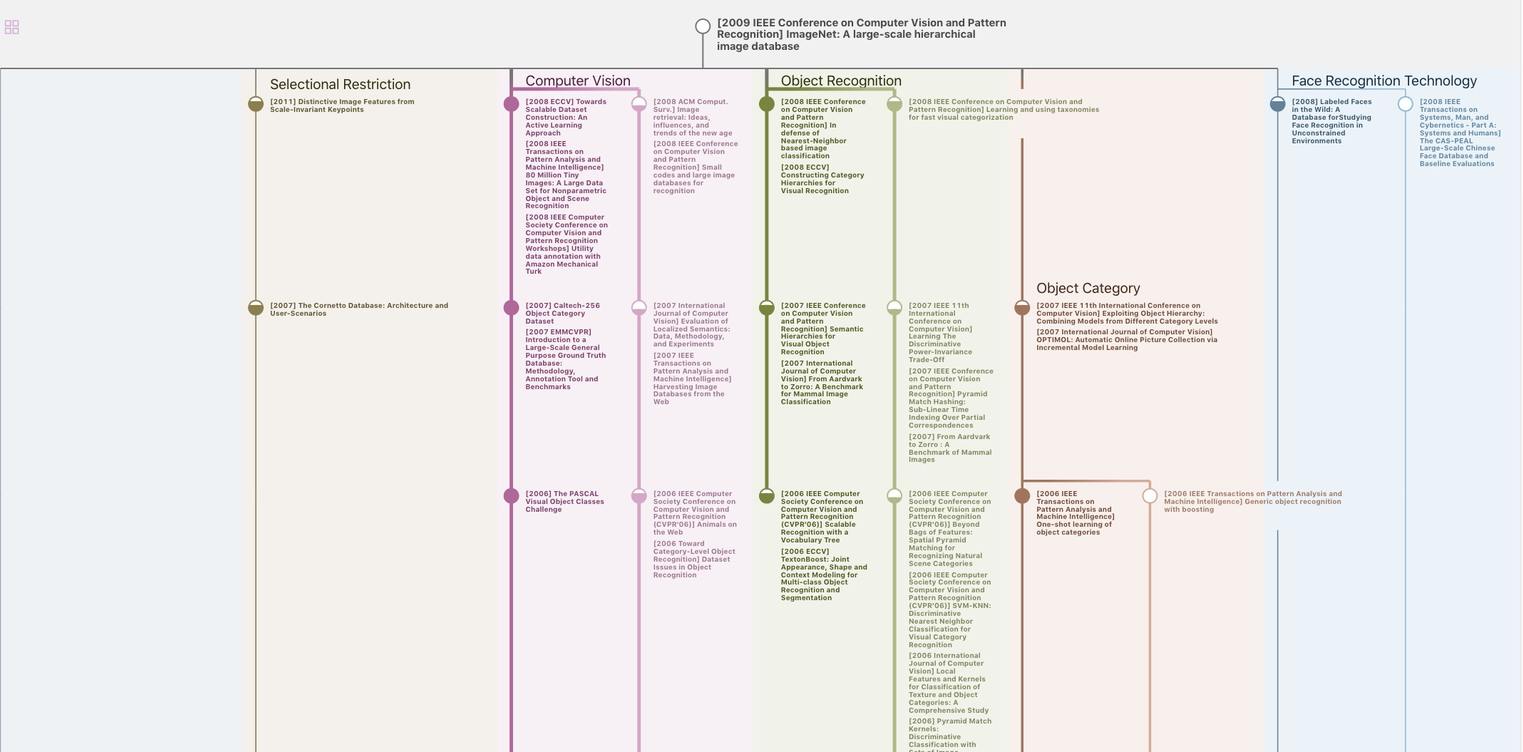
生成溯源树,研究论文发展脉络
Chat Paper
正在生成论文摘要