Deep Learning to Predict the Feasibility of Priority-Based Ethernet Network Configurations
ACM transactions on cyber-physical systems(2021)
摘要
Machine learning has been recently applied in real-time systems to predict whether Ethernet network configurations are feasible in terms of meeting deadline constraints without executing conventional schedulability analysis. However, the existing prediction techniques require domain expertise to choose the relevant input features and do not perform consistently when topologies or traffic patterns differ significantly from the ones in the training data. To overcome these problems, we propose a Graph Neural Network (GNN) prediction model that synthesizes relevant features directly from the raw data. This deep learning model possesses the ability to exploit relations among flows, links, and queues in switched Ethernet networks and generalizes to unseen topologies and traffic patterns. We also explore the use of ensembles of GNNs and show that it enhances the robustness of the predictions. An evaluation on heterogeneous testing sets comprising realistic automotive networks shows that ensembles of 32 GNN models feature a prediction accuracy ranging from 79.3% to 90% for Ethernet networks using priorities as the Quality-of-Service mechanism. The use of ensemble models provides a speedup factor ranging from 77 to 1,715 compared to schedulability analysis, which allows a far more extensive design space exploration.
更多查看译文
关键词
Machine learning,graph neural network,schedulability analysis,design space exploration,time-sensitive networking
AI 理解论文
溯源树
样例
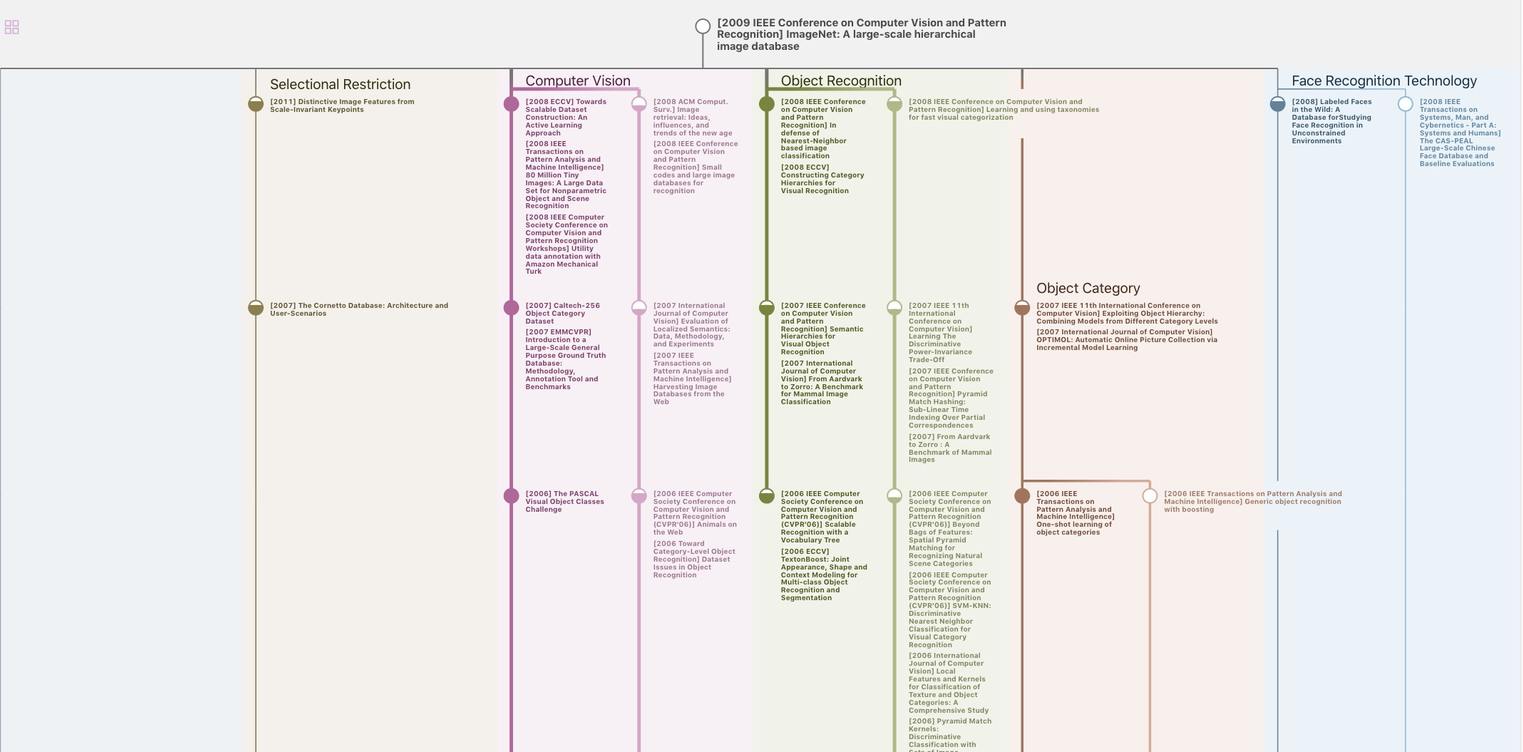
生成溯源树,研究论文发展脉络
Chat Paper
正在生成论文摘要