Improving neural machine translation with latent features feedback
Neurocomputing(2021)
摘要
Most state-of-the-art neural machine translation (NMT) models progressively encode feature representation in a bottom-up feed-forward fashion. This traditional encoding mechanism has no guidance from external signals. In computer vision tasks, the feedback connection plays a crucial role, particularly for understanding tasks. In this paper, we propose a simple but effective approach to learn latent feature representations explicitly from input sentences via a latent feature encoder (LFE), which are fed back to an NMT encoder via a top-down feedback mechanism. Through the feedback mechanism, the representations in one layer are influenced by representations of both lower and higher layers, resulting in a more effective encoding mechanism. Besides, to enhance the capability of the LFE in better capturing latent features from the source sentences, we pre-train the LFE via a Denoising Auto-Encoder (DAE) strategy. Experimentation on the large-scale WMT 2014 English-to-German and WMT 2017 Chinese-to-English translation tasks demonstrates that our proposed LFE, either pre-trained with the DAE or not, significantly outperforms the strong baseline.
更多查看译文
关键词
Neural machine translation,Latent features,Feedback,Transformer
AI 理解论文
溯源树
样例
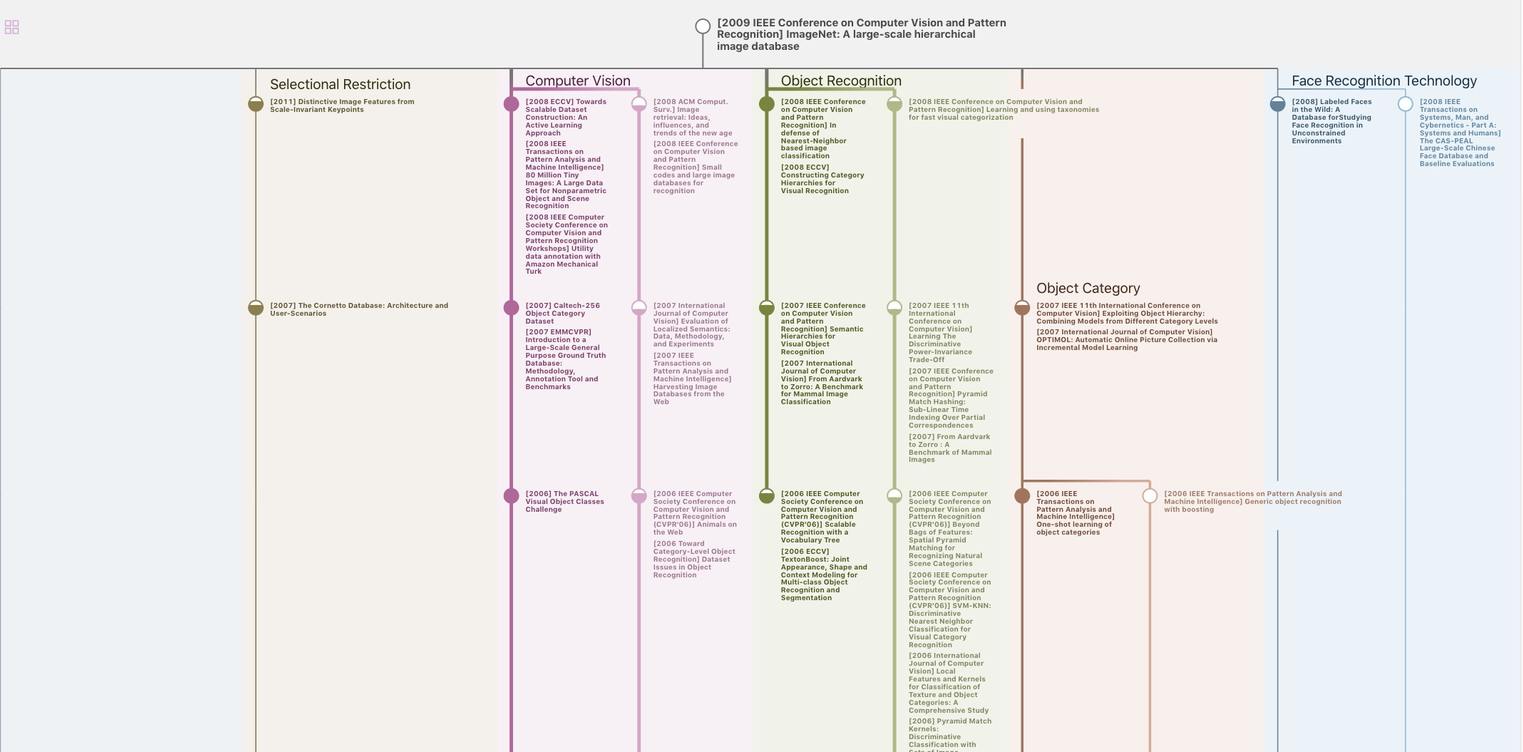
生成溯源树,研究论文发展脉络
Chat Paper
正在生成论文摘要