MRTA - Multi-Resolution Training Algorithm for Multitemporal Semantic Change Detection.
IGARSS(2021)
摘要
The multitemporal semantic change detection challenge track (Track MSD) in the 2021 Data Fusion Contest is to extract the land cover changes of the US state of Maryland from 2013 to 2017, but only low-resolution label data is provided. We present a multi-resolution training algorithm (MRTA) to alleviate the overfitting of the model on the coarse labels. First, using low-resolution coarse labels to train FCN, the average IoU can reach 0.5253. Generating pseudo-labels using this network, they are combined with coarse labels to form a multi-resolution label combination, and perform iterative fine-tuning and retrain. After that, by analyzing the loss and gain indicators of specific categories, it was found that the discrimination effect of the water area was poor, so a strong classifier was trained for the water area. We also implemented strategies such as model voting and weighted training to improve model performance. Finally, our method achieves 0.6445 mIoU on the test set, ranking 3rd in Track 2 of the IEEE Data Fusion Contest of 2021.
更多查看译文
关键词
semantic change detection,pseudo-labels,multi-resolution label
AI 理解论文
溯源树
样例
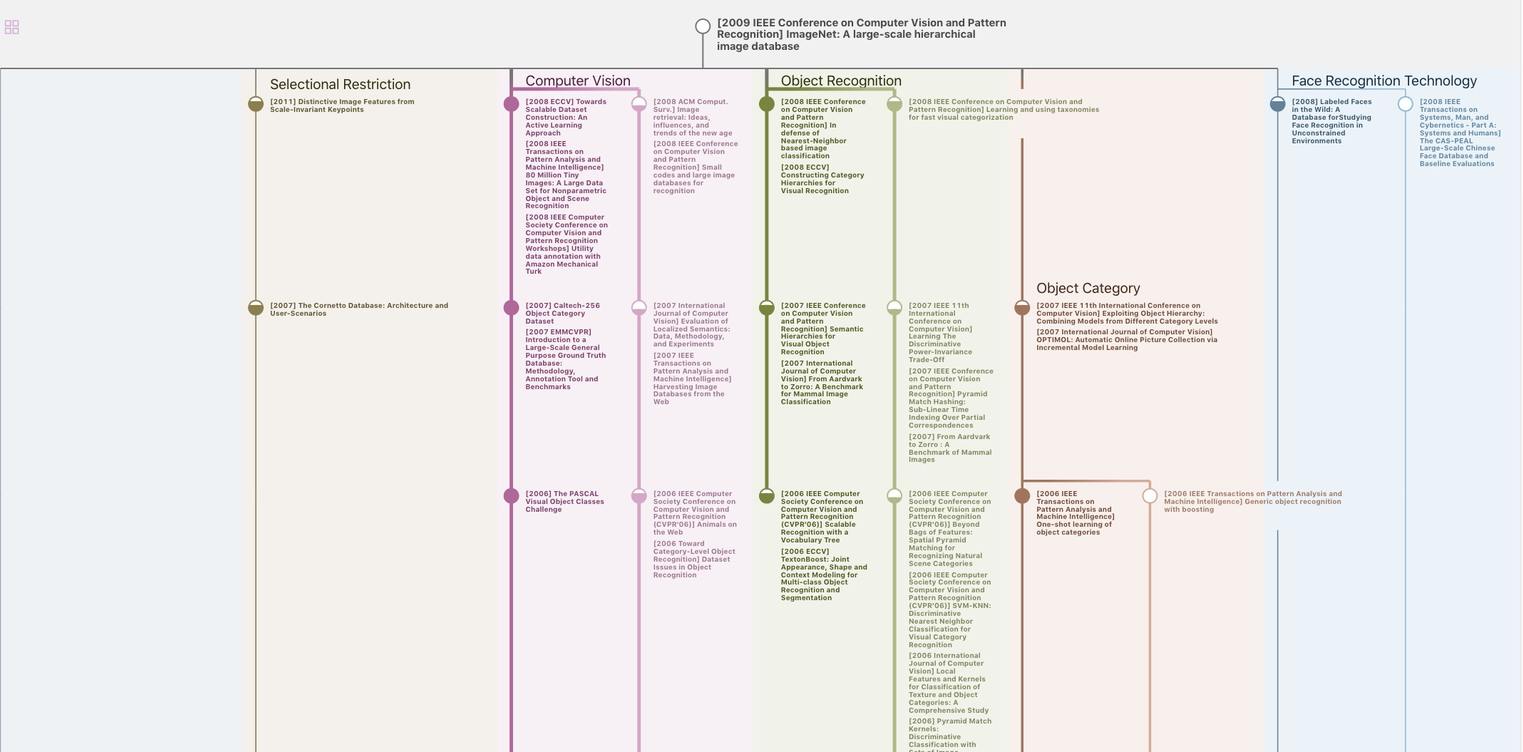
生成溯源树,研究论文发展脉络
Chat Paper
正在生成论文摘要