Bayesian Deep Learning with Monte Carlo Dropout for Qualification of Semantic Segmentation.
IGARSS(2021)
摘要
Despite the intense development of deep neural networks for computer vision, and especially semantic segmentation, their application to Earth Observation data remains usually below accuracy requirements brought by real-life scenarios. Even if well-known deep learning methods produce excellent results, they tend to be over-confident and cannot assess how relevant their predictions are. In this work, a Bayesian deep learning method, based on Monte Carlo Dropout, is proposed to tackle semantic segmentation of aerial and satellite images. Bayesian deep learning can provide both a semantic segmentation and uncertainty maps. Based on the popular U-Net architecture, our model achieves semantic segmentation with high accuracy, e.g. F1-score and overall accuracy respectively reaching 90.84% and 93.22% on a public standard dataset. Uncertainty maps, also derived from our model, show a strong interest in qualitative evaluation of the segmentation and in the improvement of the database.
更多查看译文
关键词
Deep learning,Semantic segmentation,Bayesian network,Optical imagery,Uncertainty estimation
AI 理解论文
溯源树
样例
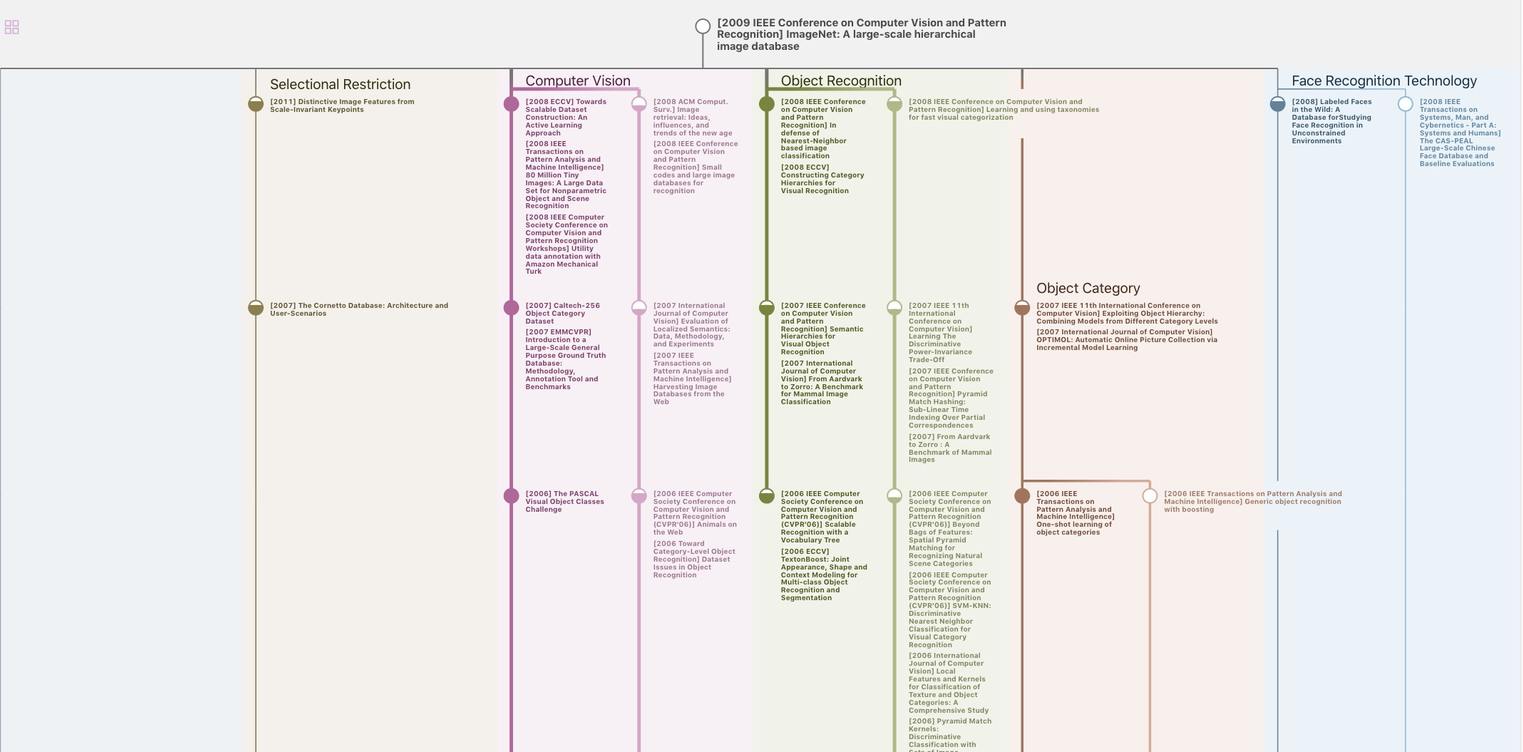
生成溯源树,研究论文发展脉络
Chat Paper
正在生成论文摘要