Deep Neural Network Architecture Search for Emulating Physical Parameterization of Planetary Boundary Layer Height.
IGARSS(2021)
摘要
Multiple satellites and ground based Lidar instruments are becoming increasingly available in providing near daily coverage of aerosol distributions over the US. Aerosols are very small particles, both liquid and solid, arising from both natural and human activities. Atmospheric aerosols directly influence incoming solar radiation by scattering and reflection and indirectly by serving as nuclei for cloud formation of rain, ice and graupel. In addition, aerosols are emitted in the surface layer and pollute the Planetary Boundary Layer (PBL) affecting human health. PBL height (PBLH) determines the vertical extent to which most pollutants effectively disperse and is a key variable in air quality studies. Incorporating aerosol emissions with microphysics and chemistry parameterizations can significantly impact sub-seasonal weather forecasts directly through model radiative transfer parametrization and indirectly can influences the cloud formation, precipitation and the onset of deep convective storms through microphysical processes. Cloud resolving regional forecast models at 0.01 0 (~ 1km) spatial resolution employing such parameterizations will require a fully dedicated petascale computer to effect sub-seasonal forecasts on a weekly basis. In this paper, we initially study the potential of Neural Architecture Searches (NAS) to accurately emulate aerosol and microphysical parameterizations at coarse spatial resolutions to assess potential speed ups over conventional computational approaches. We conduct two experiments, a coarse 1-year WRF model simulation conducted at 2.5° over N. America and 0.8° over Eastern US. with 30 levels including CHEM, Bulk 2-moment microphysics to explore the feasibility of using NAS nets for sub seasonal forecasts and a second short term 6-day high resolution simulation at 0.09°with a single domain over the US and a 0.03° inner domain over eastern US. The problem running the WRF model at cloud resolving resolutions (for example 1km - 4km) is that it will require large computational times and resources. By first studying a coarse WRF experiment, we only need 10 min./sim day on 18 nodes or ~ 14 hrs/sub-seasonal forecast compared with 4hrs /day or approximately 5 dedicated days /sub-seasonal prediction. Our 6 day forecast study is used to provide computing time estimate for testing scalability and takes 20 min./day to run the 0.09° sub-seasonal simulation when using chemical and microphysics components at 3km. We employ the Auto-Keras NAS model, which accurately emulates the 1 year coarse experiment as well as gains an expected 10x speedup. This NAS deep neural net model will be used to infer the aerosol types, sizes and distributions to enable microphysics inferences and realistic mixed boundary layer heights with expected speedups of an order of magnitude or more.
更多查看译文
关键词
NAS,PBLH,WRF,Machine Learning
AI 理解论文
溯源树
样例
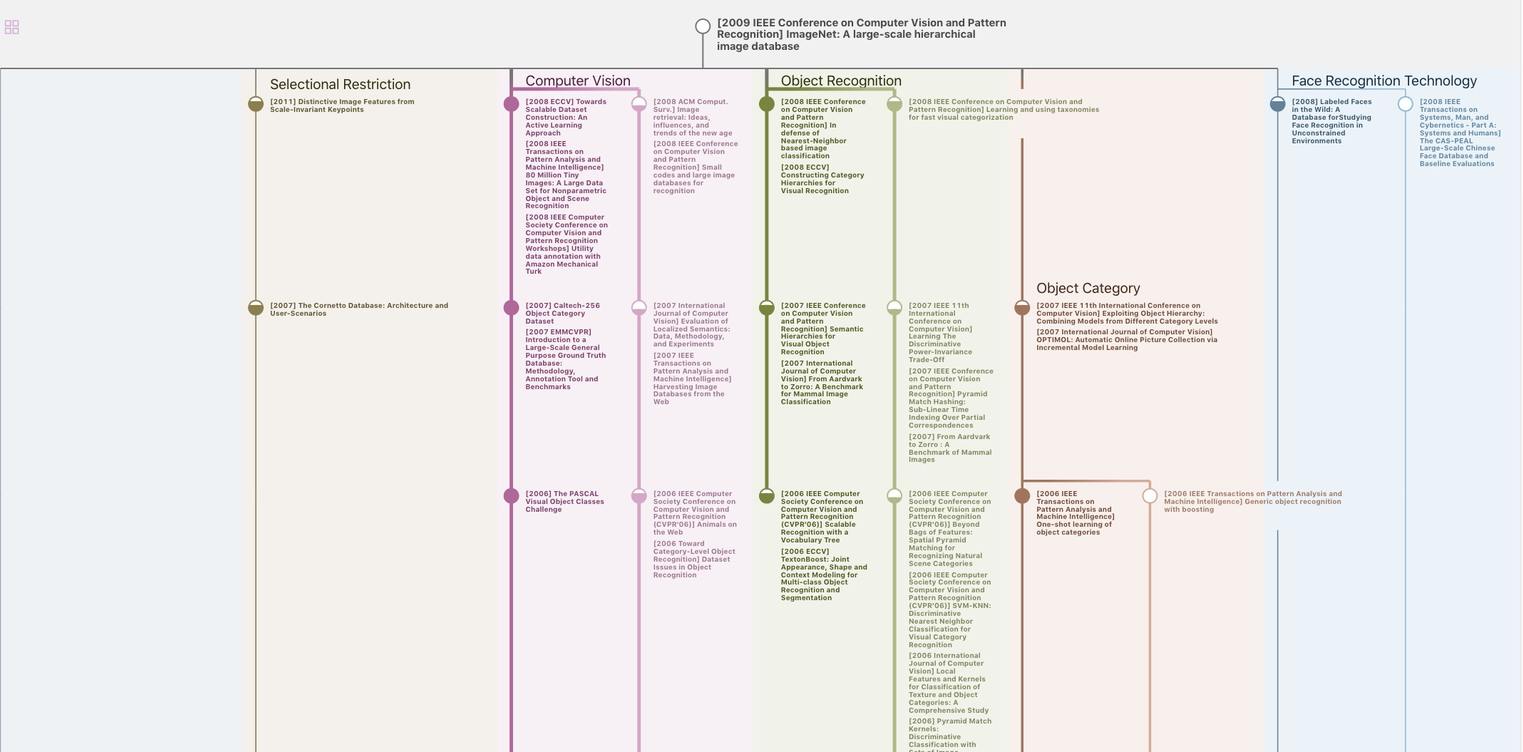
生成溯源树,研究论文发展脉络
Chat Paper
正在生成论文摘要