Spectral Unmixing Using Autoencoder with Spatial and Spectral Regularizations.
IGARSS(2021)
摘要
In this paper, we propose a novel approach for spectral unmixing based on unsupervised learning using autoencoder with Inhomogeneous Gaussian Markov random field (IGMRF) as prior for regularization. The decoder part of our autoencoder has linear weights making it a linear mixture model (LMM). The weights represent the endmember matrix that makes the hidden unit of autoencoder as abundances. IGMRF is used to apply spatial regularization on abundances that also preserves the discontinuities. To incorporate the spectral regularization, we use IGMRF priors on endmembers. In addition, we also apply the spatial and spectral regularizations on the given hyperspectral images (HSI). IGMRF parameters at every pixel locations are calculated using initial estimates of endmembers and abundances. We obtain both endmembers and their abundances by optimizing the loss function that consists of a data term and IGMRF prior terms. Experiments are performed on different noise level synthetic data and two real data (Jasper ridge and Urban). The results of the proposed approach are better when compared to existing state-of-the-art approaches.
更多查看译文
关键词
Autoencoder,IGMRF,Spectral unmixing
AI 理解论文
溯源树
样例
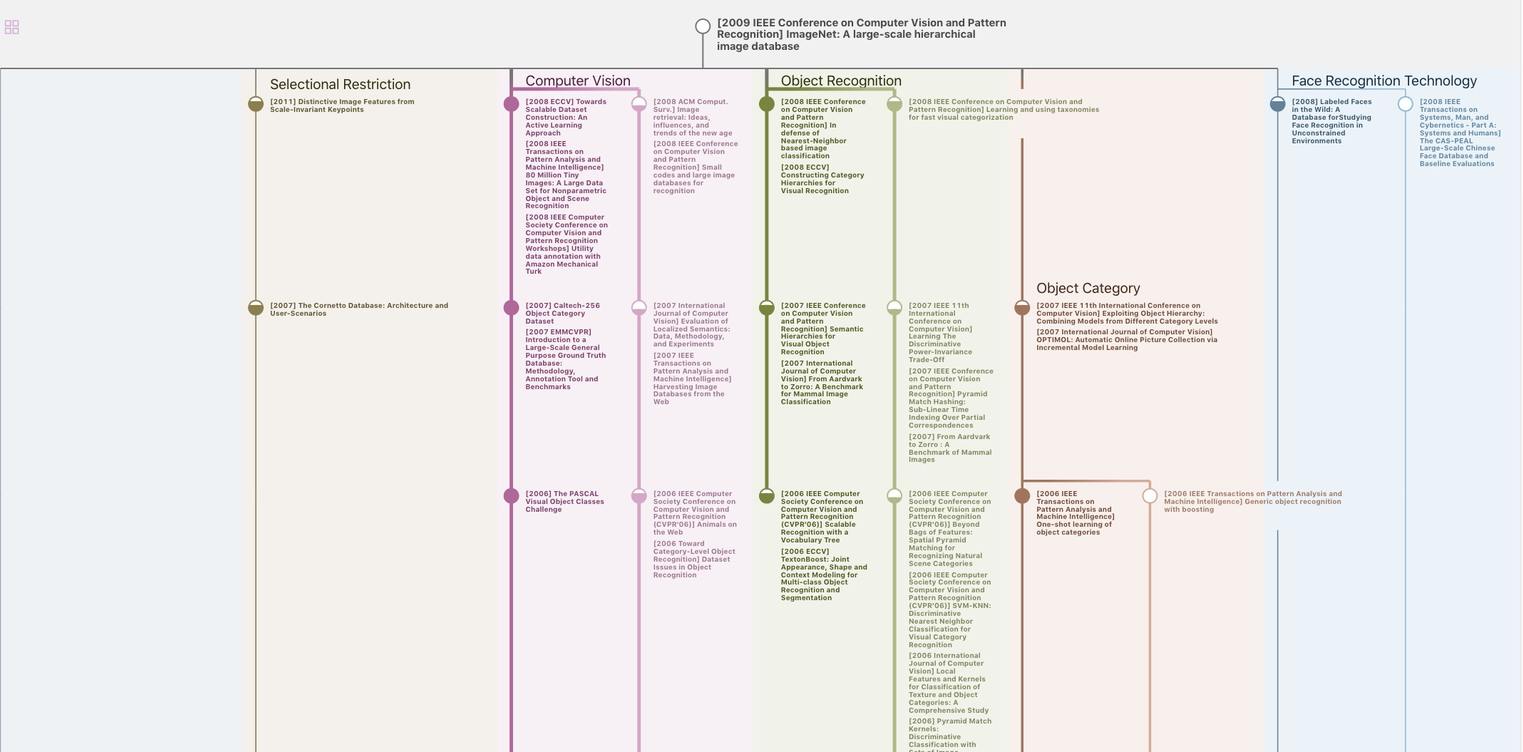
生成溯源树,研究论文发展脉络
Chat Paper
正在生成论文摘要