A Sure-Based Unsupervised Deep Learning Method for Sar Despeckling
IGARSS(2021)
摘要
Synthetic Aperture Radar (SAR) images most often get corrupted by multiplicative (granular) signal variations known as ‘speckle’ during the remote acquisition. In this paper, we propose an unsupervised deep learning approach for SAR despeckling without the need for clean SAR references. To this end, we employ Stein's unbiased risk estimator (SURE) that facilitates estimation of the true mean-squared error between the output of the de-speckling network and the ground truth, however, using only available SAR images. We utilize thus unsupervised trained U-Net architecture, coupled with the proposed SURE-based loss function. Experiments are conducted on synthetic as well as real Sentinel-l SAR images. The results are compared both qualitatively and quantitatively with popular methods in SAR despeckling.
更多查看译文
关键词
Deep learning,Despeckling,SURE,SAR,Unsupervised learning
AI 理解论文
溯源树
样例
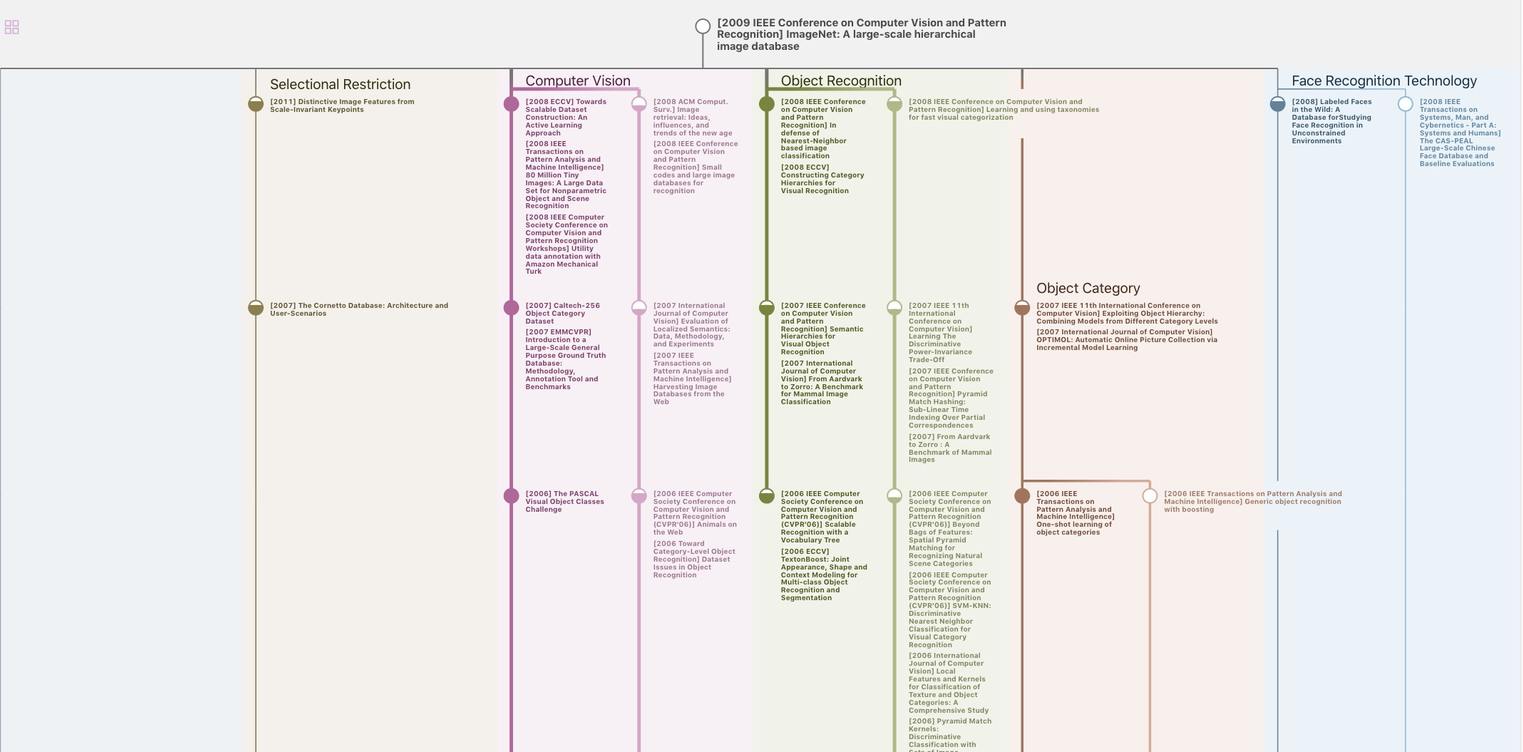
生成溯源树,研究论文发展脉络
Chat Paper
正在生成论文摘要