Robust lEarned Shrinkage-Thresholding (REST): Robust unrolling for sparse recover
arxiv(2021)
摘要
In this paper, we consider deep neural networks for solving inverse problems that are robust to forward model mis-specifications. Specifically, we treat sensing problems with model mismatch where one wishes to recover a sparse high-dimensional vector from low-dimensional observations subject to uncertainty in the measurement operator. We then design a new robust deep neural network architecture by applying algorithm unfolding techniques to a robust version of the underlying recovery problem. Our proposed network - named Robust lEarned Shrinkage-Thresholding (REST) - exhibits an additional normalization processing compared to Learned Iterative Shrinkage-Thresholding Algorithm (LISTA), leading to reliable recovery of the signal under sample-wise varying model mismatch. The proposed REST network is shown to outperform state-of-the-art model-based and data-driven algorithms in both compressive sensing and radar imaging problems wherein model mismatch is taken into consideration.
更多查看译文
关键词
sparse,shrinkage-thresholding
AI 理解论文
溯源树
样例
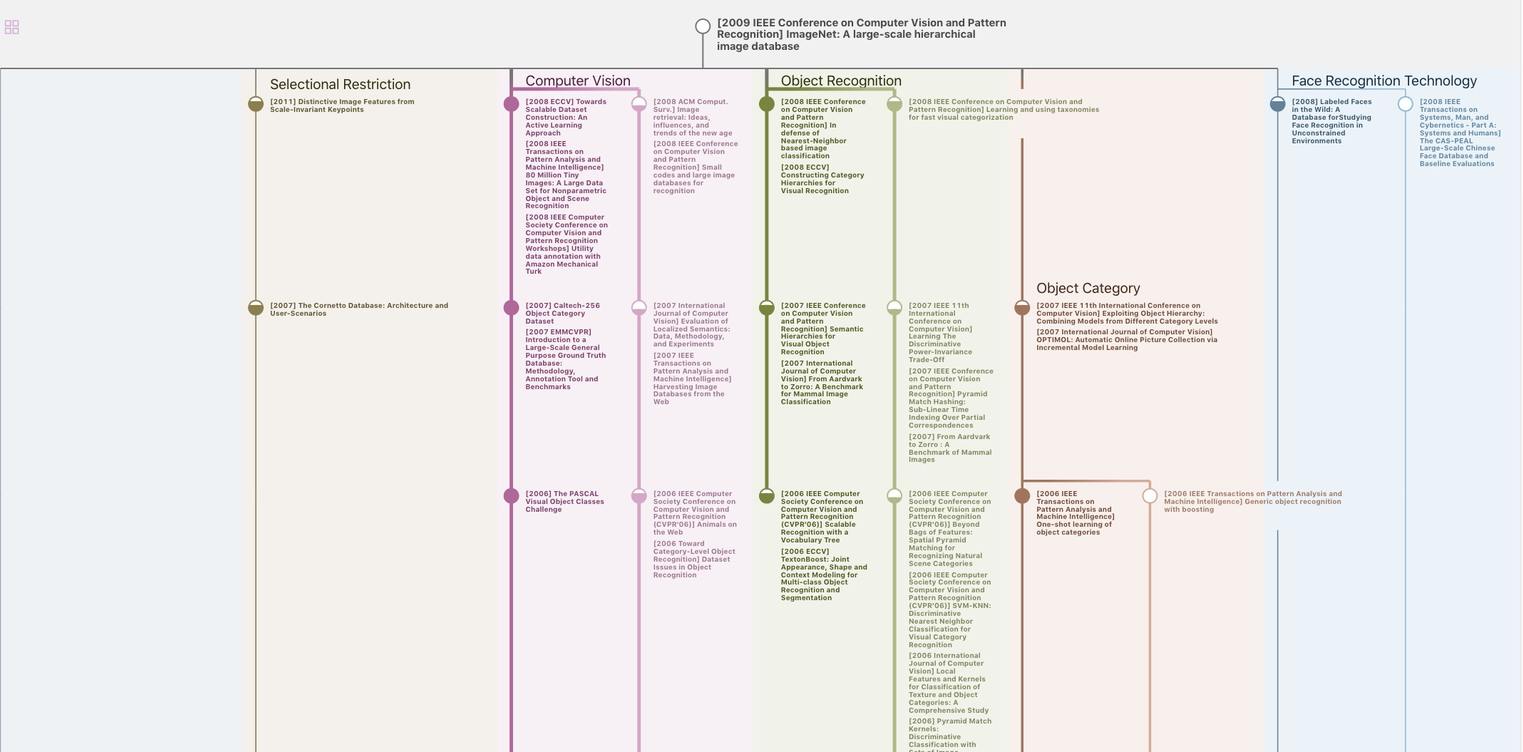
生成溯源树,研究论文发展脉络
Chat Paper
正在生成论文摘要