Convolutional neural networks for shower energy prediction in liquid argon time projection chambers
JOURNAL OF INSTRUMENTATION(2022)
摘要
When electrons with energies of O(100) MeV pass through a liquid argon time projection chamber (LArTPC), they deposit energy in the form of electromagnetic showers. Methods to reconstruct the energy of these showers in LArTPCs often rely on the combination of a clustering algorithm and a linear calibration between the shower energy and charge contained in the cluster. This reconstruction process could be improved through the use of a convolutional neural network (CNN). Here we discuss the performance of various CNN-based models on simulated LArTPC images, and then compare the best performing models to a typical linear calibration algorithm. We show that the CNN method is able to address inefficiencies caused by unresponsive wires in LArTPCs and reconstruct a larger fraction of imperfect events to within 5 % accuracy compared with the linear algorithm.
更多查看译文
关键词
Pattern recognition, cluster finding, calibration and fitting methods, Noble liquid detectors (scintillation, ionization, double-phase), Time projection Chambers (TPC)
AI 理解论文
溯源树
样例
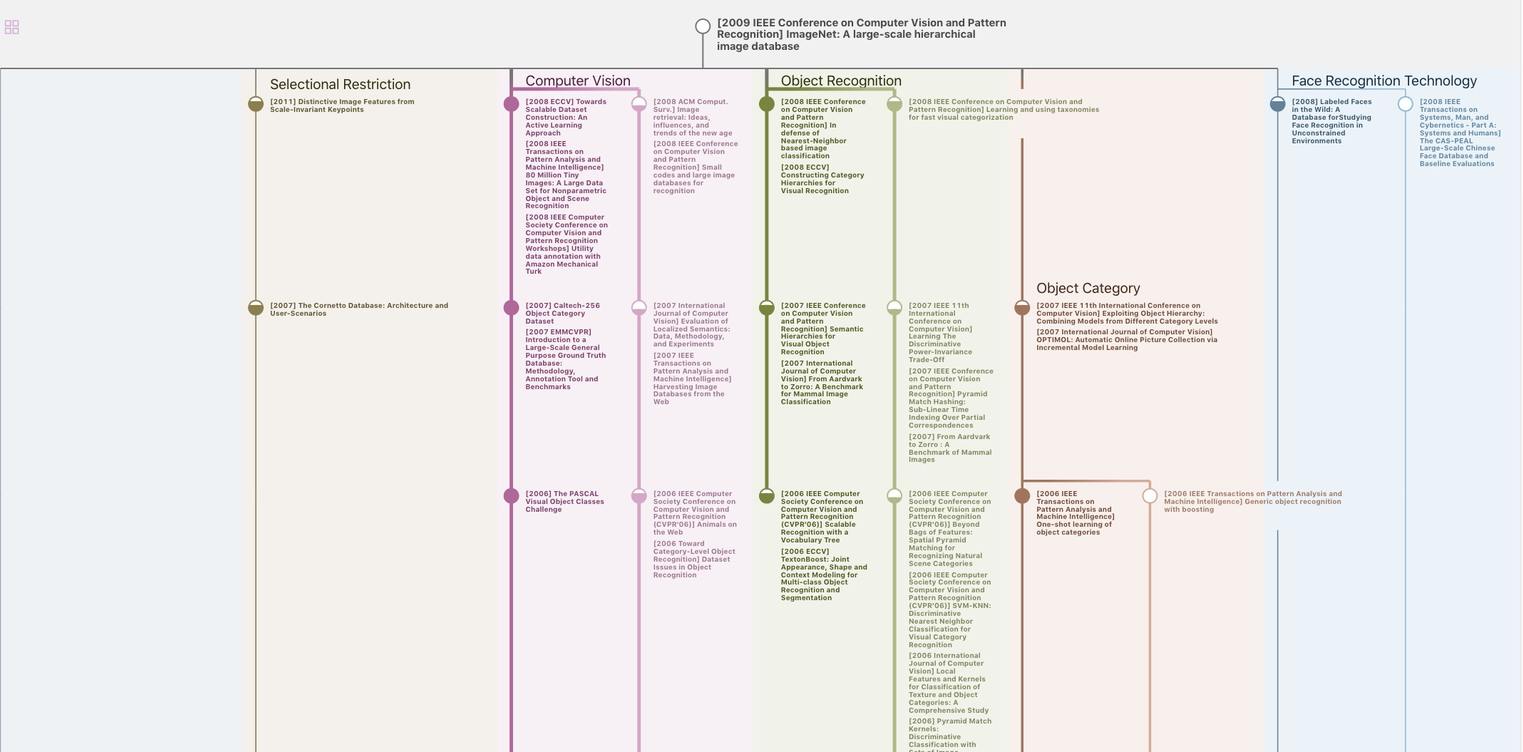
生成溯源树,研究论文发展脉络
Chat Paper
正在生成论文摘要