A Real-Time Energy and Cost Efficient Vehicle Route Assignment Neural Recommender System
arXivorg(2021)
摘要
This paper presents a neural network recommender system algorithm for assigning vehicles to routes based on energy and cost criteria. In this work, we applied this new approach to efficiently identify the most cost-effective medium and heavy duty truck (MDHDT) powertrain technology, from a total cost of ownership (TCO) perspective, for given trips. We employ a machine learning based approach to efficiently estimate the energy consumption of various candidate vehicles over given routes, defined as sequences of links (road segments), with little information known about internal dynamics, i.e using high level macroscopic route information. A complete recommendation logic is then developed to allow for real-time optimum assignment for each route, subject to the operational constraints of the fleet. We show how this framework can be used to (1) efficiently provide a single trip recommendation with a top-k vehicles star ranking system, and (2) engage in more general assignment problems where n vehicles need to be deployed over m ≤ n trips. This new assignment system has been deployed and integrated into the POLARIS Transportation System Simulation Tool for use in research conducted by the Department of Energy's Systems and Modeling for Accelerated Research in Transportation (SMART) Mobility Consortium
更多查看译文
AI 理解论文
溯源树
样例
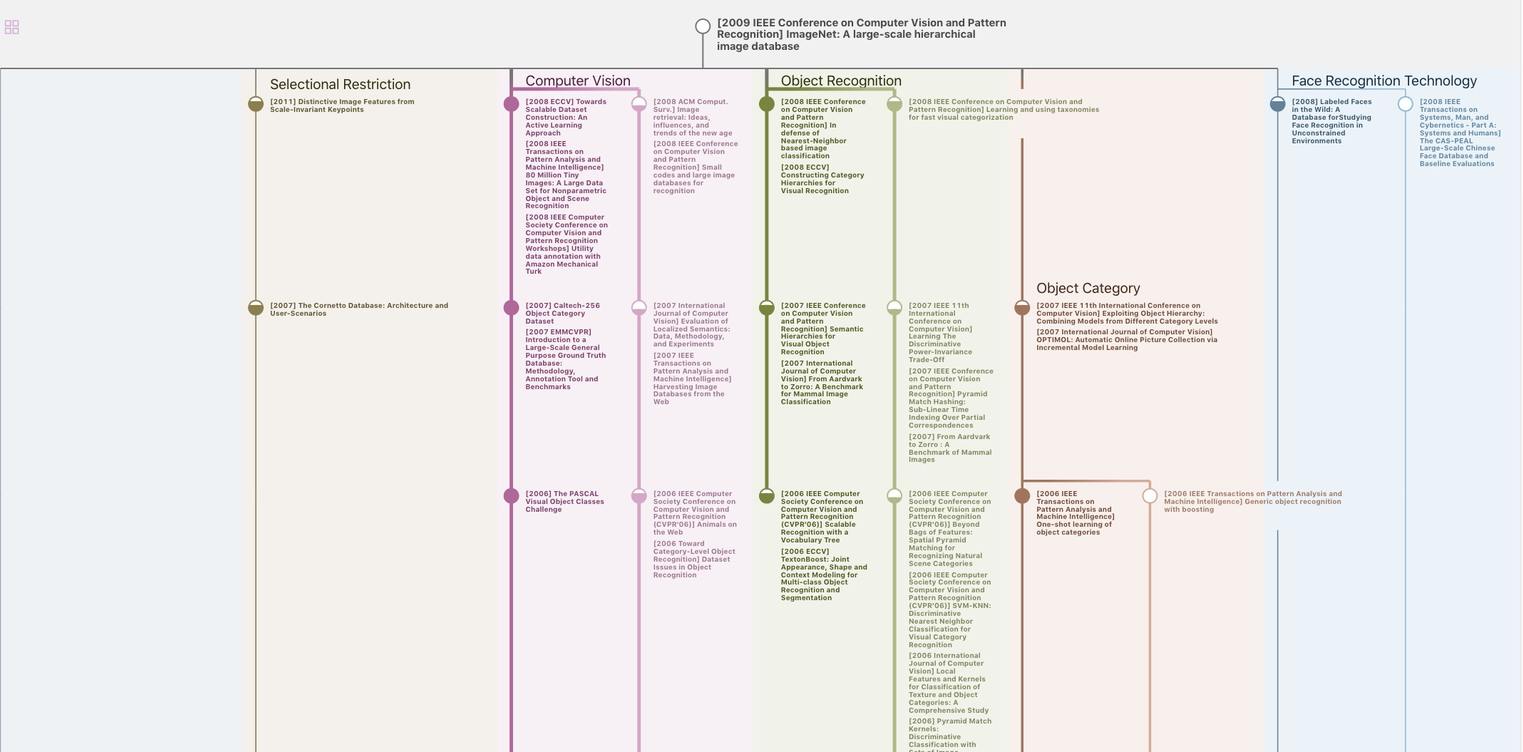
生成溯源树,研究论文发展脉络
Chat Paper
正在生成论文摘要