Fully automated grading system for the evaluation of punctate epithelial erosions using deep neural networks
The British journal of ophthalmology(2023)
摘要
Purpose The goal was to develop a fully automated grading system for the evaluation of punctate epithelial erosions (PEEs) using deep neural networks. Methods A fully automated system was developed to detect corneal position and grade staining severity given a corneal fluorescein staining image. The fully automated pipeline consists of the following three steps: a corneal segmentation model extracts corneal area; five image patches are cropped from the staining image based on the five subregions of extracted cornea; a staining grading model predicts a score for each image patch from 0 to 3, and automated grading score for the whole cornea is obtained from 0 to 15. Finally, the clinical grading scores annotated by three ophthalmologists were compared with automated grading scores. Results For corneal segmentation, the segmentation model achieved an intersection over union of 0.937. For punctate staining grading, the grading model achieved a classification accuracy of 76.5% and an area under the receiver operating characteristic curve of 0.940 (95% CI 0.932 to 0.949). For the fully automated pipeline, Pearson's correlation coefficient between the clinical and automated grading scores was 0.908 (p<0.01). Bland-Altman analysis revealed 95% limits of agreement between the clinical and automated grading scores of between -4.125 and 3.720 (concordance correlation coefficient=0.904). The average time required for processing a single stained image during pipeline was 0.58 s. Conclusion A fully automated grading system was developed to evaluate PEEs. The grading results may serve as a reference for ophthalmologists in clinical trials and residency training procedures.
更多查看译文
关键词
cornea,imaging
AI 理解论文
溯源树
样例
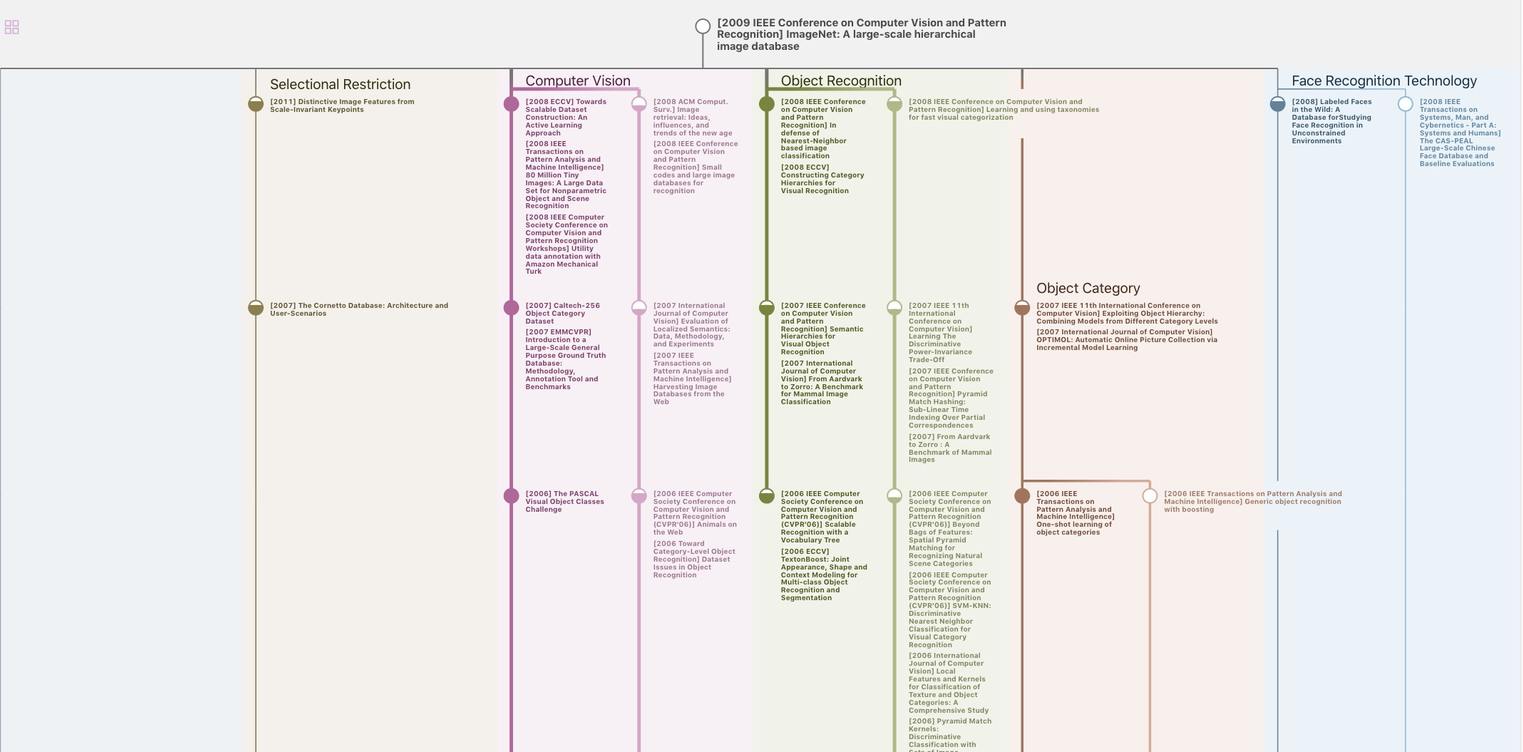
生成溯源树,研究论文发展脉络
Chat Paper
正在生成论文摘要