Distilling Bit-level Sparsity Parallelism for General Purpose Deep Learning Acceleration
MICRO(2021)
摘要
ABSTRACT Along with the rapid evolution of deep neural networks, the ever-increasing complexity imposes formidable computation intensity to the hardware accelerator. In this paper, we propose a novel computing philosophy called “bit interleaving” and the associate accelerator design called “Bitlet” to maximally exploit the bit-level sparsity. Apart from existing bit-serial/parallel accelerators, Bitlet leverages the abundant “sparsity parallelism” in the parameters to enforce the inference acceleration. Bitlet is versatile by supporting diverse precisions on a single platform, including floating-point 32 and fixed-point from 1b to 24b. The versatility enables Bitlet feasible for both efficient inference and training. Empirical studies on 12 domain-specific deep learning applications highlight the following results: (1) up to 81 × /21 × energy efficiency improvement for training/inference over recent high performance GPUs; (2) up to 15 × /8 × higher speedup/efficiency over state-of-the-art fixed-point accelerators; (3) 1.5mm2 area and scalable power consumption from 570mW (float32) to 432mW (16b) and 365mW (8b) @28nm TSMC; (4) highly configurable justified by ablation and sensitivity studies.
更多查看译文
AI 理解论文
溯源树
样例
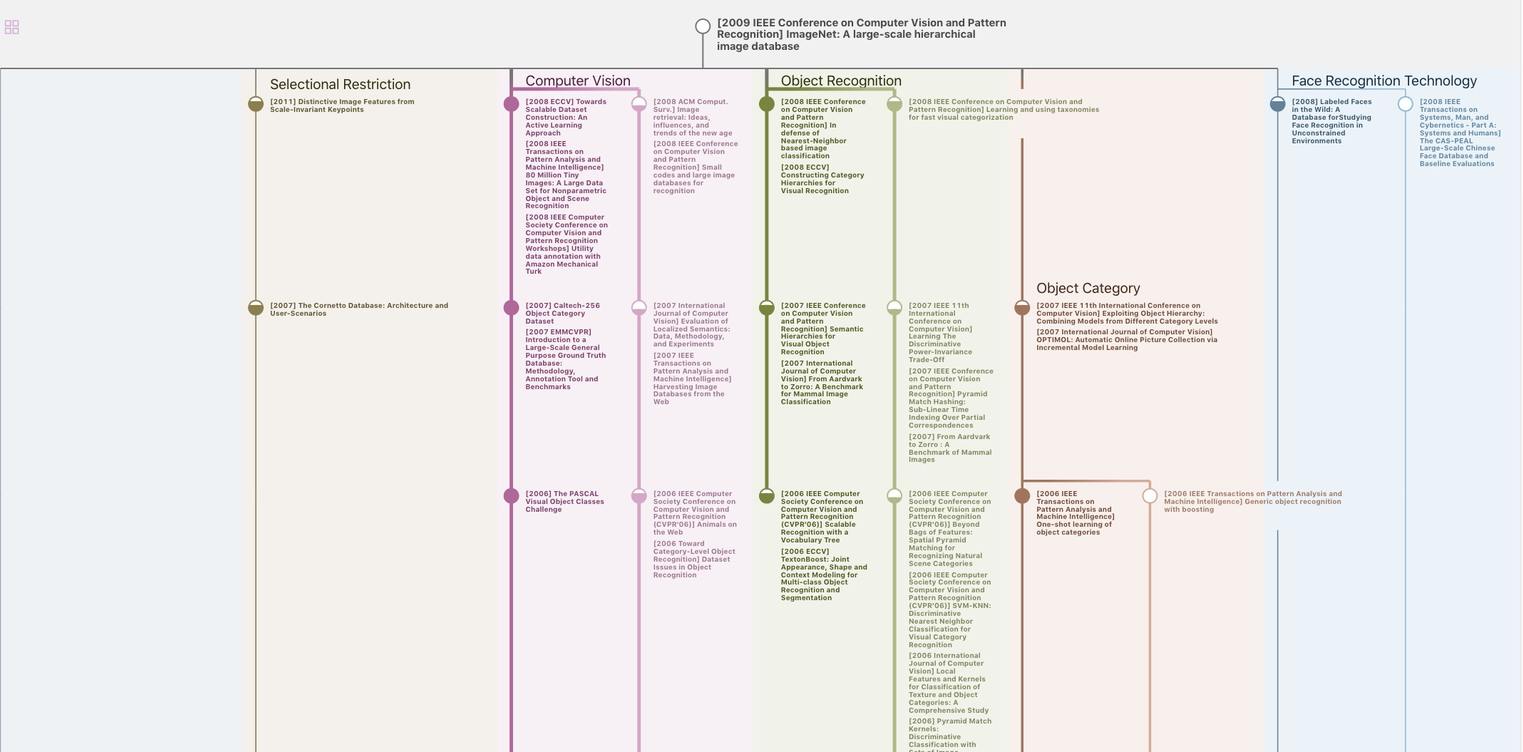
生成溯源树,研究论文发展脉络
Chat Paper
正在生成论文摘要