Few-shot Learning for Multi-Modality Tasks
International Multimedia Conference(2021)
摘要
ABSTRACTRecent deep learning methods rely on a large amount of labeled data to achieve high performance. These methods may be impractical in some scenarios, where manual data annotation is costly or the samples of certain categories are scarce (e.g., tumor lesions, endangered animals and rare individual activities). When only limited annotated samples are available, these methods usually suffer from the overfitting problem severely, which degrades the performance significantly. In contrast, humans can recognize the objects in the images rapidly and correctly with their prior knowledge after exposed to only a few annotated samples. To simulate the learning schema of humans and relieve the reliance on the large-scale annotation benchmarks, researchers start shifting towards the few-shot learning problem: they try to learn a model to correctly recognize novel categories with only a few annotated samples.
更多查看译文
AI 理解论文
溯源树
样例
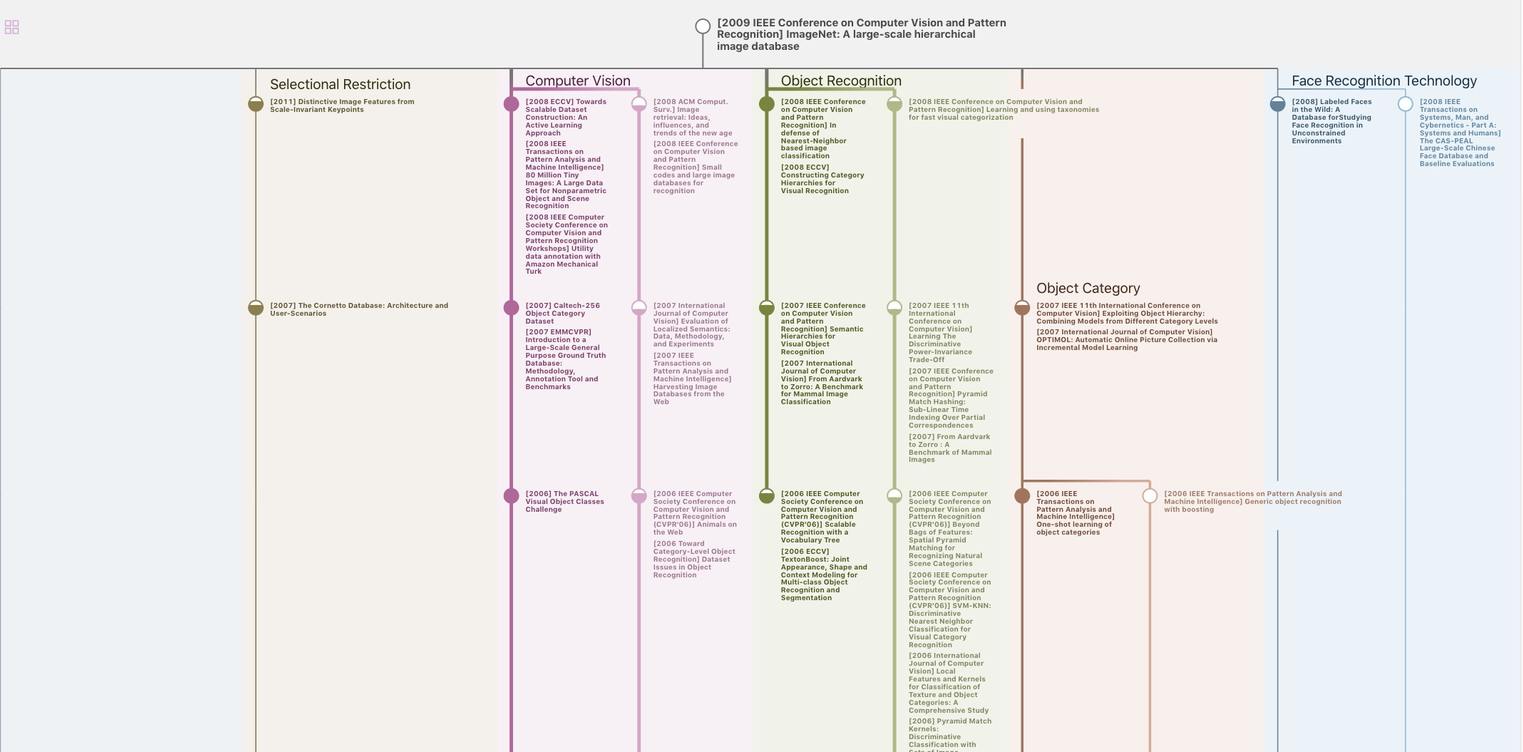
生成溯源树,研究论文发展脉络
Chat Paper
正在生成论文摘要