Annotation-Efficient Semantic Segmentation with Shape Prior Knowledge
International Multimedia Conference(2021)
摘要
ABSTRACTDeep learning methods have achieved great success on semantic segmentation in recent years. But the training typically relies on large-scale fully-annotated ground truth masks, which are difficult to obtain in practice. In this research, we study the problem of reducing the annotation cost of segmentation network training with a focus on exploring the shape prior knowledge of objects. Under the context of three applications, we study three types of shape priors. Specifically, we first exploit the implicit shape prior of curve structures to propose a weakly supervised curve structure segmentation method, and then explicitly formulate the shape prior of anatomical structures as loss functions to propose a one-shot anatomical structures segmentation network. Last, we try to generalize the shape constraint to arbitrary objects to propose a class-agnostic few-shot segmentation framework. Experiment results show that our methods could achieve comparable or better performance than fully supervised segmentation methods with less annotation costs on the studied applications.
更多查看译文
AI 理解论文
溯源树
样例
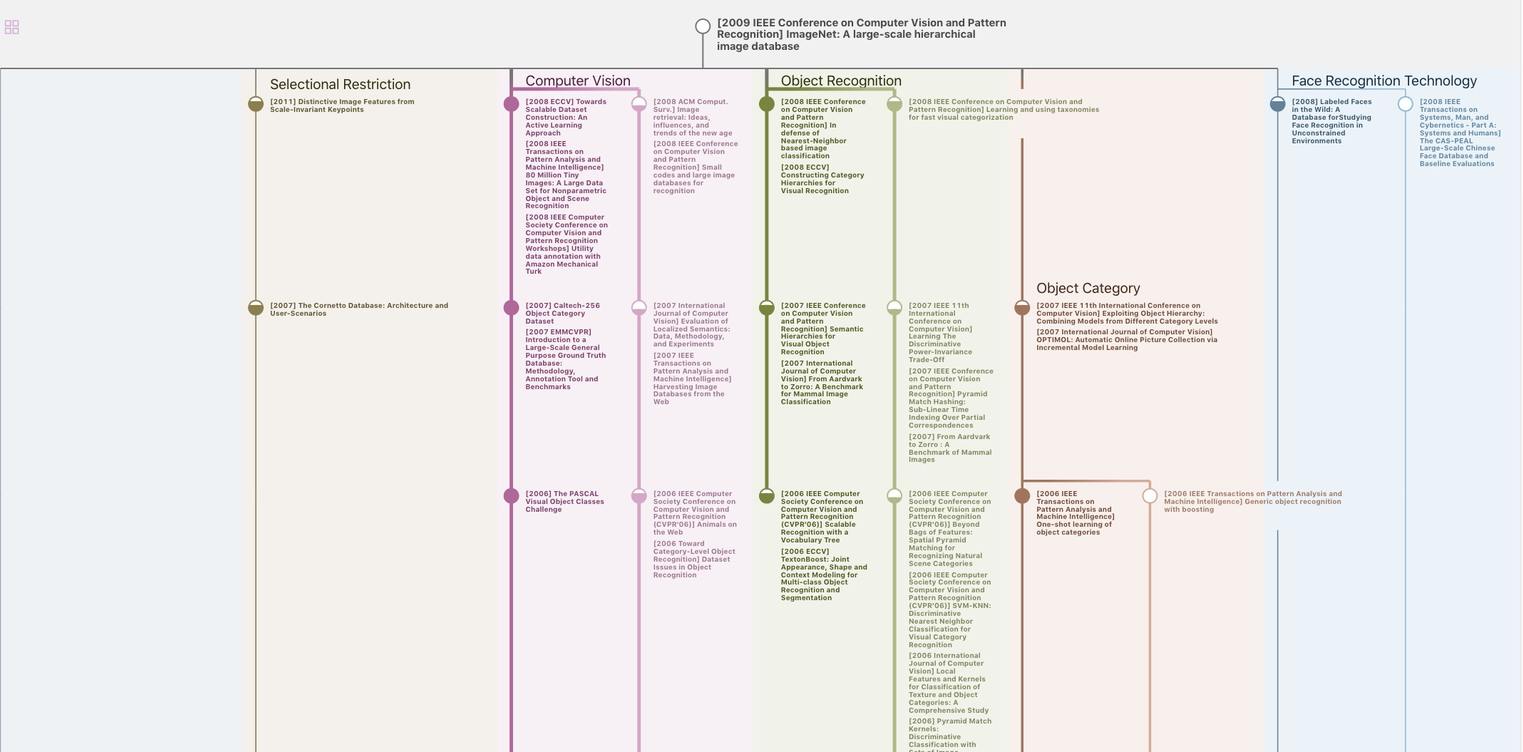
生成溯源树,研究论文发展脉络
Chat Paper
正在生成论文摘要