Interpreting Super-Resolution CNNs for Sub-Pixel Motion Compensation in Video Coding
International Multimedia Conference(2021)
摘要
ABSTRACTMachine learning approaches for more efficient video compression have been developed thanks to breakthroughs in deep learning. However, they typically bring coding improvements at the cost of significant increases in computational complexity, making them largely unsuitable for practical applications. In this paper, we present open-source software for convolutional neural network-based solutions which improve the interpolation of reference samples needed for fractional precision motion compensation. Contrary to previous efforts, the networks are fully linear, allowing them to be interpreted, with a full interpolation filter set derived from trained models, making it simple to integrate in conventional video coding schemes. When implemented in the context of the state-of-the-art Versatile Video Coding (VVC) test model, the complexity of the learned interpolation schemes is significantly reduced compared to the interpolation with full neural networks, while achieving notable coding efficiency improvements on lower resolution video sequences. The open-source software package is available at https://github.com/bbc/cnn-fractional-motion-compensation under the 3-clause BSD license.
更多查看译文
AI 理解论文
溯源树
样例
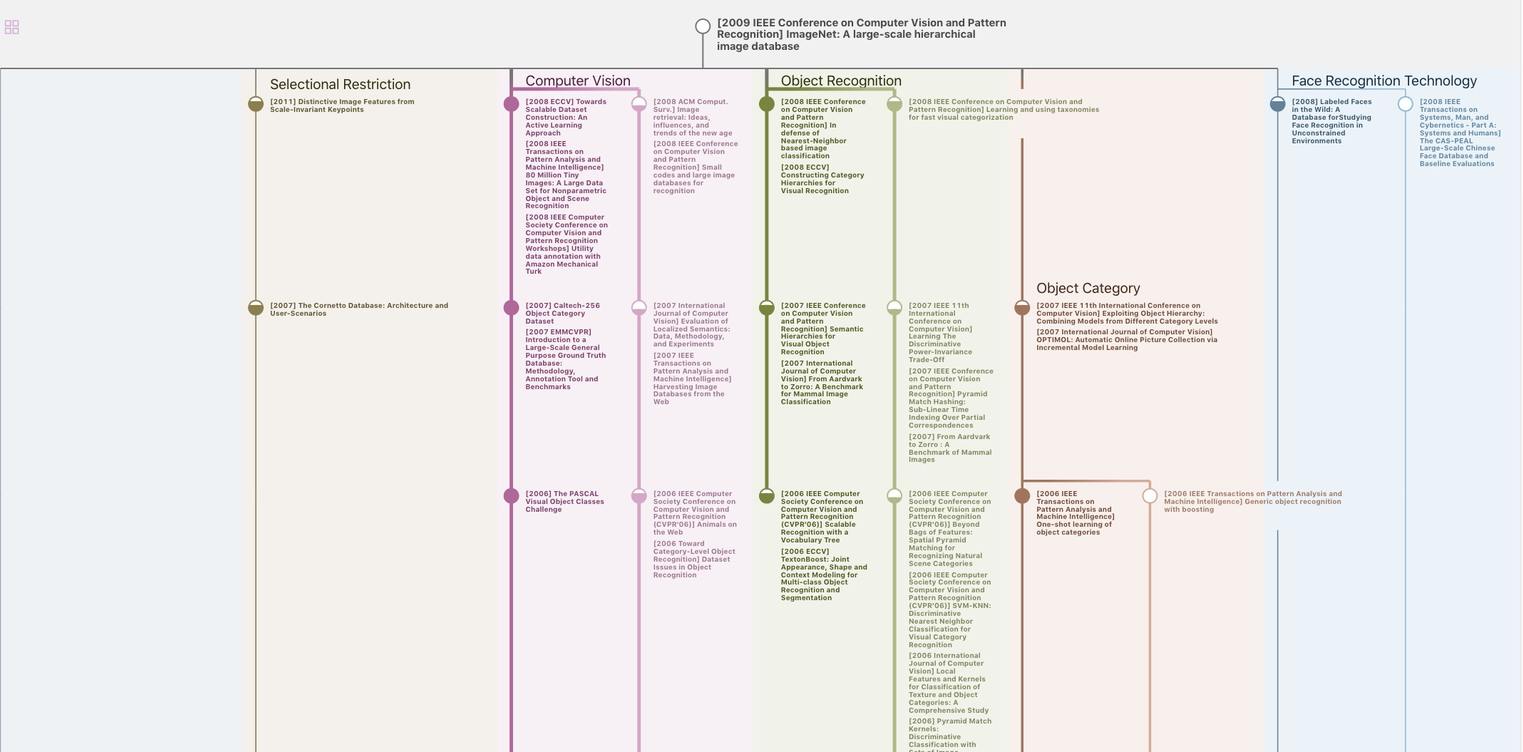
生成溯源树,研究论文发展脉络
Chat Paper
正在生成论文摘要