Semantic-aware Transfer with Instance-adaptive Parsing for Crowded Scenes Pose Estimation
International Multimedia Conference(2021)
摘要
ABSTRACTCrowded scenes human pose estimation remains challenging, which requires joint comprehension of multi-persons and their keypoints in a highly complex scenario. The top-down mechanism, which is a detect-then-estimate pipeline, has become the mainstream solution for general pose estimation and obtained impressive progress. However, simply applying this mechanism to crowded scenes pose estimation results in unsatisfactory performance due to several issues, in particular involving missing keypoints in crowds and ambiguously labeling during training. To tackle above two issues, we introduce a novel method named Semantic-aware Transfer with Instance-adaptive Parsing (STIP). Specifically, our STIP first enhances the discriminative power of pixel-level representations with a semantic-aware mechanism, where it smartly decides which pixels to enhance and what semantic embeddings to add. In this way, the missing keypoints detection can be alleviated.Secondly, instead of adopting a standard regressor with fixed parameters, we propose a new instance-adaptive parsing method, where it dynamically generates instance-specific parameters for reducing adverse effects caused by ambiguously labeling. Notably, STIP is designed in a plugin fashion and it can be integrated into any top-down models, such as HRNet. Extensive experiments on two challenging benchmarks, i.e., CrowdPose and MS-COCO, demonstrate the superiority and generalizability of our approach.
更多查看译文
AI 理解论文
溯源树
样例
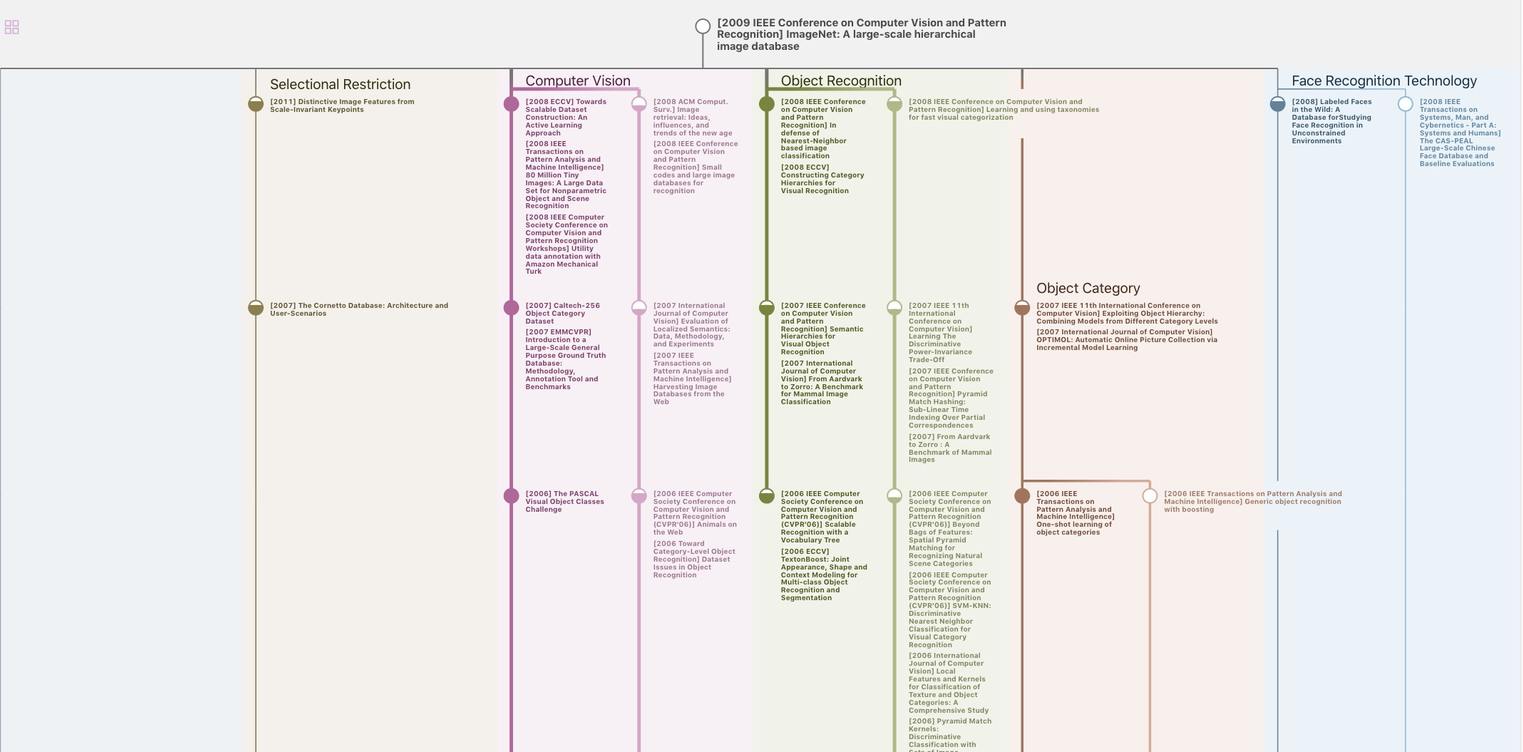
生成溯源树,研究论文发展脉络
Chat Paper
正在生成论文摘要