FOCAS: Practical Video Super Resolution using Foveated Rendering
PROCEEDINGS OF THE 29TH ACM INTERNATIONAL CONFERENCE ON MULTIMEDIA, MM 2021(2021)
摘要
Super-resolution (SR) is a well-studied technique for reconstructing high-resolu- tion (HR) images from low-resolution (LR) ones. SR holds great promise for video streaming since an LR video segment can be transmitted from the video server to the client that then reconstructs the HR version using SR, resulting in a significant reduction in network bandwidth. However, SR is seldom used in practice for real-time video streaming, because the computational overhead of frame reconstruction results in large latency and low frame rate. To reduce the computational overhead and make SR practical, we propose a deep-learning-based SR method called Foveated Cascaded Video Super Resolution (FOCAS). FOCAS relies on the fact that human eyes only have high acuity in a tiny central foveal region of the retina. FOCAS uses more neural network blocks in the foveal region to provide higher video quality, while using fewer blocks in the periphery as lower quality is sufficient. To optimize the computational resources and reduce reconstruction latency, FOCAS formulates and solves a convex optimization problem to decide the number of neural network blocks to use in each region of theframe. Using extensive experiments, we show that FOCAS reduces the latency by 50% - 70% while maintaining comparable visual quality as traditional (non-foveated) SR. Further, FOCAS provides a 12 - 16x reduction in the client-to-server network bandwidth in comparison with sending the full HR video segments.
更多查看译文
关键词
super resolution,foveated rendering,deep learning,latency
AI 理解论文
溯源树
样例
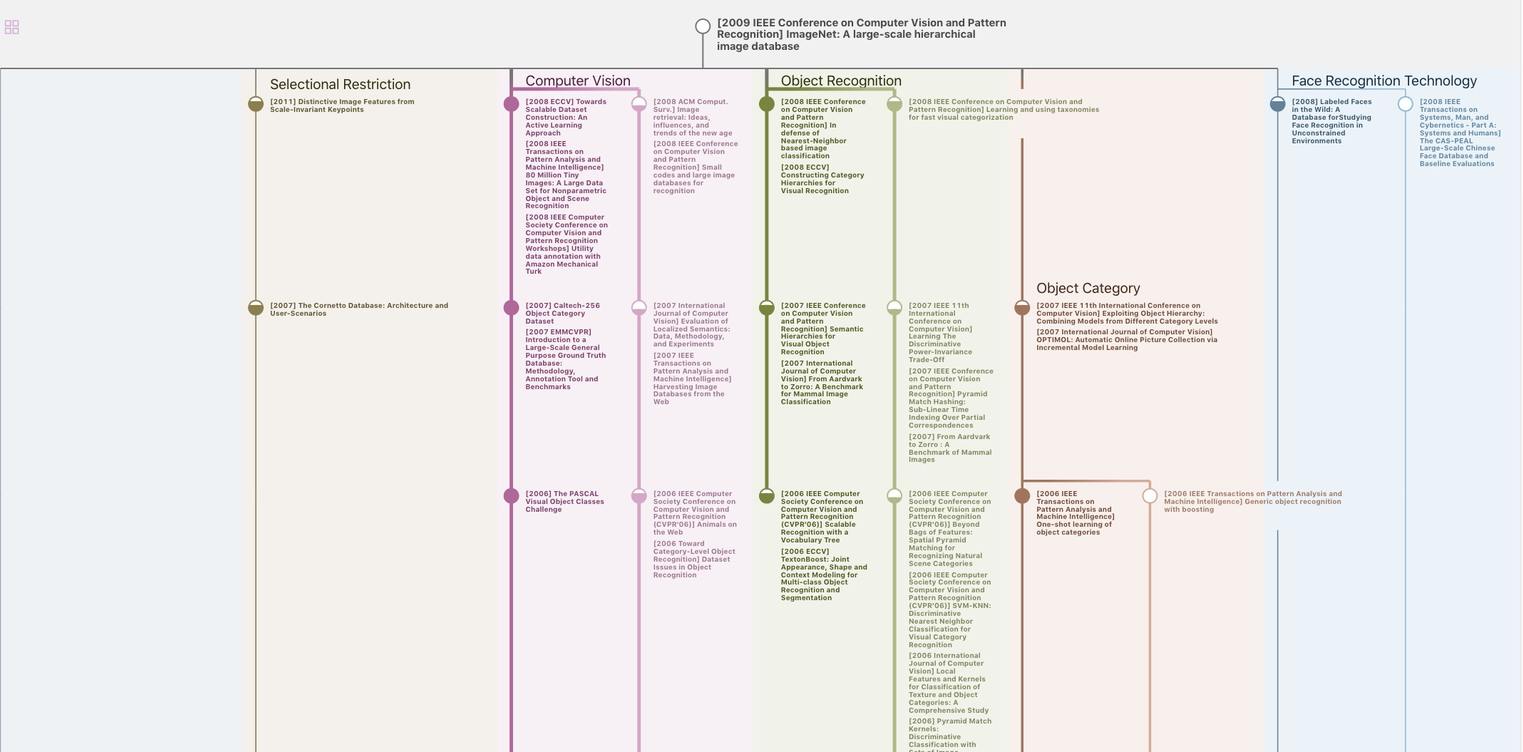
生成溯源树,研究论文发展脉络
Chat Paper
正在生成论文摘要