Recycling Discriminator: Towards Opinion-Unaware Image Quality Assessment Using Wasserstein GAN
International Multimedia Conference(2021)
摘要
ABSTRACTGenerative adversarial networks (GANs) have been extensively used for training networks that perform image generation. After training, the discriminator in GAN was not used anymore. We propose to recycle the trained discriminator for another use: no-reference image quality assessment (NR-IQA). We are motivated by twofold facts. First, in Wasserstein GAN (WGAN), the discriminator is designed to calculate the distance between the distribution of generated images and that of real images; thus, the trained discriminator may encode the distribution of real-world images. Second, NR-IQA often needs to leverage the distribution of real-world images for assessing image quality. We then conjecture that using the trained discriminator for NR-IQA may help get rid of any human-labeled quality opinion scores and lead to a new opinion-unaware (OU) method. To validate our conjecture, we start from a restricted NR-IQA problem, that is IQA for artificially super-resolved images. We train super-resolution (SR) WGAN with two kinds of discriminators: one is to directly evaluate the entire image, and the other is to work on small patches. For the latter kind, we obtain patch-wise quality scores, and then have the flexibility to fuse the scores, e.g., by weighted average. Moreover, we directly extend the trained discriminators for authentically distorted images that have different kinds of distortions. Our experimental results demonstrate that the proposed method is comparable to the state-of-the-art OU NR-IQA methods on SR images and is even better than them on authentically distorted images. Our method provides a better interpretable approach to NR-IQA. Our code and models are available at https://github.com/YunanZhu/RecycleD.
更多查看译文
AI 理解论文
溯源树
样例
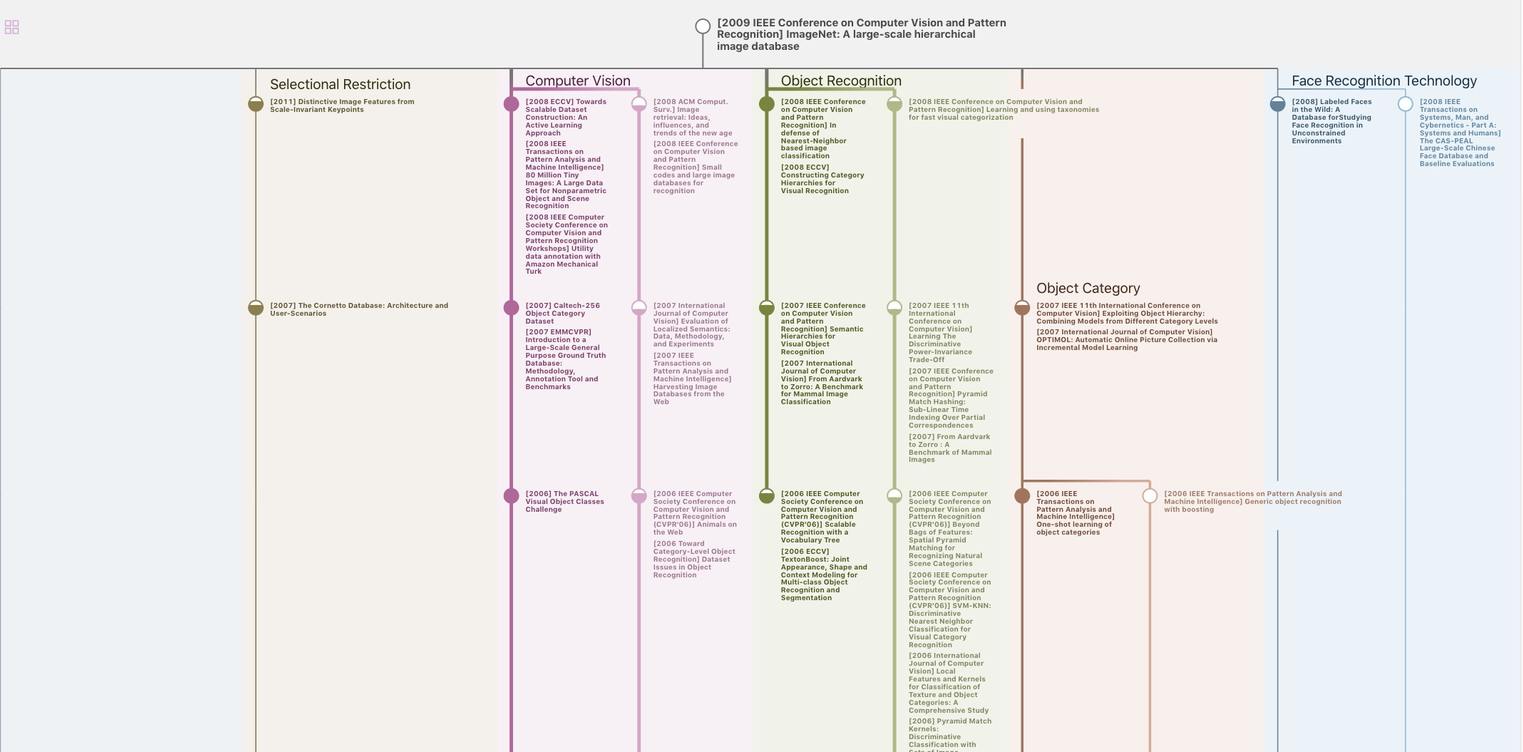
生成溯源树,研究论文发展脉络
Chat Paper
正在生成论文摘要