Learning Text-image Joint Embedding for Efficient Cross-modal Retrieval with Deep Feature Engineering
ACM Transactions on Information Systems(2022)
摘要
AbstractThis article introduces a two-phase deep feature engineering framework for efficient learning of semantics enhanced joint embedding, which clearly separates the deep feature engineering in data preprocessing from training the text-image joint embedding model. We use the Recipe1M dataset for the technical description and empirical validation. In preprocessing, we perform deep feature engineering by combining deep feature engineering with semantic context features derived from raw text-image input data. We leverage LSTM to identify key terms, deep NLP models from the BERT family, TextRank, or TF-IDF to produce ranking scores for key terms before generating the vector representation for each key term by using Word2vec. We leverage Wide ResNet50 and Word2vec to extract and encode the image category semantics of food images to help semantic alignment of the learned recipe and image embeddings in the joint latent space. In joint embedding learning, we perform deep feature engineering by optimizing the batch-hard triplet loss function with soft-margin and double negative sampling, taking into account also the category-based alignment loss and discriminator-based alignment loss. Extensive experiments demonstrate that our SEJE approach with deep feature engineering significantly outperforms the state-of-the-art approaches.
更多查看译文
关键词
Cross-modal retrieval, deep feature engineering, multi-modal learning
AI 理解论文
溯源树
样例
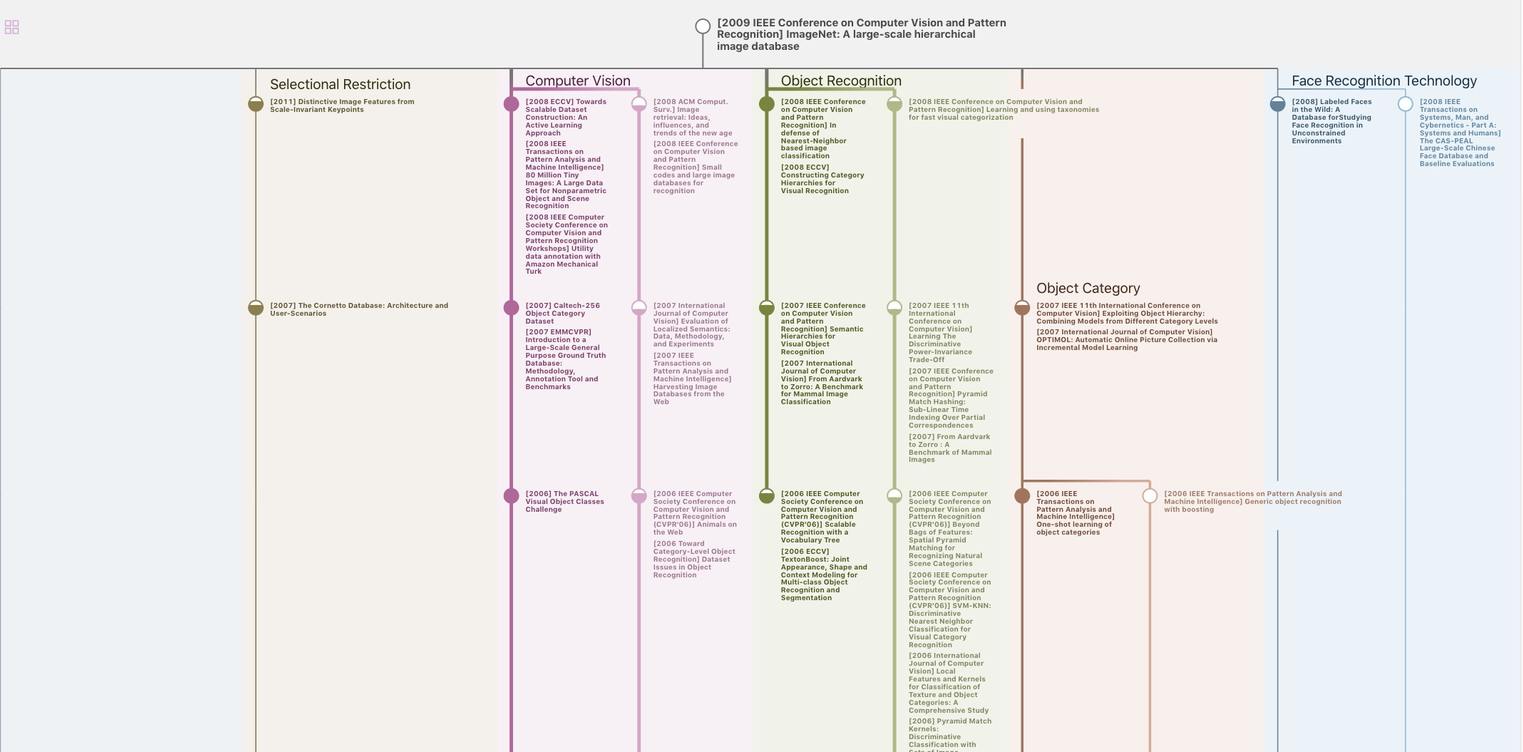
生成溯源树,研究论文发展脉络
Chat Paper
正在生成论文摘要