Rethinking Generalization Performance of Surgical Phase Recognition with Expert-Generated Annotations
arxiv(2021)
摘要
As the area of application of deep neural networks expands to areas requiring expertise, e.g., in medicine and law, more exquisite annotation processes for expert knowledge training are required. In particular, it is difficult to guarantee generalization performance in the clinical field in the case of expert knowledge training where opinions may differ even among experts on annotations. To raise the issue of the annotation generation process for expertise training of CNNs, we verified the annotations for surgical phase recognition of laparoscopic cholecystectomy and subtotal gastrectomy for gastric cancer. We produce calibrated annotations for the seven phases of cholecystectomy by analyzing the discrepancies of previously annotated labels and by discussing the criteria of surgical phases. For gastrectomy for gastric cancer has more complex twenty-one surgical phases, we generate consensus annotation by the revision process with five specialists. By training the CNN-based surgical phase recognition networks with revised annotations, we achieved improved generalization performance over models trained with original annotation under the same cross-validation settings. We showed that the expertise data annotation pipeline for deep neural networks should be more rigorous based on the type of problem to apply clinical field.
更多查看译文
关键词
surgical phase recognition,annotations,expert-generated
AI 理解论文
溯源树
样例
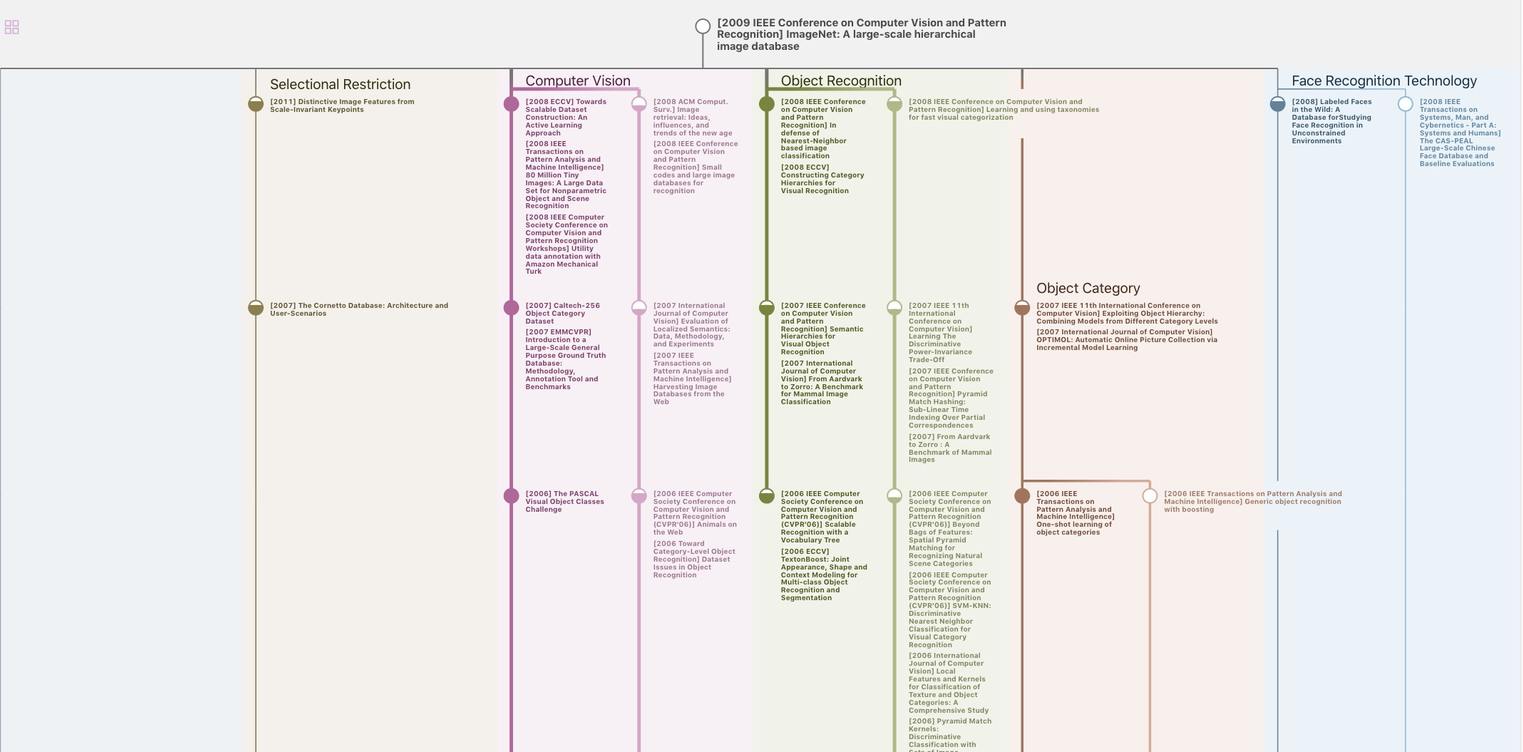
生成溯源树,研究论文发展脉络
Chat Paper
正在生成论文摘要