Critiquing-based Modeling of Subjective Preferences.
User Modeling, Adaptation, and Personalization (UMAP)(2022)
摘要
Crowdsourcing encompasses everything from large collaborative projects to microtasks performed in parallel and at scale. However, understanding subjective preferences can still be difficult: a majority of problems do not have validated questionnaires and pairwise comparisons do not scale, even with access to the crowd. Furthermore, in daily life we are used to expressing opinions as critiques (e.g. it was too cold, too spicy, too big), rather than describing precise preferences or choosing between (perhaps equally bad) discrete options. Unfortunately, it is difficult to analyze such qualitative feedback, especially when we want to make quantitative decisions. In this article, we present collective criticism, a crowdsourcing approach where users provide feedback to microtasks in the form of critiques, such as "it was too easy/too challenging". This qualitative feedback is used to perform quantitative analysis of users' preferences and opinions. Collective criticism has several advantages over other approaches: "too much/too little"-style critiques are easy for users to provide and it allows us to build predictive models for the optimal parameterization of the variables being critiqued. We present two case studies where we model: (i) aesthetic preferences in neural style transfer and (ii) hedonic experiences in the video game Tetris. These studies demonstrate the flexibility of our approach, and show that it produces robust results that are straightforward for experimenters to interpret and inline with users' stated preferences.
更多查看译文
关键词
critiquing, subjective preferences, user experience, randomized experiments
AI 理解论文
溯源树
样例
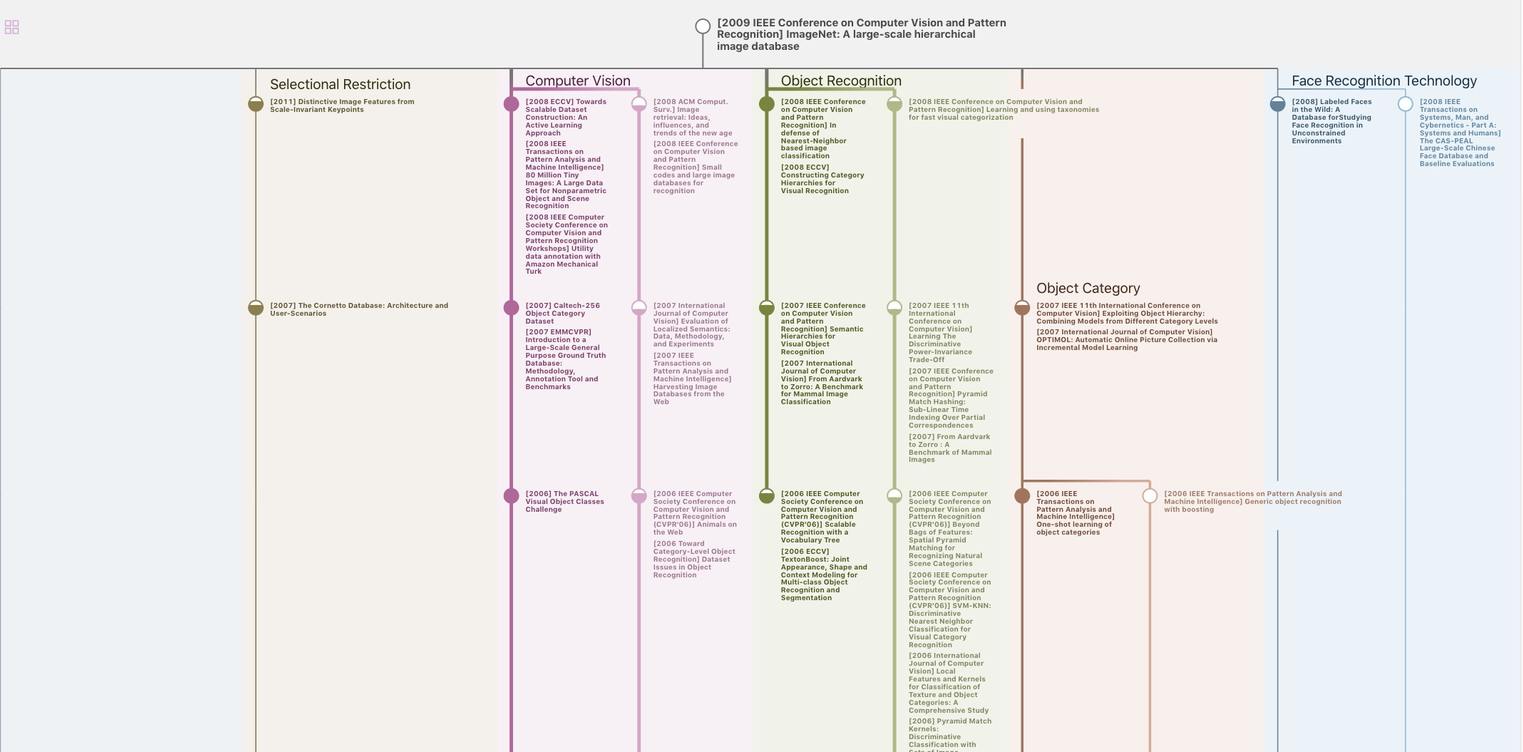
生成溯源树,研究论文发展脉络
Chat Paper
正在生成论文摘要