Global Convergence of Algorithms Under Constant Rank Conditions for Nonlinear Second-Order Cone Programming
Journal of Optimization Theory and Applications(2022)
摘要
In Andreani et al. (Weak notions of nondegeneracy in nonlinear semidefinite programming, 2020), the classical notion of nondegeneracy (or transversality) and Robinson’s constraint qualification have been revisited in the context of nonlinear semidefinite programming exploiting the structure of the problem, namely its eigendecomposition. This allows formulating the conditions equivalently in terms of (positive) linear independence of significantly smaller sets of vectors. In this paper, we extend these ideas to the context of nonlinear second-order cone programming. For instance, for an m -dimensional second-order cone, instead of stating nondegeneracy at the vertex as the linear independence of m derivative vectors, we do it in terms of several statements of linear independence of 2 derivative vectors. This allows embedding the structure of the second-order cone into the formulation of nondegeneracy and, by extension, Robinson’s constraint qualification as well. This point of view is shown to be crucial in defining significantly weaker constraint qualifications such as the constant rank constraint qualification and the constant positive linear dependence condition. Also, these conditions are shown to be sufficient for guaranteeing global convergence of several algorithms, while still implying metric subregularity and without requiring boundedness of the set of Lagrange multipliers.
更多查看译文
关键词
Second-order cone programming,Constraint qualifications,Algorithms,Global convergence,Constant rank.
AI 理解论文
溯源树
样例
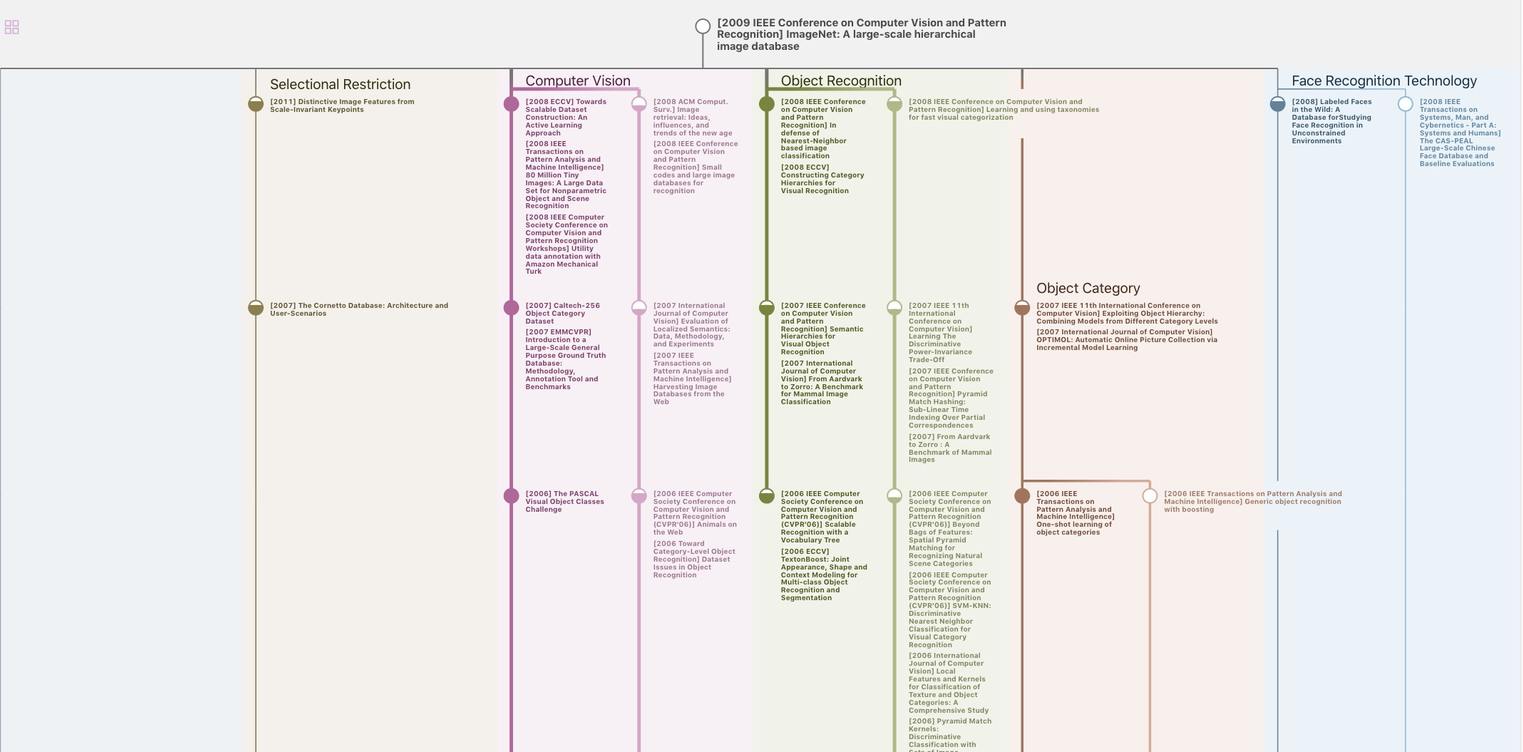
生成溯源树,研究论文发展脉络
Chat Paper
正在生成论文摘要