Learning to Estimate Without Bias
arxiv(2021)
摘要
We consider the use of deep learning for parameter estimation. We propose Bias Constrained Estimators (BCE) that add a squared bias term to the standard mean squared error (MSE) loss. The main motivation to BCE is learning to estimate deterministic unknown parameters with no Bayesian prior. Unlike standard learning based estimators that are optimal on average, we prove that BCEs converge to Minimum Variance Unbiased Estimators (MVUEs). We derive closed form solutions to linear BCEs. These provide a flexible bridge between linear regrssion and the least squares method. In non-linear settings, we demonstrate that BCEs perform similarly to MVUEs even when the latter are computationally intractable. A second motivation to BCE is in applications where multiple estimates of the same unknown are averaged for improved performance. Examples include distributed sensor networks and data augmentation in test-time. In such applications, unbiasedness is a necessary condition for asymptotic consistency.
更多查看译文
关键词
bias,learning,estimate
AI 理解论文
溯源树
样例
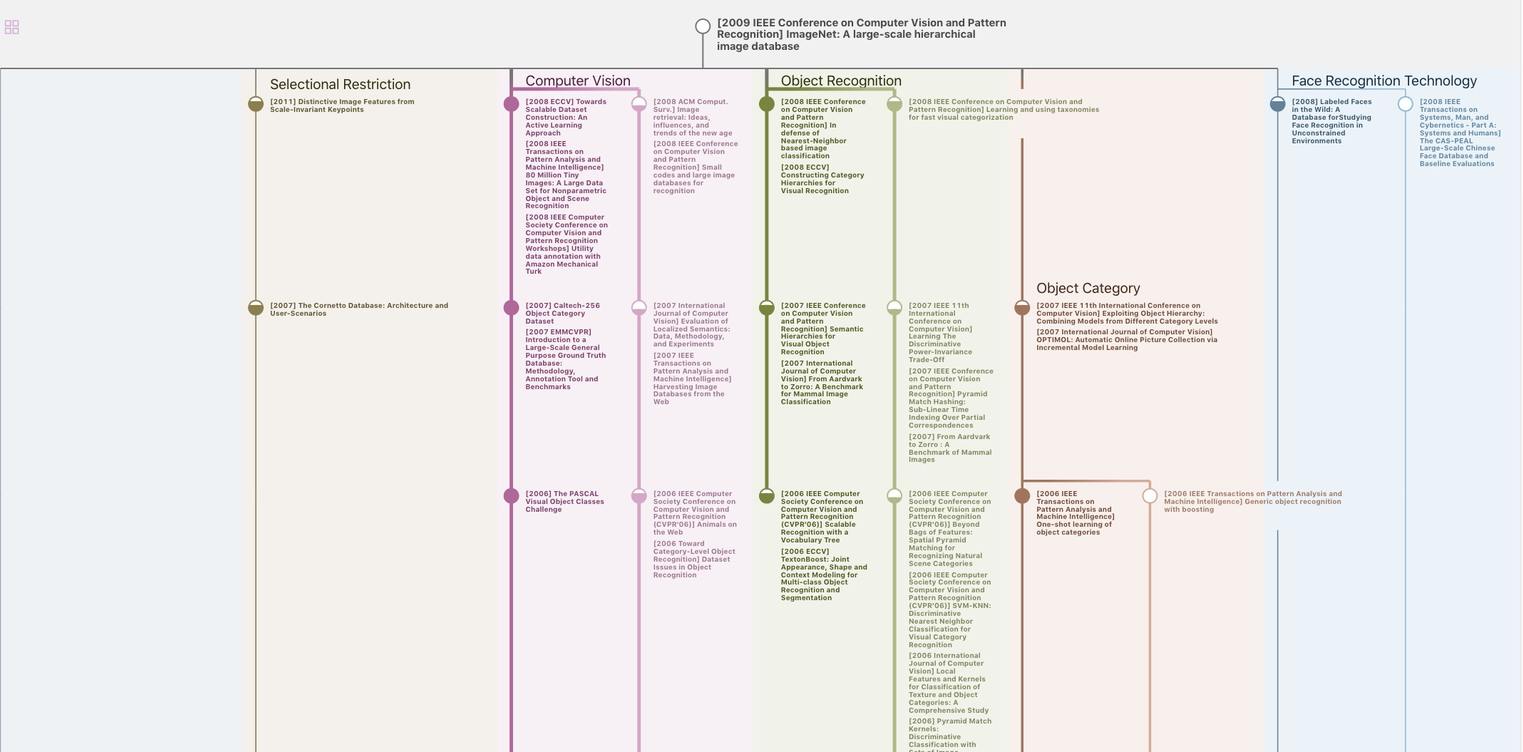
生成溯源树,研究论文发展脉络
Chat Paper
正在生成论文摘要