Online Estimation and Control with Optimal Pathlength Regret.
Conference on Learning for Dynamics & Control (L4DC)(2022)
摘要
A natural goal when designing online learning algorithms for non-stationary environments is to bound the regret of the algorithm in terms of the temporal variation of the input sequence. Intuitively, when the variation is small, it should be easier for the algorithm to achieve low regret, since past observations are predictive of future inputs. Such data-dependent "pathlength" regret bounds have recently been obtained for a wide variety of online learning problems, including OCO and bandits. We obtain the first pathlength regret bounds for online control and estimation (e.g. Kalman filtering) in linear dynamical systems. The key idea in our derivation is to reduce pathlength-optimal filtering and control to certain variational problems in robust estimation and control; these reductions may be of independent interest. Numerical simulations confirm that our pathlength-optimal algorithms outperform traditional $H_2$ and $H_{\infty}$ algorithms when the environment varies over time.
更多查看译文
关键词
optimal pathlength regret,online estimation,control
AI 理解论文
溯源树
样例
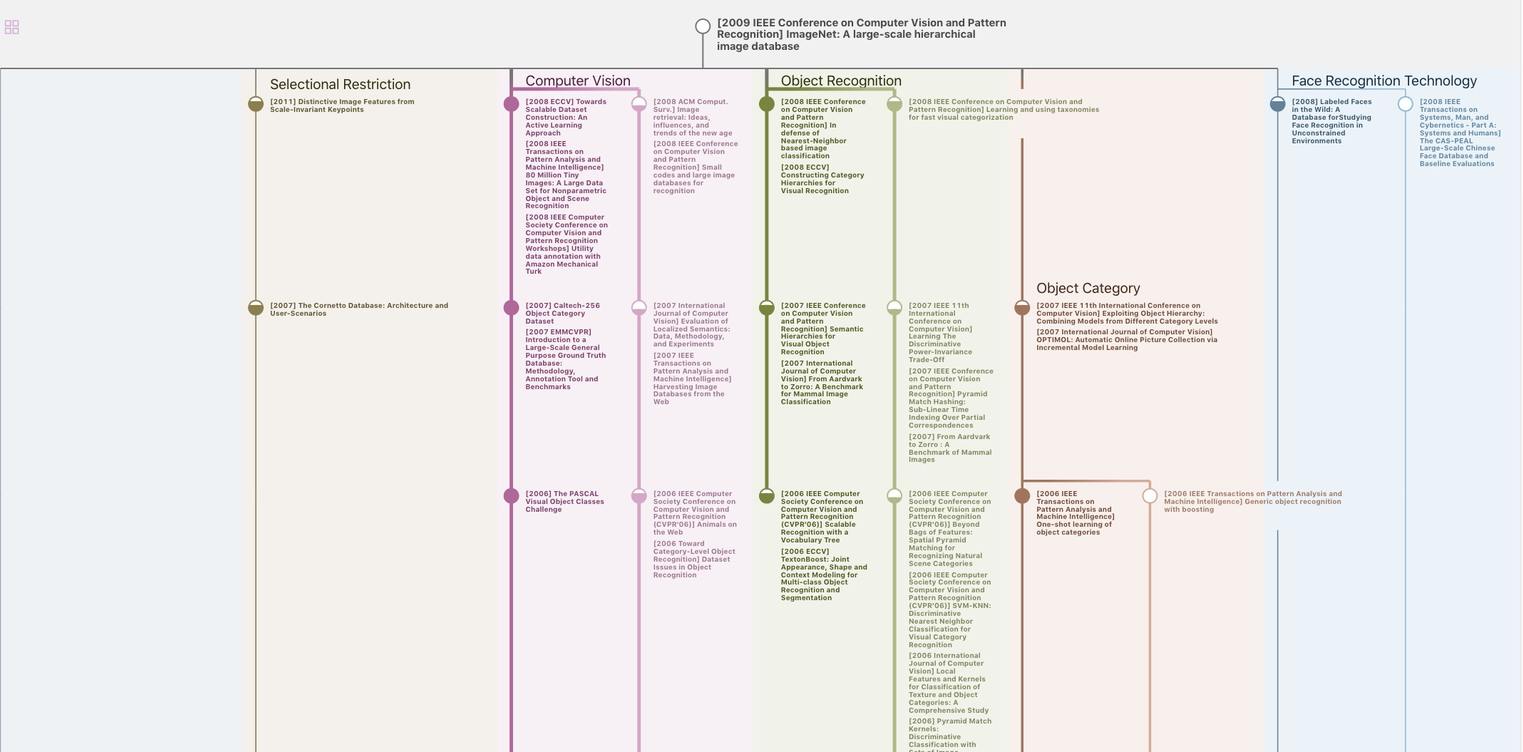
生成溯源树,研究论文发展脉络
Chat Paper
正在生成论文摘要