Operator shifting for model-based policy evaluation
COMMUNICATIONS IN MATHEMATICAL SCIENCES(2023)
摘要
In model-based reinforcement learning, the transition matrix and reward vector are often estimated from random samples subject to noise. Even if the estimated model is an unbiased estimate of the true underlying model, the value function computed from the estimated model is biased. We introduce an operator shifting method for reducing the error introduced by the estimated model. When the error is in the residual norm, we prove that the shifting factor is always positive and upper bounded by 1+ O(1/n), where n is the number of samples used in learning each row of the transition matrix. We also propose a practical numerical algorithm for implementing the operator shifting.
更多查看译文
关键词
Operator shifting,Model-based Reinforcement Learning,policy evaluation,noisy matrices
AI 理解论文
溯源树
样例
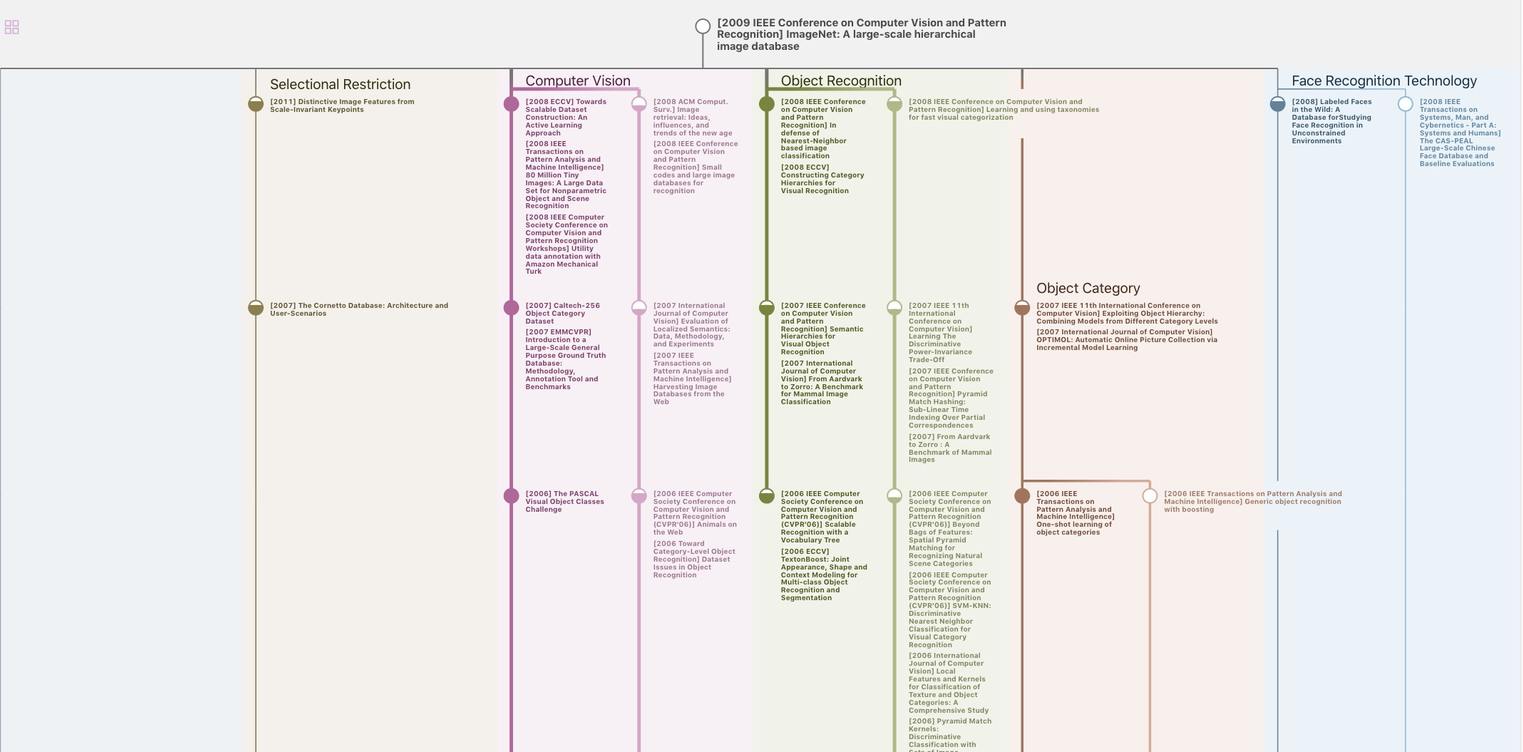
生成溯源树,研究论文发展脉络
Chat Paper
正在生成论文摘要