A novel federated learning approach based on the confidence of federated Kalman filters
International Journal of Machine Learning and Cybernetics(2021)
摘要
Federated learning (FL) is an emerging distributed artificial intelligence (AI) algorithm. It can train a global model with multiple participants and at the same time ensure the privacy of the participants’ data. Thus, FL provides a solution for the problems faced by data silos. Existing federated learning algorithms face two significant challenges when dealing with (1) non-independent and identically distributed (non-IID) data, and (2) data with noise or without preprocessing. To address these challenges, a novel federated learning approach based on the confidence of federated Kalman filters is proposed and is referred to as FedCK in this paper. Firstly, this paper proposes a deep Generative Adversarial Network with an advanced auxiliary classifier as a pre-training module. The Non-IID increases the discreteness of the parameters of local models, it is difficult for FL to aggregate an excellent global model. The pre-training module proposed in this paper can deeply mine hidden features and increase the correlation between local model parameters. Secondly, a federated learning framework based on Federated Kalman Filter (FKF) is proposed in this paper. Because the general federation average aggregation algorithm cannot identify the model parameters with noise. This paper uses the idea of FKF to propose a set of adaptive confidence to improve the fault tolerance of FL. Experiments carried out on the MNIST, CIFAR-10 and SVHN datasets demonstrate that FedCK has better robustness and accuracy than classical federated learning methods.
更多查看译文
关键词
Federated learning, Federated Kalman filter, Non-independent and identically distributed data, Confidence
AI 理解论文
溯源树
样例
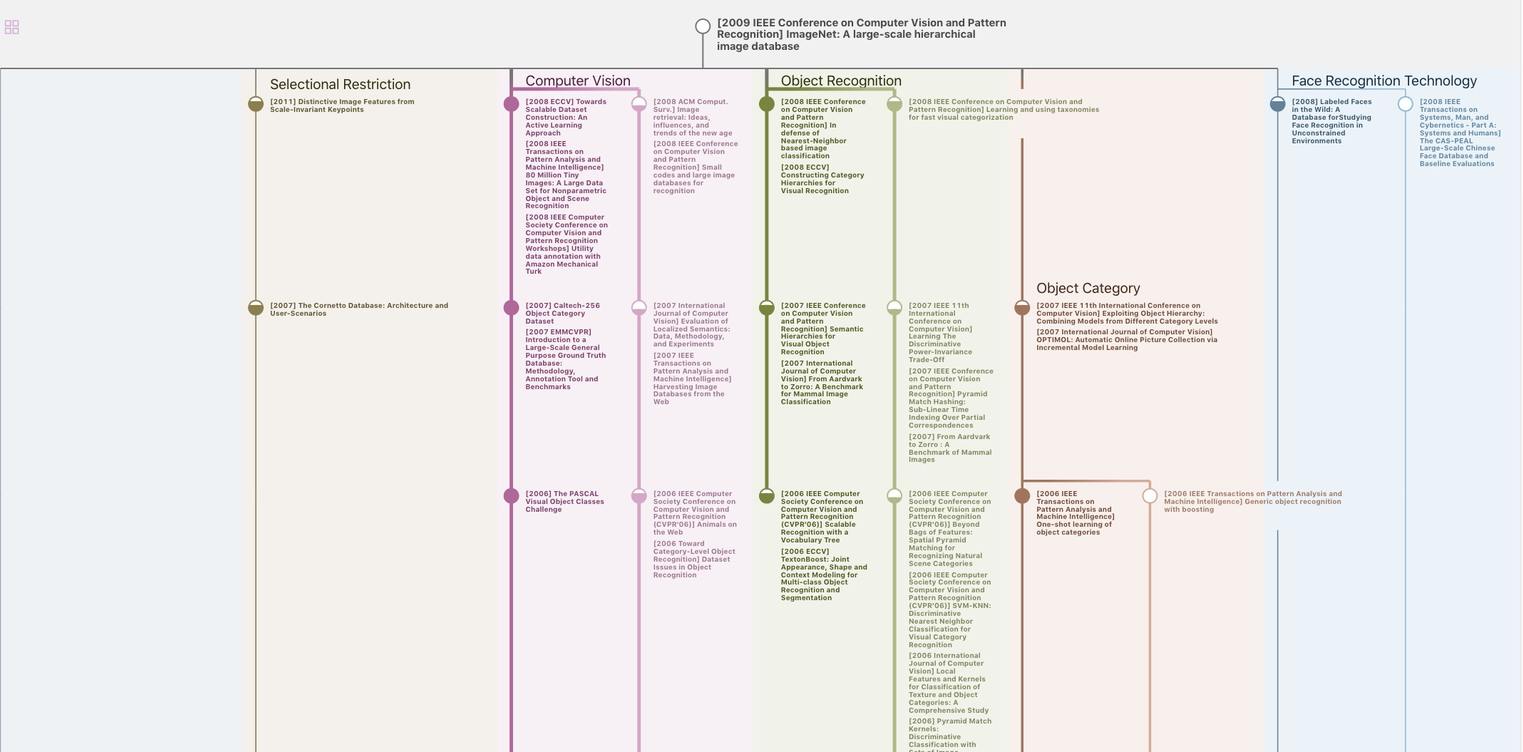
生成溯源树,研究论文发展脉络
Chat Paper
正在生成论文摘要